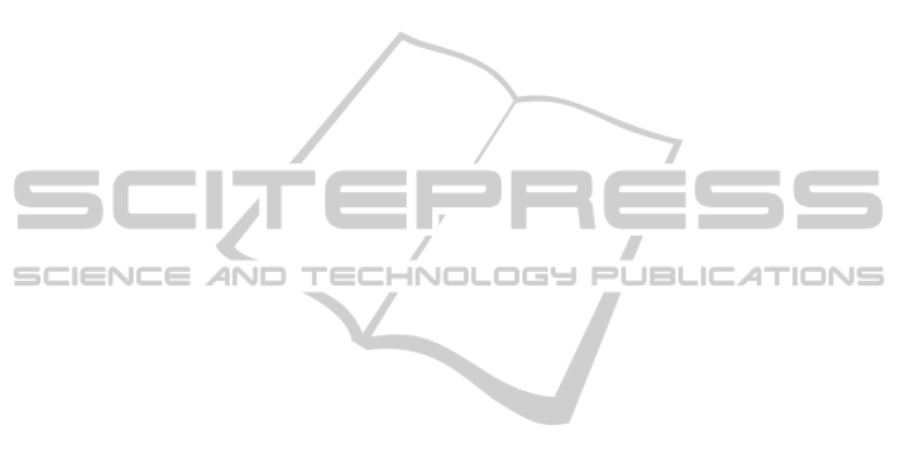
disease investigation, we think that using two
physiological signals (ECG and PCG) could be
efficient. Both ECG and PCG signals can thus be
used together for early stage detection of heart
disease into E-care platform.
In this work, an automated system for
preliminary heart defect detection is proposed. This
system is based on the concept of time-frequency
signal analysis.
REFERENCES
Ahlstrom, C., Lanne, T., Ask, P. & Johansson, A. 2008. A
Method For Accurate Localization Of The First Heart
Sound And Possible Applications. Physiol Meas, 29,
417-28.
Assous, S. & Boashash, B. 2012. Evaluation Of The
Modified S-Transform For Time-Frequency
Synchrony Analysis And Source Localisation. Eurasip
J Adv Signal Process, 2012, 49.
Auger, F. & Flandrin, P. 1995. Improving The Readability
Of Time-Frequency And Time-Scale Representations
By The Reassignment Method. Signal Processing,
Ieee Transactions On, 43, 1068-1089.
Auger, F., Flandrin, P., Yu-Ting, L., Mclaughlin, S.,
Meignen, S., Oberlin, T. & Hau-Tieng, W. 2013.
Time-Frequency Reassignment And
Synchrosqueezing: An Overview. Signal Processing
Magazine, Ieee, 30, 32-41.
Daubechies, I. 1990. The Wavelet Transform, Time-
Frequency Localization And Signal Analysis.
Information Theory, Ieee Transactions On, 36, 961-
1005.
Daubechies, I. & Maes, S. 1996. A Nonlinear Squeezing
Of The Continuous Wavelet Transform Based On
Auditory Nerve Models. Wavelets In Medicine And
Biology, Crc Press, 527–546.
Djurović, I., Sejdić, E. & Jiang, J. 2008. Frequency-Based
Window Width Optimization For -Transform. Aeu -
International Journal Of Electronics And
Communications, 62, 245-250.
Dugnol, B., Fernández, C., Galiano, G. & Velasco, J.
2007. Implementation Of A Diffusive Differential
Reassignment Method For Signal Enhancement: An
Application To Wolf Population Counting. Applied
Mathematics And Computation, 193, 374-384.
Erikson, B. 1997. Heart Sounds And Murmurs: A
Practical Guide.
European.Commission 2012. Ehealth Action Plan 2012-
2020– Innovative Healthcare For The 21st Century.
Brussels.
Fulop, S. A. & Fitz, K. 2006. Algorithms For Computing
The Time-Corrected Instantaneous Frequency
(Reassigned) Spectrogram, With Applications. J
Acoust Soc Am, 119, 360-71.
Jabloun, M., Ravier, P., Buttelli, O., Ledee, R., Harba, R.
& Nguyen, L. D. 2013. A Generating Model Of
Realistic Synthetic Heart Sounds For Performance
Assessment Of Phonocardiogram Processing
Algorithms. Biomedical Signal Processing And
Control, 8
, 455-465.
Kotte, O., Niethammer, M. & Jacobs, L. J. 2006. Lamb
Wave Characterization By Differential Reassignment
And Non-Linear Anisotropic Diffusion. Ndt & E
International, 39, 96-105.
Mansinha, L., Stockwell, R. G. & Lowe, R. P. 1997.
Pattern Analysis With Two-Dimensional Spectral
Localisation: Applications Of Two-Dimensional S
Transforms. Physica A: Statistical Mechanics And Its
Applications, 239, 286-295.
Moukadem, A. 2011. Segmentation Et Classification Des
Signaux Non-Stationnaires : Application Au
Traitement Des Sons Cardiaque Et A L'aide Au
Diagnostic. Université De Haute Alsace - Mulhouse.
Moukadem, A., Dieterlen, A., Hueber, N. & Brandt, C.
2013. A Robust Heart Sounds Segmentation Module
Based On S-Transform. Biomedical Signal Processing
And Control, 8, 273-281.
Phanphaisarn, W., Roeksabutr, A., Wardkein, P.,
Koseeyaporn, J. & Yupapin, P. 2011. Heart Detection
And Diagnosis Based On Ecg And Epcg
Relationships. Med Devices (Auckl), 4, 133-44.
Ping, Z. & Zhigang, W. A Computer Location Algorithm
For Ecg, Pcg And Cap. Engineering In Medicine And
Biology Society, 1998. Proceedings Of The 20th
Annual International Conference Of The Ieee, 29 Oct-
1 Nov 1998 1998. 220-222 Vol.1.
Sejdic, E., Djurovic, I. & Jin, J. S-Transform With
Frequency Dependent Kaiser Window. Acoustics,
Speech And Signal Processing, 2007. Icassp 2007.
Ieee International Conference On, 15-20 April 2007
2007. Iii-1165-Iii-1168.
Sejdic, E. & Jin, J. 2007. Selective Regional Correlation
For Pattern Recognition. Systems, Man And
Cybernetics, Part A: Systems And Humans, Ieee
Transactions On, 37, 82-93.
Stanković, L. 2001. A Measure Of Some Time–Frequency
Distributions Concentration. Signal Processing, 81,
621-631.
Stockwel, R. 2007. Why Use The S-Transform. Ed. By A
Wong (Fields Institute Communications, Providence),
Pseudo-Differential Operators: Pdes And Time-
Frequency Analysis, 279-309.
Stockwell, R. G., Mansinha, L. & Lowe, R. P. 1996.
Localization Of The Complex Spectrum: The S
Transform. Signal Processing, Ieee Transactions On,
44, 998-1001.
Weinstein, R. S., Lopez, A. M., Joseph, B. A., Erps, K. A.,
Holcomb, M., Barker, G. P. & Krupinski, E. A.
Telemedicine, Telehealth And Mhealth Applications
That Work: Opportunities And Barriers.
The American
Journal Of Medicine.
Zannad, F., Agrinier, N. & Alla, F. 2009. Heart Failure
Burden And Therapy. Europace, 11 Suppl 5, V1-9.
BIOSTEC2014-DoctoralConsortium
40