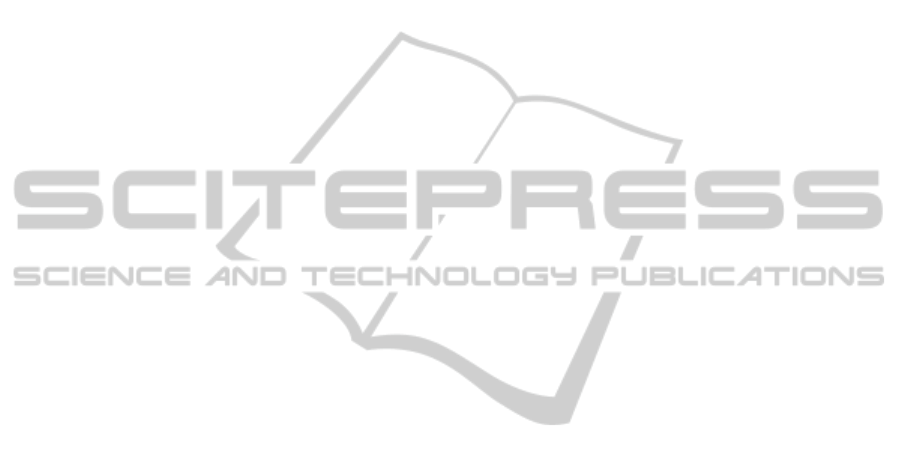
sults indicated that the incentives chosen for this sim-
ulation only had an effect for car users in the way
that they would be more willing to participate in car-
pooling. The fact that bike usage and walking did
not change substantially when incentives were intro-
duced could be due to the nature of these two modes:
they can be used flexibly, do not cost anything and
no ticket is needed for them, so the motivation to use
them might be different from e.g. car and public trans-
port.
Principally, this work substantiates the hypothesis
that agent-based demand simulation is a suitable in-
strument to examine the effects of TDM measures and
incentives in urban transportation in comparison and
combination. Compared to established TDM evalua-
tions, agent-based demand simulation investigates the
interactions of large numbers of entities in complex
transportation networks. Furthermore, daily routines
and situational effects like traffic congestion can be
incorporated into investigations. This adds an addi-
tional value for planning processes.
Nevertheless, there are some drawbacks. First, the
explanatory power of ABS approaches is substantially
dependent on the underlying data quality. To validate
an ABS in this scientific field, a widespread investi-
gation under realistic conditions (Jacobs et al., 1982)
is more suitable than an online survey.
In addition, demand simulation becomes more
complex in the area of monetary incentives or regu-
lations. Even though it is basically possible to equip
agents with budgets concerning agent modeling, our
focus groups showed that participants have problems
to specify a certain mobility budget. Moreover, focus
groups indicated the individual requirements concern-
ing specific measures.
In fact, findings like these underline the valuable
contribution of focus group studies already in the be-
ginning of modeling and simulation processes of hu-
man behavior and interactions.
5.1 Limitations and Future Work
Concerning the qualitative and quantitative surveys
on mobility choices, the data has limited significance
for very young or aged population groups, because
the study concentrated on young students. Besides
this, the results were not analyzed for specific user
groups, but only on an aggregated basis for the sample
as a whole. Future research should take into account
that user groups might differ in regard to the most ac-
cepted and most efficient incentive, which could then
also help to strategically tailor incentives to certain
groups of people.
This simulation worked with a simplified model-
ing of car pooling. There are some research efforts
to improve joint trip modeling further (Dubernet and
Axhausen, 2012), which would also be valuable to
implement.
Furthermore, scientific contributions show that
ABS, and especially MATSim, is suitable for
economic investigations, e.g. pricing measures
(Kickh
¨
ofer, 2009). Concerning this background, it
would be interesting to evaluate the demand effects
of mobility service bundling.
ACKNOWLEDGMENTS
This work was funded by the Excellence Initiative of
the German State and Federal Government (Project
Urban Future Outline). Thanks to Lino Kolb for re-
search assistance.
REFERENCES
Axelrod, R. (2006). Agent-Based Modeling as a Bridge
between Disciplines. In Tesfastian, L. and Judd,
K. L., editors, Handbook of Computational Eco-
nomics, pages 1565–1638. Elsevier, Amsterdam.
Ben-Akiva, M. and Atherton, T. J. (1977). Methodology
for short-range travel demand predictions: analysis
of carpooling incentives. Journal of Transport Eco-
nomics and Policy, pages 224–261.
Dubernet, T. and Axhausen, K. W. (2012). Including joint
trips in a multi-agent transport simulation. 12th Swiss
Transport Research Conference.
Ferguson, E. (1990). Transportation Demand Management.
Planning, Development and Implementation. Journal
of American Planning Association, 56(4):442–456.
Freudenstein, J. (2003). Agentenbasierte Simulation indi-
vidueller Tagesabl
¨
aufe (Agent Based Simulation of
Individual Daily Routines). Master’s thesis, RWTH
Aachen.
Hensher, D. A. and Pucket, S. M. (2007). Congestion
and variable user charging as an effective travel de-
mand management instrument. Transportation Re-
search Part A: Policy and Practice, 41(7):615–626.
Horni, A., Axhausen, K. W., and Nagel, K. (2011). High-
Resolution Destination Choice in Agent-Based De-
mand Models High-Resolution Destination Choice in
Agent-Based Demand Models. Technical report, Eid-
gen
¨
ossische Technische Hochschule Z
¨
urich, IVT, In-
stitut f
¨
ur Verkehrsplanung und Transportsysteme.
Ison, S. and Rye, T. (2008). TDM Measures and their Im-
plementation. In Ison, S. and Rye, T., editors, The Im-
plementation and Effectiveness of Transport Demand
Management Measures, pages 1–12. Ashgate Publish-
ing Limited, Hampshire, England.
Jacobs, H. E., Fairbanks, D., Poche, C. E., and Bailey, J. S.
(1982). Multiple Incentives in Encouraging Car Pool
SMARTGREENS2015-4thInternationalConferenceonSmartCitiesandGreenICTSystems
322