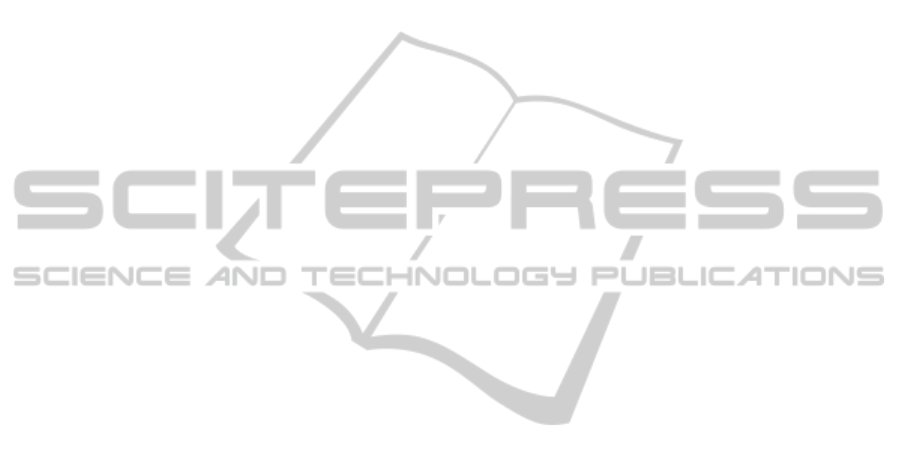
Bansal, N., Mims, J., Kuremsky, J., Olex, A., Zhao, W., Yin,
L., Wani, R., Qian, J., Center, B., Marrs, G., Poros-
nicu, M., Fetrow, J., Tsang, A., and Furdui, C. (2014).
Broad phenotypic changes associated with gain of ra-
diation resistance in head and neck squamous cell can-
cer. Antioxid Redox Signal., 21(2):221–236.
Beissbarth, T. and Speed, T. (2004). GOstat: find statisti-
cally overrepresented gene ontologies within a group
of genes. Bioinformatics., 197(1):1464–1465.
Bendl, J., Stourac, J., Salanda, O., Pavelka, A., Wieben, E.,
Zendulka, J., Brezovsky, J., and Dombrsky, J. (2014).
PredictSNP: Robust and accurate consensus classi-
fier for prediction of disease-related mutations. PLoS
Comput Biol., 10(1):e1003440.
Bhattacharya, A., Ziebarth, J., and Cui, Y. (2014).
PolymiRTS database 3.0: linking polymorphisms in
microRNAs and their target sites with human dis-
eases and biological pathways. Nucleic Acids Res.,
42(D1):D86–D91.
Budworth, H., Snijders, A., Marchetti, F., Mannion, B.,
Bhatnagar, S., Kwoh, E., Tan, Y., Wang, S., Blakely,
W., Coleman, M., Peterson, L., and Wyrobek, A.
(2012). Dna repair and cell cycle biomarkers of ra-
diation exposure and inflammation stress in human
blood. PLoS One, 7(11):e48619.
Bush, W. and Moore, J. (2012). Chapter 11: Genome-wide
association studies. PLoS Comput Biol., 8(12).
Chen, F., Zhou, C., Lu, Y., Yuan, L., Peng, F., Zheng, L.,
and Li, X. (2013). Expression of hsa-mir-186 and its
role in human colon carcinoma cells. Nan Fang Yi Ke
Da Xue Xue Bao, 33(5):654–60.
Chen, F., Zhu, H., Zhou, L., Wu, S., Wang, J., and Chen, Z.
(2010). Inhibition of c-flip expression by mir-512-3p
contributes to taxol-induced apoptosis in hepatocellu-
lar carcinoma cells. Oncology Reports, 23(5):1457–
1462.
Davis, F., Azimi, I., Faville, R., Peters, A., Jalink, K.,
Putney, J., Goodhill, G., Thompson, E., Roberts-
Thomson, S., and Monteith, G. (2014). Induction
of epithelialmesenchymal transition (EMT) in breast
cancer cells is calcium signal dependent. Oncogene.,
33:2307–2316.
Deveci, M., Catalyrek, U., Svoboda, M., and Toland,
A. (2014). mrSNP: Software to detect SNP ef-
fects on microRNA binding. BMC Bioinformatics.,
15):doi:10.1186/1471–2105–15–73.
Dhillon, A., Hagan, S., Rath, O., and Kolc, W. (2007).
MAP kinase signalling pathways in cancer. Onco-
gene., 26:3279–3290.
