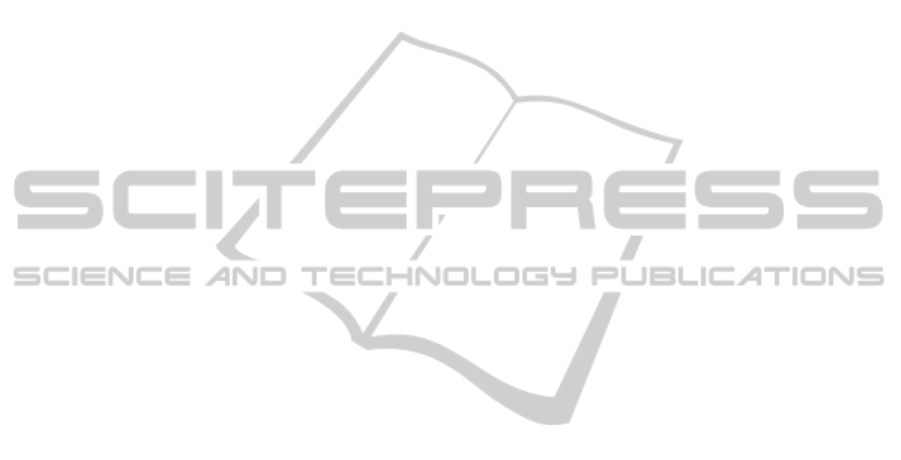
adopted to provide users with semantic elements,
and this is useful to understand strategic alliances.
Moreover, hypertrees have been successfully
exploited by several authors (Müller n.d.; Katifori et
al. 2007) to explore complex data-sets, to
comprehend the relationship existing in complex
phenomena and to find clusters, outliers and other
relevant patterns such as those outlined in our paper.
Finally, clouds of words have been used in a
wide diversity of applications, ranging from the
analytical to the emotional, and can be used for an
immediate visualization or most used words (static
word clouds) or for the illustration of the content
evolution in a stream of documents (Cui et al. 2010).
5 CONCLUSIONS AND FUTURE
WORKS
In this paper we present the main lines of a work
aimed at developing an interpretative framework to
understand how to use KPIs’ for monitoring and
benchmarking of Strategic Alliances. In particular,
we propose an ontology, two taxonomies and two
information visualization objects to help answering
question such as “which KPIs should I use in my
SA?”, “how can I understand if my SA or my firm
are achieving the predefined goals?”.
The proposal will be used to design and
implement an online database for strategic
partnerships governance and analysis and to test it
on the field. This online database can be useful to
SMEs that lack of the economical and managerial
resources required to enforce such a complex
performance measurement system.
Future works will include an improvement of the
content analysis, the linkage between goals and KPIs
and the analysis of SAs financial statements
.
REFERENCES
Agndal, H. & Nilsson, U., 2008. Supply chain decision-
making supported by an open books policy.
International Journal of Production Economics,
116(1), pp.154–167.
Bertolazzi, P., Krusich, C. & Missikoff, M., 2001. An
approach to the definition of a core enterprise
ontology: CEO. In OES-SEO 2001, International
Workshop on Open Enterprise Solutions: Systems,
Experiences, and Organizations. Citeseer, pp. 14–15.
Bititci, U.S. et al., 2004. Creating and managing value in
collaborative networks. International Journal of
Physical Distribution & Logistics Management,
34(3/4), pp.251–268.
Caglio, A. & Ditillo, A., 2008. A review and discussion of
management control in inter-firm relationships:
Achievements and future directions, Elsevier Ltd.
Caglio, A. & Ditillo, A., 2012a. Interdependence and
accounting information exchanges in inter-firm
relationships. Journal of Management & Governance,
16(1), pp.57–80.
Caglio, A. & Ditillo, A., 2012b. Opening the black box of
management accounting information exchanges in
buyer–supplier relationships. Management Accounting
Research, 23(2), pp.61–78.
Caputo, F., Livieri, B. & Venturelli, A., 2013. Intangibles
and Value Creation in Network Agreements: Analysis
of Italian firms. In Second “Management Control
Journal” Workshop: Controlling and Reporting for
Intangibles.
Comuzzi, M., Vanderfeesten, I. & Wang, T., 2013.
Optimized cross-organizational business process
monitoring: Design and enactment. Information
Sciences, 244, pp.107–118.
Comuzzi, M., Vonk, J. & Grefen, P., 2012. Measures and
mechanisms for process monitoring in evolving
business networks. Data & Knowledge Engineering,
71(1), pp.1–28.
Cui, W. et al., 2010. Context preserving dynamic word
cloud visualization. In Pacific Visualization Sym-
posium (PacificVis), 2010 IEEE. IEEE, pp. 121–128.
Das, T.K. & Teng, B.-S., 2000. A Resource-Based Theory
of Strategic Alliances. Journal of Management, 26(1),
pp.31–61.
Dietz, J., 2006. Enterprise Ontology: Theory and
Methodology, Springer.
Dyer, J.H., Kale, P. & Singh, H., 2001. Strategic Alliances
Work. MIT Sloan Management Review, pp.37–43.
Essa, S.A.G., Dekker, H.C. & Groot, T., 2014. The
influence of information and control on trust building
in buyer-supplier negotiations. In Management
Accounting Section (MAS) Research and Case
Conference.
Fox, M.S. et al., 1995. An Organization Ontology for
Enterprise Modelling. In Enabling Technologies:
Infrastructure for Collaborative Enterprises, 1995.,
Proceedings of the Fourth Workshop on. IEEE. pp. 1–25.
Fox, M.S., Chionglo, J.F. & Fadel, F.G., 1993. A
Common-Sense Model of the Enterprise. In
Proceedings of the 2nd Industrial Engineering
Research Conference.
Frank, U., 2012. Multi-perspective enterprise modeling:
foundational concepts, prospects and future research
challenges.
Software & Systems Modeling.
Gruninger, M. & Fox, M.S., 1994. The Design and
Evaluation of Ontologies for Enterprise Engineering.
In Workshop on Implemented Ontologies, European
Workshop on Artificial Intelligence, Amsterdam, The
Netherlands. pp. 1–14.
Hoogervorst, J.A.P., 2009. Enterprise Governance and
Enterprise Engineering, Springer.
Jamshidi, M., 2011. System of Systems Engineering: Inno-
vations for the Twenty-first century, John Wiley & Sons.
ICEIS2014-16thInternationalConferenceonEnterpriseInformationSystems
408