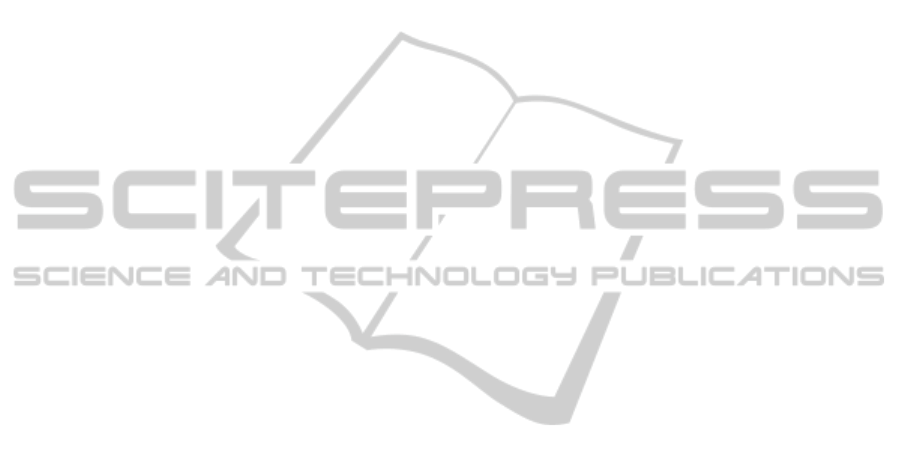
good correlation both individually and for the total
data set. The assessment of the RS2 may be useful for
the non-invasive assessment of PEP and LVET during
exercise. Heart sound amplitude was also observed to
be increased with stress, the result of the cardiovascu-
lar response to the extra need for oxygenated blood in
the muscles during exercise, leading to an increase in
CO, HR and BP.
S2/S1 was decreased with increasing exercise,
and although HFC of S2 tend to be higher than of S1,
in this study we observed that during a stress test this
relation may be altered, leading to the need of differ-
ent features in the discrimination of S1 and S2 than
those usually proposed.
The description of these features allows a more
comprehensive assessment of the cardiovascular sys-
tem, and the dynamic changes that occur during a
stress test, paving the way for future studies, includ-
ing the assessment of these variables with blood pres-
sure measurement and simultaneous pulse wave am-
plitude analysis (vascular transit time).
ACKNOWLEDGEMENTS
This work was partially funded by the Fundac¸
˜
ao
para a Ci
ˆ
encia e Tecnologia (FCT, Portuguese Foun-
dation for Science and Technology) under the ref-
erence Heart Safe PTDC/EEI-PRO/2857/2012; and
Project I-CITY - ICT for Future Health/Faculdade de
Engenharia da Universidade do Porto, NORTE-07-
0124-FEDER-000068, funded by the Fundo Europeu
de Desenvolvimento Regional (FEDER) through the
Programa Operacional do Norte (ON2) and by na-
tional funds through FCT/MEC (PIDDAC); and the
french telemedicine project E-Care.
REFERENCES
Assous, S. and Boashash, B. (2012). Evaluation of the mod-
ified s-transform for time-frequency synchrony analy-
sis and source localisation. EURASIP Journal on Ad-
vances in Signal Processing, 2012(1).
Bartels, A. and Harder, D. (1992). Non-invasive determina-
tion of systolic blood pressure by heart sound pattern
analysis. Clinical Physics and Physiological Mea-
surement, 13(3):249 –256.
Biswal, M. and Dash, P. (2013). Detection and characteriza-
tion of multiple power quality disturbances with a fast
s-transform and decision tree based classifier. Digital
Signal Processing, 23(4):1071 – 1083.
Carvalho, P., Paiva, R., Couceiro, R., Henriques, J., An-
tunes, M., Quintal, I., Muehlsteff, J., and Aubert, X.
(2010). Comparison of systolic time interval measure-
ment modalities for portable devices. In Engineer-
ing in Medicine and Biology Society (EMBC), 2010
Annual International Conference of the IEEE, pages
606–609.
Chandrasekaran, V., Dantu, R., Jonnada, S., Thiyagaraja,
S., and Subbu, K. (2013). Cuffless differential blood
pressure estimation using smart phones. Biomedi-
cal Engineering, IEEE Transactions on, 60(4):1080–
1089.
Dennis, A., Michaels, A. D., Arand, P., and Ventura, D.
(2010). Noninvasive diagnosis of pulmonary hyper-
tension using heart sound analysis. Computers in Bi-
ology and Medicine, 40(9):758 – 764.
Felner, J. M. (1990a). Clinical Methods: The History, Phys-
ical, and Laboratory Examinations, chapter 22. The
First Heart Sound. CRC Press, Boston: Butterworths.
Felner, J. M. (1990b). Clinical Methods: The History, Phys-
ical, and Laboratory Examinations, chapter 23. The
Second Heart Sound. CRC Press, Boston: Butter-
worths.
Guyton, A. and Hall, J. E., editors (2006). Textbook of Med-
ical Physiology. Elsevier Saunders, 11th edition.
Hansen, J., Zimmermann, H., Schmidt, S., Hammershoi,
D., and Struijk, J. (2011). System for acquisition of
weak murmurs related to coronary artery diseases. In
Computing in Cardiology, 2011, pages 213–216.
Kohler, B.-U., Hennig, C., and Orglmeister, R. (2002). The
principles of software qrs detection. Engineering in
Medicine and Biology Magazine, IEEE, 21(1):42–57.
Moukadem, A., Dieterlen, A., Hueber, N., and Brandt, C.
(2013). A robust heart sounds segmentation module
based on s-transform. Biomedical Signal Processing
and Control, 8(3):273 – 281.
Paiva, R., Carvalho, P., Aubert, X., Muehlsteff, J., Hen-
riques, J., and Antunes, M. (2009). Assessing pep and
lvet from heart sounds: Algorithms and evaluation. In
Engineering in Medicine and Biology Society, 2009.
EMBC 2009. Annual International Conference of the
IEEE, pages 3129–3133.
Pinnegar, C. R. and Mansinha, L. (2003). The s-transform
with windows of arbitrary and varying shape. Geo-
physics, 68(1):381–385.
Pluim, B. M., Zwinderman, A. H., van der Laarse, A., and
van der Wall, E. E. (2000). The athletes heart: A meta-
analysis of cardiac structure and function. Circulation,
101(3):336–344.
Ronved, S., Gjerlov, I., Brokjaer, A., and Schmidt,
S. (2011). Phonocardiographic recordings of
first and second heart sound in determining the
systole/diastole-ratio during exercise test. In Drem-
strup, K., Rees, S., and Jensen, M., editors, 15th
Nordic-Baltic Conference on Biomedical Engineer-
ing and Medical Physics (NBC 2011), volume 34 of
IFMBE Proceedings, pages 85–88. Springer Berlin
Heidelberg.
Smith, R. and Ventura, D. (2013). A general model
for continuous noninvasive pulmonary artery pres-
sure estimation. Computers in Biology and Medicine,
43(7):904 – 913.
AnalysisoftheElectromechanicalActivityoftheHeartfromSynchronizedECGandPCGSignalsofSubjectsUnderStress
55