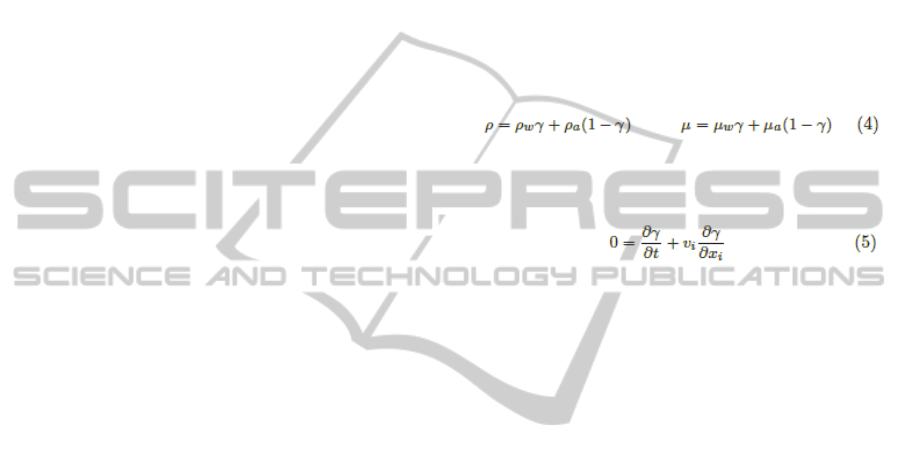
that typically occurs at the transom of a boat like the
Laser. Therefore, this turbulence model seems to be
well suited for the current CFD application..
EAS Model. The Explicit Algebraic Stress
Model (EASM) proposed in Wallin and Johansson
(2000) provides an alternative to linear eddy
viscosity models (such as the SST k-ω) based on the
Boussinesq hypothesis. Often, linear eddy viscosity
models fail to offer satisfactory predictions for
complex three-dimensional flows, due to the
involvement of the Boussinesq assumption. This
leads to nonlinear stress-strain relations in
turbulence modelling that contradict the Boussinesq
assumption. (Gatski and Speziale, 1993).
Nevertheless, owing to their high level of stability,
these linear eddy viscosity models are commonly
used in the industry (Versteeg and Malalasekera,
2007).
The original algebraic stress model (ASM)
model is not often used as a result of robustness
issues and frequent instances of singular behaviour
(Deng, Queutey, and Visonneau, 2005), both of
which the EAS Model addresses by suggesting
treatment of the non-linear term by the production-
to-dissipation rate ratio, and the number of tensor
bases used to represent the explicit solution of those
equations. Gatski and Speziale (Gatski and Speziale,
1993) have identified an exact solution for three-
dimensional flow involving a ten-term tensor, but
require too much computational power. Alternatives
discerns that five terms yields acceptable
approximation of the solution to the algebraic stress
equation (Deng, Queutey, and Visonneau, 2005).
2.3 The Volume of Fluid Method, VOF
The VOF method is a multiphase flow method that
computes the interaction of several fluids or phases
of a fluid present in the same domain, and obtains
the interface between these fluids (Marek,
Aniszewski and Boguslawski, 2008). For the
purposes of yachting applications, implementation
of this method allows for the accurate inclusion of
the computation of the free water surface around the
hull of the yacht.
The VOF method calls for the solving of, the
same Navier-Stokes equation as do single-phase
flows. The difference lies in a phase indicating
function γ (Hirt and Nichols, 1979). This phase,
called the colour function or volume fraction,
displays the measure of the mixture of phases in
each cell. For instance, if γ = 1, the cell is
completely occupied by phase one, and if γ = 0.3,
30% of phase one and 70% of phase two are present
in the cell. In terms of yachting applications, the two
present fluids are water and air. As air is included in
this method, the spatial discretization must extend
above the waterline as well. This does, of course,
increase the computational effort of the simulation,
but it offers a significantly more accurate physical
representation of the waves, as will be explained
below.
The physical fluid properties used in the Navier-
Stokes equation for a multiphase flow is a blend of
the properties of the present fluids. In the case of
yachting, in which the present fluids are air and
water, the computational properties are blended in
the following manner:
To track the motion of the interface, a separate
transport equation for the colour function is used:
This method does, however, give rise to a
numerical problem regarding the smearing of
boundaries between the phases over several cells.
This smearing denotes that the water surface is
constituted by a gradual change in density between
water and air. As the water surface is a discontinuity,
a jump in density, this smearing represents an
unwanted phenomenon. It is a result of the
convective averaging being conducted across the
water surface. The remedy for this smearing is to
implement, in the code, a way to detect the presence
of a boundary (Hirt and Nichols, 1998) and treat the
bounded areas separately. In the Shipflow software,
the smearing problem is addressed by
implementation of a compressive discretization
scheme, as suggested by Orych, Larsson and
Regnstrom (2010).
To render visible the surface of the water, the
distribution of the colour function is evaluated.
Where 0<γ<1 there is a mixture between the fluids
and the free water surface is found. As mentioned,
however, the boundary between the phases may be
smeared, and therefore a specific value of γ is
selected to display the surface.
The VOF method belongs to the class of surface
capturing methods. In such methods, the interface
between two fluids is computed somewhere inside
the domain. The main difference from single-phase
surface tracking methods is that, in this case, the
dynamics of the air are also computed. In single-
phase methods, the water surface geometry forms
the top boundary of the domain, and thus these
icSPORTS2014-InternationalCongressonSportSciencesResearchandTechnologySupport
194