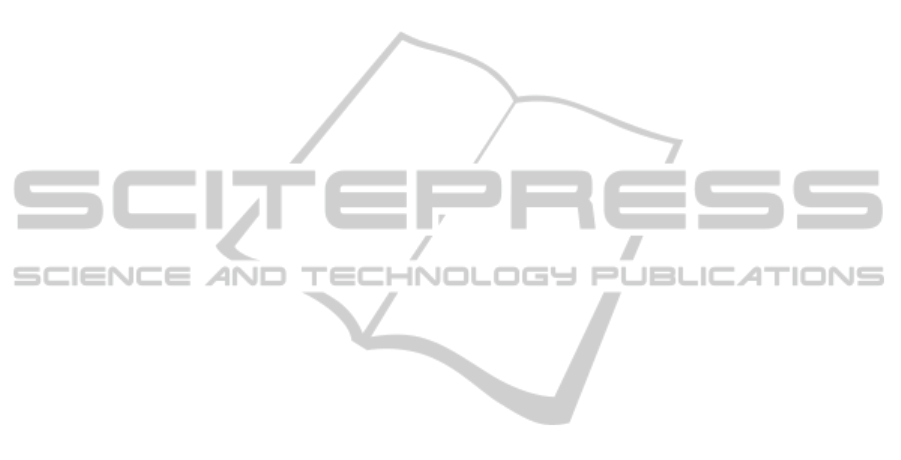
Bonner, R., & Nossal, R. (1981). Model for laser Doppler
measurements of blood flow in tissue. Applied optics,
20(12), 2097-2107.
Fredriksson, I., Fors, C., & Johansson, J. (2007). Laser
doppler flowmetry-a theoretical framework.
Department of Biomedical Engineering, Linköping
University.
Rowell, L. B. (1986). Human circulation: regulation
during physical stress (p. 215). Oxford: Oxford
University Press.
Smith, J. J. (1990). Circulatory response to the upright
posture (No. 6). CRC Press.
Yamamoto, Y., & Hughson, R. L. (1991). Coarse-graining
spectral analysis: for studying heart rate variability.
Penaz, J. (1978). Mayer waves: history and methodology.
Automedica, 2(3), 135-142.
Söderström, T., Stefanovska, A., Veber, M., & Svensson,
H. (2003). Involvement of sympathetic nerve activity
in skin blood flow oscillations in humans. American
Journal of Physiology-Heart and Circulatory
Physiology, 284(5), H1638-H1646.
Nogami, H., Iwasaki, W., Abe, T., Kimura, Y., Onoe, A.,
Higurashi, E., ... & Sawada, R. (2011). Use of a simple
arm-raising test with a portable laser Doppler blood
flow meter to detect dehydration. Proceedings of the
Institution of Mechanical Engineers, Part H: Journal
of Engineering in Medicine, 225(4), 411-419.
Iwasaki, W., Nogami, H., Ito, H., Gotanda, T., Peng, Y.,
Takeuchi, S., ... & Sawada, R. (2012). Useful method
to monitor the physiological effects of alcohol
ingestion by combination of micro-integrated laser
Doppler blood flow meter and arm-raising test.
Proceedings of the Institution of Mechanical
Engineers, Part H: Journal of Engineering in
Medicine, 226(10), 759-765.
Ogata, H., Fujimaru, I., Yamada, K., & Kondo, T. (2012).
Suppression of cardiocirculatory responses to
orthostatic stress by passive walking-like leg
movement in healthy young men. J Physiol Anthropol,
31, 24.
Hess, W. R. (1954). Diencephalon, autonomic and
extrapyramidal functions (Vol. 3). Grune & Stratton.
Ohhashi, T., Sakaguchi, M., & Tsuda, T. (1998). Human
perspiration measurement. Physiological
measurement, 19(4), 449.
Homma, S., Nakajima, Y., Toma, S., Ito, T., & Shibata, T.
(1998). Intracerebral source localization of mental
process-related potentials elicited prior to mental
sweating response in humans. Neuroscience letters,
247(1), 25-28.
Tanaka, H., Yamaguchi, H., Matushima, R., & Tamai, H.
(1999). Instantaneous orthostatic hypotension in
children and adolescents: a new entity of orthostatic
intolerance. Pediatric research, 46
(6), 691-691.
Low, P. A., Opfer-Gehrking, T. L., Textor, S. C.,
Benarroch, E. E., Shen, W. K., Schondorf, R., ... &
Rummans, T. A. (1995). Postural tachycardia
syndrome (POTS). Neurology, 45(4 Suppl 5), S19-25.
Wheeler, T., & Watkins, P. J. (1973). Cardiac denervation
in diabetes. British Medical Journal, 4(5892), 584.
Oka, H., Mochio, S., Sato, K., Sato, H., Katayama, K.,
Watanabe, S., ... & Isogai, Y. (1995). Spectral
analyses of RR interval and systolic blood pressure in
diabetic autonomic neuropathy. Journal of the
autonomic nervous system, 52(2), 203-211.
Bonner, R. F., Clem, T. R., Bowen, P. D., & Bowman, R.
L. (1981). Laser-Doppler continuous real-time monitor
of pulsatile and mean blood flow in tissue
microcirculation. In Scattering Techniques Applied to
Supramolecular and Nonequilibrium Systems (pp.
685-701). Springer US.
Kano, T., Shimoda, O., Higashi, K., Sadanaga, M., &
Sakamoto, M. (1993). Fundamental patterns and
characteristics of the laser-Doppler skin blood flow
waves recorded from the finger or toe. Journal of the
autonomic nervous system, 45(3), 191-199.
Abe, M., Ando, Y., Higashi, K., & Kano, T. (1996). Non-
neurogenic periodic fluctuations in heart rate and
vasomotion appearing in familial amyloid
polyneuropathy (FAP) Type I (Met30). Journal of the
autonomic nervous system, 60(1), 71-75.
Ide, J., Yamaga, M., Kitamura, T., & Takagi, K. (1997).
Quantitative evaluation of sympathetic nervous system
dysfunction in patients with reflex sympathetic
dystrophy. The Journal of Hand Surgery: British &
European Volume, 22(1), 102-106.
Ando, Y., Araki, S., Shimoda, O., & Kano, T. (1992).
Role of autonomic nerve functions in patients with
familial amyloidotic polyneuropathy as analyzed by
laser Doppler flowmetry, capsule hydrograph, and
cardiographic R–R interval. Muscle & nerve, 15(4),
507-512.
Mano, T. (1990). Sympathetic nerve mechanisms of
human adaptation to environment—findings obtained
by recent microneurographic studies. Environ. Med,
34, 1-35.
BIOSIGNALS2015-InternationalConferenceonBio-inspiredSystemsandSignalProcessing
218