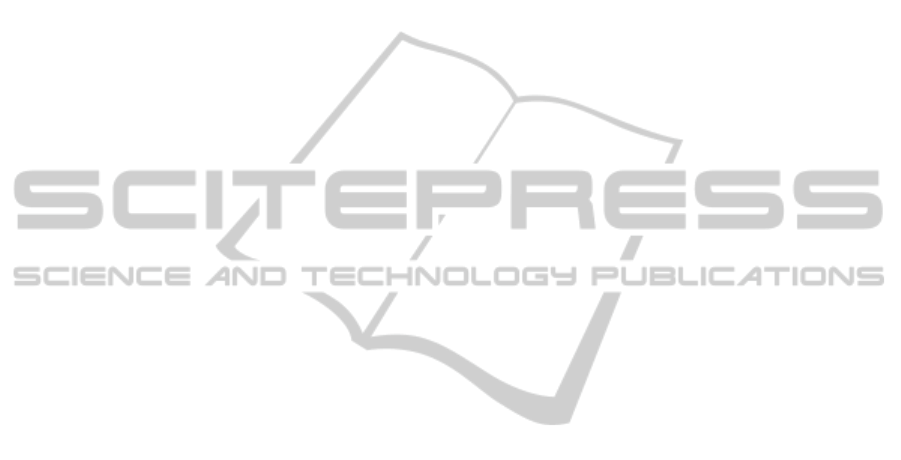
Management Science: 138-155.
Huber, G.P. (1984) “The Nature and Design of Post
Industrial Organisations”, Management Science, 30(8):
928-951.
Huber, G. P. and R. L. Daft (1987). "The information
environments of organizations."
Huber, G. P. and R. R. McDaniel (1986). "The Decision-
Making Paradigm of Organizational Design."
Management Science 32(5): 572-589.
Huseman, R. C. and Miles, E. W. (1988) “Organisational
Communication in the Information Age: Implications
of Computer-Based Systems”, Journal of
Management, 14(2): 181-204.
Ilmola, L. and O. Kuusi (2006). "Filters of weak signals
hinder foresight: Monitoring weak signals efficiently
in corporate decision-making." Futures 38(8): 908-
924.
Kabir, N., & Carayannis, E. (2013). Big Data, Tacit
Knowledge and Organizational Competitiveness.
Journal of Intelligence Studies in Business, 3(3).
Kakabadse,
N. K., Kouzmin, A. and Kakabadse, A.
(2001) “From tacit knowledge to knowledge
management: leveraging invisible assets”, Knowledge
and Process Management, 8(3): 137-154.
Kettinger, William J., and Yuan Li. "The infological
equation extended: towards conceptual clarity in the
relationship between data, information and
knowledge." European Journal of Information
Systems 19.4 (2010): 409-421.
Kirrane, D.E. (1999) “Getting wise to Knowledge
Management”, Association Management, 51(8): 31-
42.
LaValle, S., E. Lesser, R. Shockley, M. S. Hopkins and N.
Kruschwitz (2011). "Big data, analytics and the path
from insights to value." MIT Sloan Management
Review 52(2): 21-32.
Lycett, M. (2013). "‘Datafication’: making sense of (big)
data in a complex world." European Journal of
Information Systems 22(4): 381-386.
MacCrimmon, K.R. (1985) “Understanding strategic
decisions: three systematic approaches”, in Pennings
et al. (Eds), Organisational Strategy and Change,
Jossey-Bass Publishers, London.
Mason, R.O. and Mitroff, I.I. (1973) “A program for
research on management information systems”,
Management Science, 19: 475-485.
McAfee, A. and E. Brynjolfsson (2012). "Big data: the
management revolution." Harvard business review
90(10): 60-68.
McDermott, R. and O’Dell, C. (2001) “Overcoming
Cultural Barriers to Sharing Knowledge”, Journal of
Knowledge Management, 5(1): 76-85.
Mentzas, G. (1994). "A functional taxonomy of computer-
based information systems." International journal of
information management 14(6): 397-410.
Meredith, R. May, D. and Piorun, J. (2000) “Looking at
Knowledge in Three Dimensions”, IFIP TC8/WG8.3
International Conference on Decision Support through
Knowledge Management, 9-11 July 2000, Stockholm,
Sweden: 241-254.
Mintzberg, H. (1975) “The Manager’s Job: Folklore and
Fact”, Harvard Business Review, Jul./Aug.: 49-61.
Nonaka, I. and Takeuchi, H. (1995) The Knowledge-
Creating Company. Oxford University Press, New
York, NY.
O'Dell, C., Grayson, C.J.J. (1998) If Only We Knew What
We Know: The Transfer of Internal Knowledge and
Best Practice, The Free Press, New York, NY.
O’Flaherty, B. and Heavin, C.(2014), “Positioning
Predictive Analytics – A Design Evaluation”, DSS 2.0
– Supporting decision making with new technologies
IFIP WG 8.3 June 2014, Paris, France.
Oxford English Dictionary of Current English (Compact)
(2005), Third Edition ISBN-13: 978-0-19-861022-9.
Pfeffer, J. and R. I. Sutton (2006). Evidence-Based
Management. Harvard Business Review 84(1): 62-74.
Polanyi, M. (1966) The Tacit Dimension. Routledge and
Kegan Paul, London, UK.
Porter, M. and V. Millar (1985). How information changes
the way you compete. Harvard Business Review: 149-
160.
Provost, F., and Fawcett, T. 2013. “Data Science and Its
Relationship to Big Data and Data-Driven Decision
Making.” Big Data 1(1):51–59.
Rahman, N., F. Aldhaban and S. Akhter (2013). Emerging
technologies in business intelligence. Technology
Management in the IT-Driven Services (PICMET),
2013 Proceedings of PICMET'13:, IEEE.
Ross, Jeanna W., Cynthia M. Beath, and Anne Quaadgras.
2013. You May Not Need Big Data After All.
December. http://hbr.org/2013/12/you-may-not-need-
big-data-after-all/ar/1.[last accessed 20/6/14]
Spender, J.C. (2004) “Knowledge Fields: Some Post -9/11
Thoughts about the Knowledge Based Theory of the
Firm”, The Handbook of Knowledge Management
Volume 1, Eds. C.W. Holsapple, Verlanger: Berlin:
59-72.
Speier, C. (2006). "The influence of information
presentation formats on complex task decision-making
performance." International Journal of Human-
Computer Studies 64(11): 1115-1131.
Speier, C. and M. G. Morris (2003). "The influence of
query interface design on decision-making
performance." MIS Quarterly 27(3): 397-423.
Starbuck, W. H. (1976) “Organisations and their
environments”, in Dunnette (Ed.) Handbook of
Industrial and Organisational Psychology, Rand
McNally College Publishing Company, Chicago:
1069-1123.
Stein, E.W. and Zwass, V. (1995) “Actualizing
organizational memory with information systems”,
Information Systems Research, 6(2): 85-118.
Tata Consulting Services (TCS) (2013) “Managing
Knowledge from Big Data Analytics in Product
Development”http://www.tcs.com/SiteCollectionDocu
ments/White%20Papers/Knowledge-Big-Data-Analy
tics-Product-Development-1213-1.pdf [last accessed
20/6/14]
Wiig, K.M. (1993) Knowledge Management Foundations
– Thinking About Thinking – How People and
KMIS2014-InternationalConferenceonKnowledgeManagementandInformationSharing
296