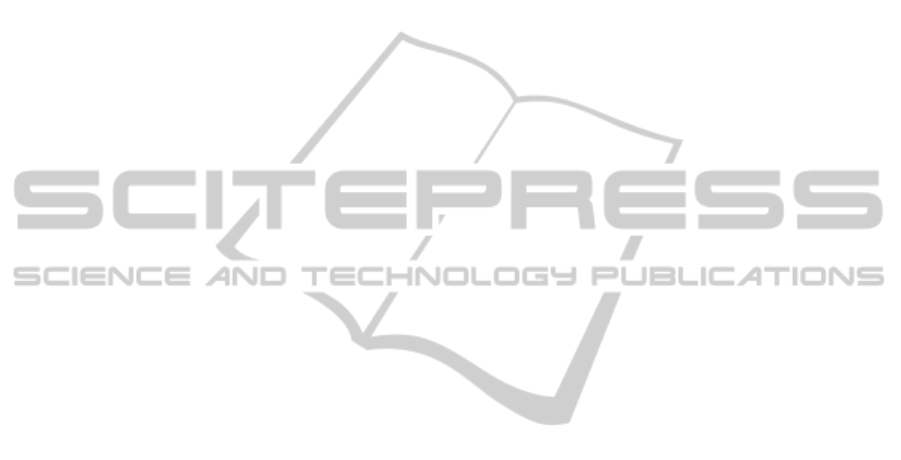
opments based on current restrictions and conditions.
2 BACKGROUND
Diagnosis-related groups (DRGs) is a patient classifi-
cation system that links similar types of cases that a
hospital treats, to the resources that the hospital uses
for the treatment. DRG systems were pioneered in
the USA, but are now in use in many other countries
(Schrey
¨
ogg et al., 2006).
The SwissDRG system is a variation of German
DRG but refers to Swiss reference values (Swiss
DRG, Web). Like in all DRG systems, single cases
are classified into a specific class according to their
diagnoses (using ICD10), treatment types (e.g. hip
surgery), and other case attributes. Each group has a
unique code, which enables a precise attribution of a
case’s revenues to its costs. This results in a shift from
a more cost-oriented daily allowance to a product-
oriented view, where optimal management along the
patient pathway gains in relevance (e.g. in (Gocke
et al., 2002) and (Rohner, 2012)). This shift opens
the potential for providing a basis for evidence-based
decisions in an operative and above all in a strategic
perspective.
The dimensions of interest for strategic analysis
are: average length of stay, cost weights, and the over-
all base rate. The average length of stay (ALOS) is
used in a DRG system as an output measurement for
complexity of the case (Luke, 1979). The other di-
mension is the cost weight, a relative indicator for
the severity of the case, which serves as a measure
for resource intensity. Cost weight is recalculated ev-
ery year based on cost data provided by hospitals to
the Case Mix Office (i.e. SwissDRG in Switzerland).
Each DRG is assigned specific values for these two in-
dicators, and all the values for all the DRGs are stored
in a reference catalog.
The base rate (which is basically determined by
negotiation between the hospital and insurance com-
panies) is multiplied with the cost weight attribute to
determine the monetary value of a case that is clas-
sified into a particular DRG. Besides revenues from
private insurance and specialized pharmaceuticals or
technologies for special treatments (e.g. specialized
oncological treatments) these revenues represent the
main income that a hospital can generate from their
patients.
As theoretically the cases behind any DRG code
should on average represent similar cases in each hos-
pital, these elements make hospitals comparable and
allow a benchmark-oriented approach. Comparisons
can be achieved by using one hospital’s cases, and
then comparing them either with the reference values
of the DRG catalog, or to the portfolio of a peer hos-
pital, both at the service and at the cost level. Most
importantly, this allows comparing the efficiency of
hospitals, but it will also enable quality or other as-
sessments.
The services that a hospital offers are influenced
by many factors. In most administrative districts (i.e.
in our Swiss case corresponding to cantons), service
portfolios or service requirements are defined by pol-
icy makers. However not all districts decide to pro-
vide a full scope of medical services, but might del-
egate the remaining activities to other providers that
can be situated beyond their control or area of di-
rect responsibility. This is complicated by the fact
that with the new reimbursement system, patients also
gained the freedom to choose their hospital of treat-
ment independently of their canton of residency.
On top of that, there are a number of national poli-
cies that impose further restrictions, e.g., the highly
specialized medicine act that restricts the number of
centers for very complicated treatments, or the chang-
ing outpatient health care provision that still heav-
ily depends on resident physicians, who are however
overaged and struggle to find replacing practice hold-
ers. All these factors push hospitals towards stratify-
ing patient portfolios and focusing on selected medi-
cal areas, in order to improve their economic situation
actively.
Modern hospitals must engage in strategic discus-
sions about visions, cooperations, specialization, cen-
tralization and further infrastructure or organization-
ally relevant questions. These decisions affect their
market position and help them to cope with growing
and dynamic competitors both at administrative dis-
trict or regional levels.
For example, hospitals that today provide maxi-
mal service levels, will have to decide whether they
will focus on more specific therapies that generate
high cost weights (L
¨
ungen and Lauterbach, 2002), or
whether they will continue offering a broad range of
health care services, focusing on a me-too strategy.
The analysis of pancreas and esophagus carci-
noma surgery in Germany as another example has
shown that it can be highly beneficial for hospitals to
treat a high number of benign cases instead of focus-
ing on the malign cases with high cost weights but bad
average length of stay management. This is the case
even if the minimal numbers are reached that would
be required by the official rules and regulations.
Ultimately, Swiss hospitals will have to adapt their
current management models. In order to take the right
decisions in this complex environment, hospital man-
agers need to rely on data, and models that are based
StrategicPlanningofHospitalServicePortfolios-TheDRGeeViewer
83