Dong, H., Lei, J., Ding, L., Wen, Y., Ju, H., and Zhang, X.
(2013). MicroRNA: Function, detection, and bioanal-
ysis. Chem. Rev., 113(8):6207–6233.
Dweep, H., Sticht, C., Pandey, P., and Gretz, N. (2014).
miRWalk - database: prediction of possible mirna
binding sites by ’walking’ the genes of 3 genomes.
Nucleic Acids Research, 42:D78D85.
Edlund, K., Lindskog, C., Saito, A., Berglund, A., Pontn,
F., Gransson-Kultima, H., Isaksson, A., Jirstrm, K.,
Planck, M., Johansson, L., Lambe, M., Holmberg,
L., Nyberg, F., Ekman, S., Bergqvist, M., Lan-
delius, P., Lamberg, K., Botling, J., Ostman, A., and
Micke, P. (2012). CD99 is a novel prognostic stromal
marker in non-small cell lung cancer. Int J Cancer,
131(10):2264–2273.
Eke, I. and Cordes, N. (2014). Focal adhesion signaling and
therapy resistance in cancer. Semin Cancer Biol., pii:
S1044-579X(14)00098-4.
Evangelou, E. and Ioannidis, J. (2013). Meta-analysis meth-
ods for genome-wide association studies and beyond.
Nat. Rev. Genet., 14:379389.
Finnon, P., Robertson, N., Dziwura, D., Raffy, C., Zhang,
W., Ainsbury, L., Kaprio, J., Badie, C., and Bouf-
fler, S. (2008). Evidence for significant heritability
of apoptotic and cell cycle responses to ionising radi-
ation. Hum Genet., 123(5):485–493.
Griffiths-Jones, S., Saini, H., van Dongen, S., and Enright,
A. (2008). mirbase tools for microrna genomics. Nu-
cleic Acids, 36:154–158.
Han, X., Xue, L., Zhou, L., Gong, L., Zhu, S., Yao, L.,
Wang, S., Lan, M., Li, Y., and Zhang, W. (2013). The
role of ptpn13 in invasion and metastasis of lung squa-
mous cell carcinoma. Exp Mol Pathol., 95(3):270–58.
Hatakeyamai, S. (2011). TRIM proteins and cancer. Nat
Rev Cancer, 11(11):792–804.
Hirshoren, N., Bulvik, R., Neuman, T., Rubinstein, A.,
Meirovitz, A., and Elkin, M. (2014). Induction of hep-
aranase by HPV E6 oncogene in head and neck squa-
mous cell carcinoma. J Cell Mol Med., 18(1):181–
186.
Hsu, S., Tseng, Y., Shrestha, S., Lin, Y., Khaleel, A., Chou,
C., Chu, C., Huang, H., Lin, C., Ho, S., Jian, T.,
Lin, F., Chang, T., Weng, S., Liao, K., Liao, I., Liu,
C., and Huang, H. (2014). MiRTarBase update 2014:
an information resource for experimentally validated
miRNA-target interactions. Nucleic Acids Research,
42:D78D85.
Jianq, N., Zhu, S., Chen, J., Niu, Y., and Zhou, L. (2013).
A-methylacyl-CoA racemase (AMACR) and prostate-
cancer risk: a meta-analysis of 4,385 participants.
PLoS One., 8(10):e74386.
Kabacik, S., Mackay, A., Tamber, N., Manning, G., Finnon,
P., Paillier, F., Ashworth, A., Bouffler, S., and Badie,
C. (2011a). Gene expression following ionising radi-
ation: identification of biomarkers for dose estimation
and prediction of individual response. Int J Radiat
Biol., 87(2):115–129.
Kabacik, S., Ortega-Molina, A., Efayan, A., Finnon, P.,
Bouffler, S., Serrano, M., and Badie, C. (2011b). A
minimally invasive assay for individual assessment
of the atm/chek2/p53 pathway activity. Cell Cycle.,
10(7):1152–1161.
Kapoor, S. (2014). Maspin and its evolving role in tumor
progression in systemic malignancies. Breast Cancer.,
21(2):249.
Lahtz, C. and Pfeifer, G. (2011). Epigenetic changes of dna
repair genes in cancer. J Mol Cell Biol., 3(1):51–55.
Lewis, B., Burge, C., and Bartel, D. (2005). Conserved
seed pairing, often flanked by adenosines, indicates
IstheIdentificationofSNP-miRNAInteractionsSupportingthePredictionofHumanLymphocyteTranscriptional
RadiationResponses?
249