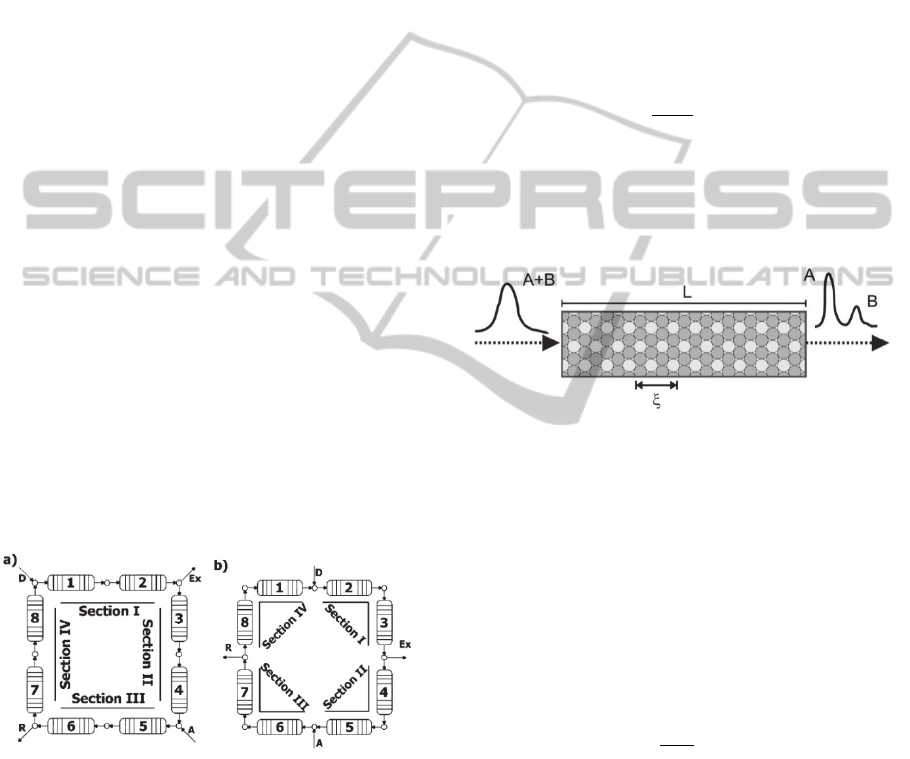
and continuous (dispersion) models can model
chromatographic columns. Currently, the models
used by the researchers are robust and efficient, but
they require a numerical treatment of partial
differential equations, which carries a high
computational cost.
In order to get a new math model to predict the
profiles of separating compounds of a mixture
submitted to a chromatographic separation by SMB
adsorption process, the new approach called Front
Velocity is proposed.
This approach does not require equilibrium
experiments, does not need application of adsorption
isotherms for characterizing the components
involved in the process, and is compost for ordinary
differential equations.
To validate the proposed model, it was applied in
the separation of the enantiomers of ketamine
anaesthetic (Santos, 2004; Santos et al., 2004) and
the results were compared with those obtained by
conventional models.
2 THEORY
The SMB process used by Santos in the separation
of enantiomers of racemic Ketamine consists of
eight chromatographic columns connected in series,
divided two by two per section. Each column has
0.77 cm diameter and 20 cm in length as shown in
Figure 1. The more retained enantiomer (R) is
collected in the extract (Ex), while the least adsorbed
enantiomer (S) is collected in the raffinate (R).
Figure 1: SMB process with two chromatography columns
per section.
In the stationary and mobile phases were used,
respectively, MCTA (microcrystalline cellulose
acetate) and ethanol. (Santos et al., 2004) also used a
HPLC (High Performance Liquid Chromatography)
column, where was injected ketamine samples for
calibration and determination of purity. The HPLC
column used has 0.46 cm in diameter and 20 cm in
length.
To represent the mass transfer, which occurs
inside one chromatography column, during a
separation process, the Front Velocity new approach
was developed. This establishes that convection is
the dominant phase in the solute transport along the
chromatographic column. The Front Velocity is a
discrete model (mixed cells), where the flow rate
determines the liquid phase advances along the
column. The rate at which the liquid phase
percolates the porous column (v), is the ratio
between volumetric flow rate of the mobile phase
through the porous medium is described by Equation
1,
.
(1)
where, , and
represent the volumetric flow
rate, porosity and the total area of the porous
column, respectively (these data are obtained
experimentally).
Figure 2: Chromatographic column of length L, and
volume control length ξ.
To perform the calculation of the mass transfer,
the chromatographic column was divided into
control volumes (mixed cells) of length ξ, that
moves along the column with the same speed as the
eluent, as can be seen in Figure 2.
The necessary time to move the liquid phase
along each control volume is obtained from
Equation 2, where is the total volume of the
column and is the number of mixed cells.
∆
.
.
(2)
To perform the calculation of the mass transfer in
the chromatography process SMB, the equations
with lumped mass transfer parameter kinetics (Eqs.
3, 4) assumes that equilibrium is achieved
everywhere at all times, so that the effects of axial
dispersion and the mass transfer resistance were
disregarded. Kinetic equations of mass transfer has
been successfully employed in chromatographic
processes (Câmara, 2014; Bihain et al., 2012).
SIMULTECH2014-4thInternationalConferenceonSimulationandModelingMethodologies,Technologiesand
Applications
6