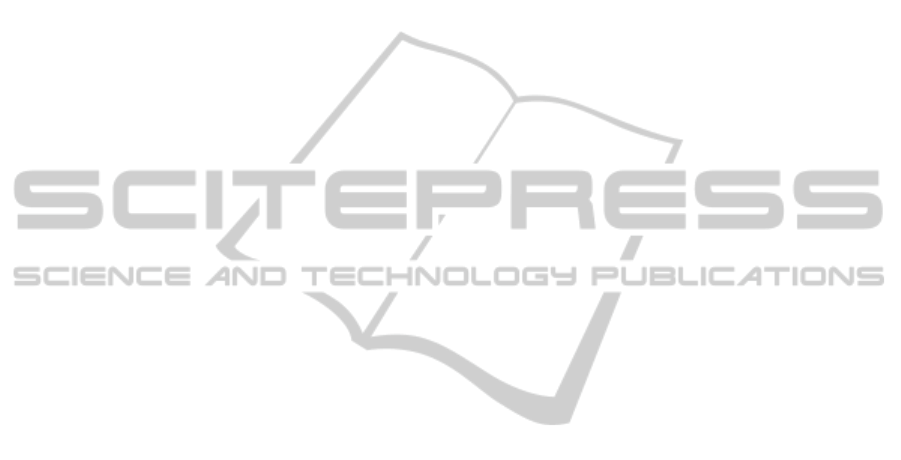
• Experimental Session 1 - a set of experiments per-
formed in the morning after usual sleep
• Experimental Session 2 - a set of experiments per-
formed in the afternoon after usual sleep
• Experimental Session 3 - a set of experiments per-
formed in the morning after sleep deprivation
• Experimental Session 4 - a set of experiments per-
formed in the afternoon after sleep deprivation
• GAX - the grand average computed by averaging
together the averaged waveforms of the partici-
pants of the experimental session X
• GAXY - the grand average computed by averag-
ing together the averaged waveforms of the par-
ticipants of the experimental sessions X and Y
Figure 1 shows the grand averages on the elec-
trode Fz for all experimental sessions and Figure 2
shows the grand averages on the electrode Fz for ses-
sions after usual sleep and sleep deprivation.
Table 2 presents the latency of the P3 component
on the electrode Fz using the technique of peak la-
tency. The resulting data were determined manually
using Brain Vision Analyzer software. Table 3 and
Table 4 show the latency of the P3 component af-
ter application of the fractional 50% peak latency and
fractional 50% area latency. These latencies were de-
termined by using a custom software application.
It is not possible to mutually compare the laten-
cies obtained by using different techniques. The im-
portance of latency is not in its absolute value but in
the difference between the values measured for the
experimental sessions described above.
The results did not demonstrate that the latency of
the P3 component was influenced by daytime. The
assumption that the latency of the P3 component in-
creases with sleep deprivation can be shown on the
results from Table 2 and Table 4. However, latencies
of individual participants do not confirm this hypothe-
sis when any technique described in this paper is used.
One possible reason for this result is that the number
of the target stimuli for each participant is too small to
get rid of signal noise. This noise is more eliminated
when grand averages are computed.
7 CONCLUSIONS
This paper shortly described the experiment that had
investigated attention of drivers by using the methods
of electroencephalography and event related poten-
tials. Experimental results showed that the P3 com-
ponent had been clearly identified during all exper-
imental sessions. Despite expectations, prolongation
of peak latency in time was not clearly observed when
the grand average measure of each participant was in-
vestigated. On the other hand, this prolongation was
observable when the techniques of peak latency and
fractional 50% area latency were applied to compute
the grand average for each experimental session. The
results are currently not statistically evaluated to pro-
vide more detailed information.
In the future, it would be probably appropriate to
increase the number of target stimuli (i.e. to prolong
the drive) to get more evident results from individual
participants.
ACKNOWLEDGEMENTS
The work was supported by the UWB grant SGS-
2013-039 Methods and Applications of Bio- and
Medical Informatics and by the European Regional
Development Fund (ERDF), Project ”NTIS - New
Technologies for Information Society”, European
Centre of Excellence, CZ.1.05/1.1.00/02.0090.
REFERENCES
Brain Products (2013a). Brain vision analyzer.
Brain Products (2013b). Brain vision recorder.
Bueno, M., Fabrigoule, C., Deleurence, P., Ndiaye, D., and
Fort, A. (2012). An electrophysiological study of the
impact of a forward collision warning system in a sim-
ulator driving task. Brain Research, 1470:69–79. cited
By (since 1996)1.
EEG/ERP Portal (2008-2013). EEG/ERP Portal.
Filtness, A., Reyner, L., and Horne, J. (2012). Driver
sleepiness-comparisons between young and older men
during a monotonous afternoon simulated drive. Bio-
logical Psychology, 89(3):580–583.
Kiesel, A., Miller, J., Jolicour, P., and Brisson, B. (2008).
Measurement of erp latency differences: A compari-
son of single-participant and jackknife-based scoring
methods. Psychophysiology, 45(2):250–274.
Li, W., He, Q.-C., Fan, X.-M., and Fei, Z.-M. (2012). Eval-
uation of driver fatigue on two channels of eeg data.
Neuroscience Letters, 506(2):235–239.
Luck, S., Woodman, G., and Vogel, E. (2000). Event-related
potential studies of attention. Trends in Cognitive Sci-
ences, 4(11).
Luck, S. J. (2005). An Introduction to the Event-Related Po-
tential Technique (Cognitive Neuroscience). A Brad-
ford Book, 1 edition.
Moucek, R. and Rericha, J. (2012). Driver’s attention dur-
ing monotonous driving. 2012 5th International Con-
ference on Biomedical Engineering and Informatics,
BMEI 2012, pages 486–490.
AttentionofDriverduringSimulatedDrive
549