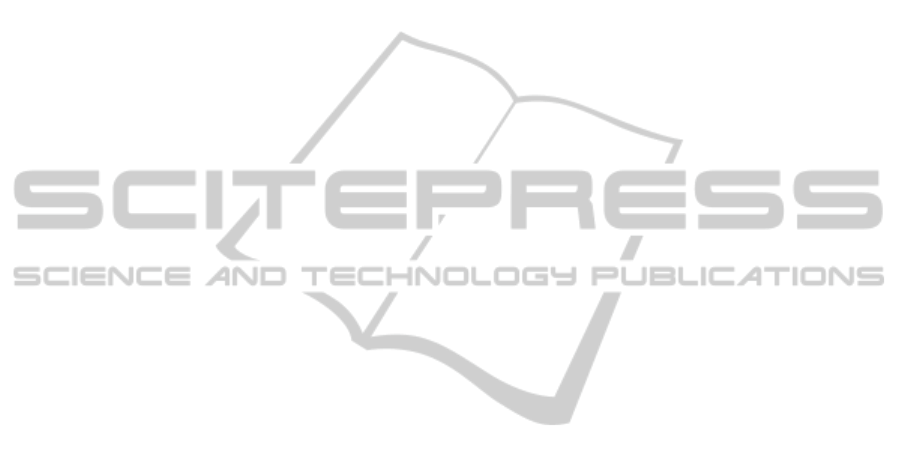
tail restricting data and behavoiurs to provide a more
tractable simulation with a more managable parame-
ter space, it is envisaged that scenarios simulating a
range of bank behaviours under idealised regulatory
regimes (e.g. Basel III) would be a logical next step,
hence the inclusion of LCR.
7 CONCLUSIONS
Ideally, an agent-based model may encapsulates ex-
pert knowledge, actor behaviours and system struc-
ture in a manner that eludes other techniques. The
model described here does not share the clean and
often elegant characteristics of a classical, analytical
model, but then the real world does not share these
features either. Neither does it posses the rich de-
tail of institutions’ financial positions, covering hun-
dreds of pages of their annual reports, one of the ex-
perts commented that there is no simple represen-
tation of a £1.4tn balance sheet. Our model does
capture behaviours, structure and expert knowledge
and includes some of the data richness of the real
world. The most important outcome of the initial sim-
ulations, like the creditworthiness example described
here, is that they demonstrate that the model is an in-
teracting set of institutions rather than merely single
entities or an aggregation of many. For example, the
effect of diminished creditworthiness on a bank was a
combination of the behavioural responses of the other
agents and its own response to those actions, all oc-
curring within the context of a realistic structure with
real data. These features are particularly suitable for
analysing crisis scenarios or testing the impact of reg-
ulation where behavioural responses can dictate out-
comes.
ACKNOWLEDGEMENTS
We wish to acknowledge the invaluable input and sup-
port we received from Peter Lightfoot of the Royal
Bank of Scotland and Simon Bailey of the CGI
Group.
REFERENCES
Acharya, V. V. and Merrouche, O. (2012). Precautionary
hoarding of liquidity and interbank markets: Evidence
from the subprime crisis. Review of Finance.
Affinito, M. (2012). Do interbank customer relation-
ships exist? and how did they function in the crisis?
learning from italy. Journal of Banking & Finance,
36(12):3163 – 3184. Systemic risk, Basel III, global
financial stability and regulation.
Allen, F. and Gale, D. (2000). Financial contagion. The
Journal of Political Economy, 108(1):1–33.
Bagehot, W. (1873). Lombard Street: A Description of the
Money Market. London:HS King, London.
Biancotti, C., D’Aurizio, L., Ilardi, G., and Terna, P. (2009).
Modelling an rtgs system with slapp. Technical report.
BIS (2013). Basel iiii the liquidity coverage ratio and liq-
uidity risk monitoring tools. Technical report, Bank
of International Settlements.
BIS (2014). Basel iii: The net stable funding ratio. Techni-
cal report, Bank of International Settlements.
Burrows, O., Learmouth, D., McKeown, J., and Williams,
R. (2012). Ramsi: a top-down stress-testing model
developed at the banks of england’s. Technical report,
Bank of England.
EC (2012). Non-bank financial institutions: Assessment of
their impact on the stability of the financial system.
Technical report, European Commission Directorate-
General for Economic and Financial Affairs.
Economist (2013). Bear in the china shop. Economist, 408.
Farmer, J. D. and Foley, D. (2009). The economy needs
agent-based modelling. Nature, 460:685–686.
Galbiati, M. and Soramki, K. (2011). An agent-based model
of payment systems. Journal of Economic Dynamics
and Control, 35(6):859–875.
Gallegati, M., Giulioni, G., and Kichiji, N. (2003). Com-
plex dynamics and financial fragility in an agent-
based model. Advances in Complex Systems (ACS),
6(03):267–282.
Geanakoplos, J., Axtell, R., Farmer, D., Howitt, P., Conlee,
B., Goldstein, J., Hendrey, M., and Palmer, Nathan
amd Yang, C.-Y. (2012). Getting at systemic risk via
an agent-based model of the housing market. Techni-
cal report, Cowles Foundation.
Giannone, D., Lanza, M., Pill, H., and Reichlin, L. (2012).
The ecb and the interbank market. Technical report,
European Central Bank.
Honohan, P. and Klingebiel, D. (2003). The fiscal cost im-
plications of an accommodating approach to banking
crises. Journal of Banking & Finance, 27:1539–1560.
Kindleberger, C. (1996). Maniacs, Panics and Crashes.
Macmillan.
Matsuoka, T. (2012). Imperfect interbank markets and the
lender of last resort. Journal of Economic Dynamics
and Control, 36(11):1673 – 1687.
Railsback, S. F., Lytinen, S. L., and Jackson, S. K. (2006).
Agent-based simulation platform: Review and devel-
opment recommendations. In (Railsback et al., 2006),
pages 609–623.
Thornton, M. K. and Thornton, R. L. (1990). The financial
crisis of a.d. 33: A keynsian depression? The Journal
of Economic History, 50:655–662.
van den End, J. W. and Tabbae, M. (2012). When liq-
uidity risk becomes a systemic issue: Empirical evi-
dence of bank behaviour. Journal of Financial Stabil-
ity, 8(2):107 – 120.
SIMULTECH2014-4thInternationalConferenceonSimulationandModelingMethodologies,Technologiesand
Applications
236