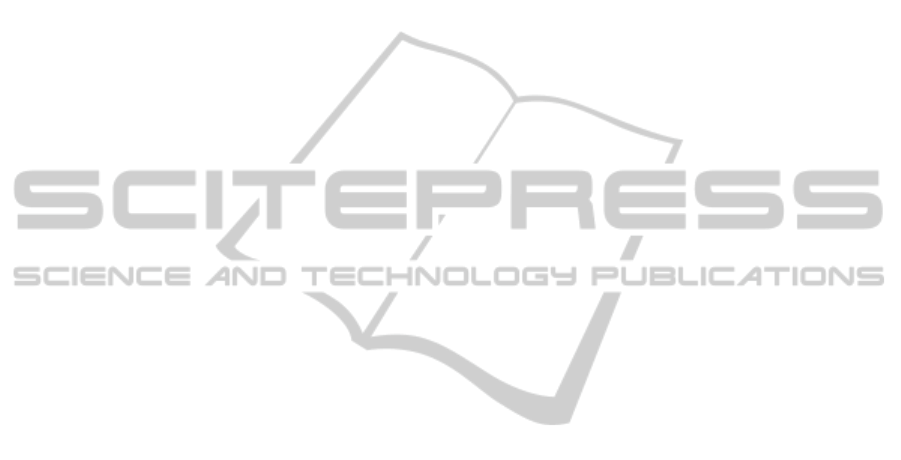
REFERENCES
Abhau, J., Belhachmi, Z., and Scherzer, O. (2009). On
a decomposition model for optical flow. In Energy
Minimization Methods in Computer Vision and Pat-
tern Recognition, pages 126–139. Springer.
Abrams, R. A. and Christ, S. E. (2003). Motion onset
captures attention. Psychological Science, 14(5):427–
432.
Bar, M. (2007). The proactive brain: using analogies and
associations to generate predictions. Trends in cogni-
tive sciences, 11(7):280–289.
Becker, S. I. and Horstmann, G. (2011). Novelty and
saliency in attentional capture by unannounced mo-
tion singletons. Acta psychologica, 136(3):290–299.
B¨ohme, M., Dorr, M., Krause, C., Martinetz, T., and Barth,
E. (2006). Eye movement predictions on natural
videos. Neurocomputing, 69(16–18):1996–2004.
Brooks, D. I., Rasmussen, I. P., and Hollingworth, A.
(2010). The nesting of search contexts within natu-
ral scenes: Evidence from contextual cuing. Journal
of Experimental Psychology: Human Perception and
Performance, 36(6):1406.
Burnham, B. R. (2007). Displaywide visual features asso-
ciated with a search displays appearance can mediate
attentional capture. Psychonomic Bulletin & Review,
14(3):392–422.
Carmi, R. and Itti, L. (2006). Visual causes versus correlates
of attentional selection in dynamic scenes. Vision Re-
search, 46(26):4333 – 4345.
Cutting, J. E., Brunick, K. L., and Candan, A. (2012). Per-
ceiving event dynamics and parsing hollywood films.
Journal of experimental psychology: human percep-
tion and performance, 38(6):1476.
Deubel, H. and Schneider, W. X. (1996). Saccade tar-
get selection and object recognition: Evidence for
a common attentional mechanism. Vision Research,
36(12):1827 – 1837.
Duncan, J. and Humphreys, G. W. (1989). Visual search and
stimulus similarity. Psychological review, 96(3):433.
Foulsham, T., Cheng, J. T., Tracy, J. L., Henrich, J., and
Kingstone, A. (2010). Gaze allocation in a dynamic
situation: Effects of social status and speaking. Cog-
nition, 117(3):319–331.
Frintrop, S., Rome, E., and Christensen, H. I. (2010). Com-
putational visual attention systems and their cognitive
foundations: A survey. ACM Transactions on Applied
Perception (TAP), 7(1):6.
Hasson, U., Nir, Y., Levy, I., Fuhrmann, G., and Malach,
R. (2004). Intersubject synchronization of cortical ac-
tivity during natural vision. science, 303(5664):1634–
1640.
Itti, L. and Baldi, P. (2009). Bayesian surprise attracts hu-
man attention. Vision research, 49(10):1295–1306.
Itti, L. and Koch, C. (2001). Computational modelling
of visual attention. Nature reviews neuroscience,
2(3):194–203.
Itti, L., Koch, C., Niebur, E., et al. (1998). A model of
saliency-based visual attention for rapid scene analy-
sis. IEEE Transactions on pattern analysis and ma-
chine intelligence, 20(11):1254–1259.
Luck, S. J. and Vogel, E. K. (1997). The capacity of vi-
sual working memory for features and conjunctions.
Nature, 390(6657):279–281.
Maljkovic, V. and Nakayama, K. (1994). Priming of
pop-out: I. role of features. Memory & cognition,
22(6):657–672.
Maxcey-Richard, A. M. and Hollingworth, A. (2013). The
strategic retention of task-relevant objects in visual
working memory. Journal of Experimental Psychol-
ogy: Learning, Memory, and Cognition, 39(3):760.
Mital, P., Smith, T. J., Luke, S., and Henderson, J. (2013).
Do low-level visual features have a causal influence
on gaze during dynamic scene viewing? Journal of
Vision, 13(9):144–144.
Najemnik, J. and Geisler, W. S. (2005). Optimal eye
movement strategies in visual search. Nature,
434(7031):387–391.
Patrone, A. R. (2014). Optical flow decomposition with
time regularization. In Conference on IMAGING SCI-
ENCE.
Posner, M. I. (1980). Orienting of attention. Quarterly Jour-
nal of Experimental Psychology, 32:3–25–.
Royden, C. S., Wolfe, J. M., and Klempen, N. (2001).
Visual search asymmetries in motion and optic flow
fields. Perception & Psychophysics, 63(3):436–444.
Rushton, S. K., Bradshaw, M. F., and Warren, P. A. (2007).
The pop out of scene-relative object movement against
retinal motion due to self-movement. Cognition,
105(1):237–245.
Smith, T. J., Levin, D., and Cutting, J. E. (2012). A window
on reality perceiving edited moving images. Current
Directions in Psychological Science, 21(2):107–113.
Theeuwes, J. (2010). Top–down and bottom–up control of
visual selection. Acta psychologica, 135(2):77–99.
Torralba, A., Oliva, A., Castelhano, M. S., and Hender-
son, J. M. (2006). Contextual guidance of eye move-
ments and attention in real-world scenes: the role of
global features in object search. Psychological review,
113(4):766.
Treisman, A. M. and Gelade, G. (1980). A feature-
integration theory of attention. Cognitive psychology,
12(1):97–136.
Valuch, C., Ansorge, U., Buchinger, S., Patrone, A. R., and
Scherzer, O. (2014). The effect of cinematic cuts on
human attention. In TVX, pages 119–122.
Valuch, C., Becker, S. I., and Ansorge, U. (2013). Priming
of fixations during recognition of natural scenes. J Vis,
13(3).
Wang, H. X., Freeman, J., Merriam, E. P., Hasson, U., and
Heeger, D. J. (2012). Temporal eye movement strate-
gies during naturalistic viewing. Journal of vision,
12(1):16.
Wolfe, J. M. (1994). Guided search 2.0 a revised model
of visual search. Psychonomic bulletin & review,
1(2):202–238.
Zelinsky, G. J. (2008). A theory of eye movements during
target acquisition. Psychological review, 115(4):787.
VisualAttentioninEditedDynamicalImages
205