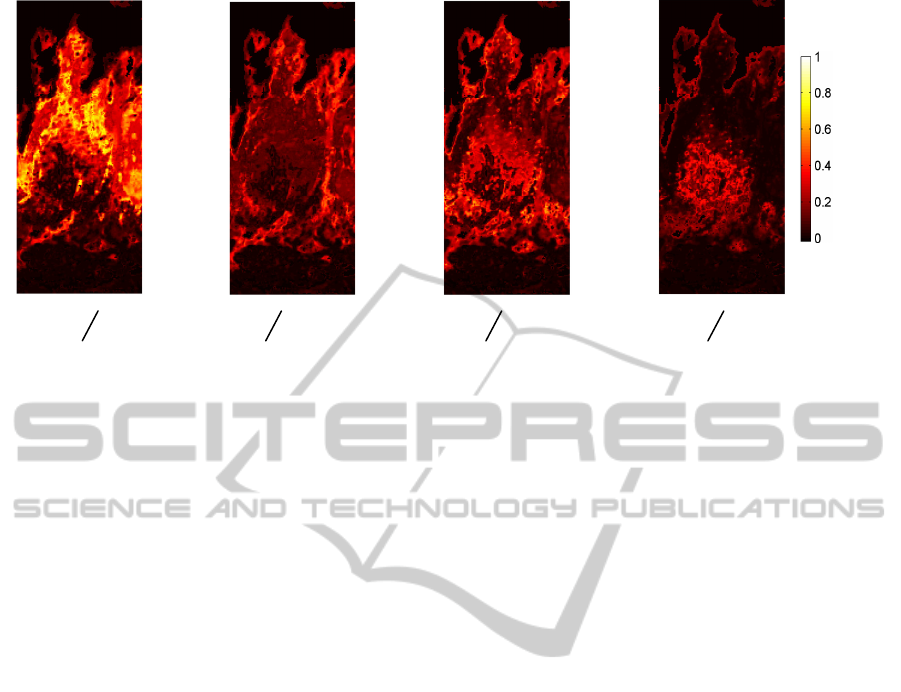
Interconnectionvalue
A B C D
Tumour
(cluster1)
Tumour
(cluster2)
Tumour
(cluster1)
Intratumoral
collagen
(cluster3)
Tumour
(cluster2)
Lymphocytes
(cluster4)
Tumour
(cluster2)
Intratumoral
collagen
(cluster3)
Figure 4: Illustration of interconnection measurements for an ulcerated superficial spreading melanoma with an invasive
nodular component (patient 2). 4 circumstances: A) between the tumour clusters, B) and C) between tumours and
intratumoral collagen clusters, D) between tumour and lymphocytes clusters.
tumour agressiveness, by revealing a risk of
malignant cell escape and consequently metastasis
formation. These first results need to be validated by
further analysis on a large number of samples,
before envisaging a potential application in clinics.
REFERENCES
Bezdek, J. C., 1981. Pattern recognition with fuzzy
objective function algorithms, Plenum Press. New
York.
Cancer Facts and Figures, 2013. American Cancer Society,
Atlanta.
Chin, L., Garraway, L. A., and Fisher, D. E., 2006.
Malignant melanoma: genetics and therapeutics in the
genomic era. Genes and development, vol. 20, pp.
2149-2182.
Gerger, A., Smolle, J., 2003. Diagnostic imaging of
melanocytic skin tumors. Journal of cutaneous
pathology, vol. 30, pp. 247-252.
Glusac, E. J., 2003. Under the microscope: doctors,
lawyers, and melanocytic neoplasms. Journal of
cutaneous pathology, vol. 30, pp. 287-293.
Hammody, Z., Argov, S., Sahu, R. K., Cagnano, E.,
Moreh, R., and Mordechai, S., 2008. Distinction of
malignant melanoma and epidermis using IR micro-
spectroscopy and statistical methods. Analyst, vol.
133, pp. 372-378.
Kong, R., Reddy, R. K., and Bhargava, R., 2010.
Characterization of tumor progression in engineered
tissue using infrared spectroscopic imaging. Analyst,
vol. 135, pp. 1569-1578.
Krishna, C. M., Sockalingum, G. D., Bhat, R. A., Venteo,
L., Kushtagi, P., Pluot, M., and Manfait, M., 2007.
FTIR and Raman microspectroscopy of normal,
benign, and malignant formalin-fixed ovarian tissues.
Analytical and Bioanalytical Chemistry, vol. 387, pp.
1649–1656.
Ly, E., Piot, O., Wolthuis, R., Durlach, A., Bernard, P.,
and Manfait, M., 2008. Combination of FTIR spectral
imaging and chemometrics for tumour detection from
paraffin-embedded biopsies. Analyst, vol. 133, pp.
197-205.
Ly, E., Piot, O., Durlach, A., Bernard, P., and Manfait, M.,
2009. Differential diagnosis of cutaneous carcinomas
by infrared spectral micro-imaging combined with
pattern recognition. Analyst, vol. 134, pp. 1208-1214.
Ly, E., Cardot-Leccia, N., Ortonne, J. P., Benchetrit, M.,
Michiels, J. F., Manfait, M., and Piot, O., 2010.
Histopathological characterization of primary
cutaneous melanoma using infrared microimaging: a
proof-of-concept study. British Journal of
Dermatology, vol. 162, pp. 1316-1323.
Marghoob, A. A., Scope, A., 2009. The complexity of
diagnosing melanoma. Journal of Investigative
Dermatology, vol. 129, pp. 11-13.
Miller, A. J., Mihm Jr, M. C., 2006. Melanoma. New
England Journal of Medicine, vol. 355, pp. 51-65.
Mordechai, S., Sahu, R. K., Hammody, Z., Mark, S.,
Kantarovich, K., Guterman, H., PODSHYVALOV,
A., GOLDSTEIN, J., and Argov, S., 2004. Possible
common biomarkers from FTIR microspectroscopy of
cervical cancer and melanoma. Journal of microscopy,
vol. 215, pp. 86-91.
Okcu, A., Hofmann-Wellenhof, R., Woltsche, I., Smolle,
J., and Kerl, H., 1996. Pathological findings
suggestive of interclonal stabilization in a case of
cutaneous melanoma. Clinical and experimental
metastasis, vol. 14, pp. 215-218.
Sebiskveradze, D., Vrabie, V., Gobinet, C., Durlach, A.,
Bernard, P., Ly, E., Manfait, M., Jeannesson, P., and
Piot, O., 2011. Automation of an algorithm based on
fuzzy clustering for analyzing tumoral heterogeneity in
BIOIMAGING2014-InternationalConferenceonBioimaging
64