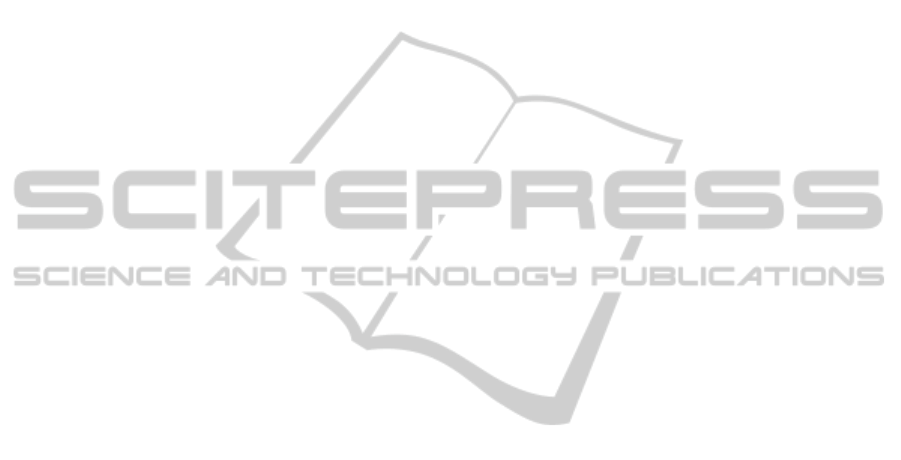
metabolic risk, lifestyle-habits risks).
To reach methodological and technological
objectives, clinical scores of cardiometabolic risk
have a twofold role: they provide a path to develop
the new indices for cardiometabolic risk and offer a
well-established basis to validate the system.
It is worth noting that several signs observed by
the Wize Mirror are related to the parameters used in
the risk scores shown in Figure 2.
During the validation part of the project we plan
to evaluate the association of metabolic parameters
with the measured clinical parameters in order to
evaluate the new cardiometabolic risk scores (i.e.
WBI components) made available by the Wize
Mirror platform.
5 CONCLUSIONS
In recent years, self-monitoring and self-training
approaches to personalized strategies for the
cardiometabolic risk prevention have experienced
growing interest from both the scientific community
and health care systems.
In this context, medical semeiotics offers a sound
methodological frame to build new computational
tools also exploiting innovative multi-sensing
devices. The rich variety of signs detectable in an
individual’s face is particularly attractive to
implement effective methods for self-assessment of
individuals’ health status. The integration of
computational descriptors of well-established face
signs (e.g. expressive traits, morphometric and
colorimetric features) with new measurements of
physiological quantities (e.g. skin cholesterol, AGE
concentration, heart and respiratory rates, analysis of
exhaled gases) is an important step towards digital
semeiotics. In view of that, the existing charts of
cardio metabolic risk offer significant clues and
provide meaningful indications to researchers and
system developers. At the same time, they remain
essential tools to validate self-monitoring activity.
ACKNOWLEDGEMENTS
This work was partly supported by the EU FP7
Project SEMEOTICONS - SEMEiotic Oriented
Technology for Individual’s CardiOmetabolic risk
self-assessmeNt and Self-monitoring (Grant
agreement no: 611516).
REFERENCES
Alberti, K. G., R. H. Eckel, et al., 2009. "Harmonizing the
metabolic syndrome: a joint interim statement of the
International Diabetes Federation Task Force on
Epidemiology and Prevention; National Heart, Lung,
and Blood Institute; American Heart Association;
World Heart Federation; International Atherosclerosis
Society; and International Association for the Study of
Obesity." Circulation 120(16): 1640-1645.
Alberti, K. G., P. Zimmet, et al., 2006. "Metabolic
syndrome--a new world-wide definition. A Consensus
Statement from the International Diabetes Federation."
Diabet Med 23(5): 469-480.
Amato, M. C., C. Giordano, et al., 2010. "Visceral
Adiposity Index: a reliable indicator of visceral fat
function associated with cardiometabolic risk."
Diabetes Care 33(4): 920-922.
Andreozzi, F., A. Gastaldelli, et al., 2013. "Increased
carotid intima-media thickness in the physiologic
range is associated with impaired postprandial glucose
metabolism, insulin resistance and beta cell
dysfunction." Atherosclerosis 229(2): 277-281.
Anstee, Q. M., G. Targher, et al., 2013. "Progression of
NAFLD to diabetes mellitus, cardiovascular disease or
cirrhosis." Nat Rev Gastroenterol Hepatol 10(6): 330-
344.
Arsenault, B. J., I. Lemieux, et al., 2010. "The
hypertriglyceridemic-waist phenotype and the risk of
coronary artery disease: results from the EPIC-Norfolk
prospective population study." CMAJ 182(13): 1427-
1432.
Balkau, B., C. Lange, et al., 2010. "Nine-year incident
diabetes is predicted by fatty liver indices: the French
D.E.S.I.R. study." BMC Gastroenterol 10: 56.
Bedogni, G., S. Bellentani, et al., 2006. "The Fatty Liver
Index: a simple and accurate predictor of hepatic
steatosis in the general population." BMC
Gastroenterol 6: 33.
Bedogni, G., H. S. Kahn, et al., 2010. "A simple index of
lipid overaccumulation is a good marker of liver
steatosis." BMC Gastroenterol 10: 98.
Blackburn, P., I. Lemieux, et al., 2009. "The
hypertriglyceridemic waist phenotype versus the
National Cholesterol Education Program-Adult
Treatment Panel III and International Diabetes
Federation clinical criteria to identify high-risk men
with an altered cardiometabolic risk profile."
Metabolism 58(8): 1123-1130.
Calori, G., G. Lattuada, et al., 2011. "Fatty liver index and
mortality: the Cremona study in the 15th year of
follow-up." Hepatology 54(1): 145-152.
Carey, V. J., E. E. Walters, et al., 1997. "Body fat
distribution and risk of non-insulin-dependent diabetes
mellitus in women. The Nurses' Health Study." Am J
Epidemiol 145(7): 614-619.
de Graaf, F. R., J. D. Schuijf, et al., 2010. "Usefulness of
hypertriglyceridemic waist phenotype in type 2
diabetes mellitus to predict the presence of coronary
artery disease as assessed by computed tomographic
HEALTHINF2014-InternationalConferenceonHealthInformatics
604