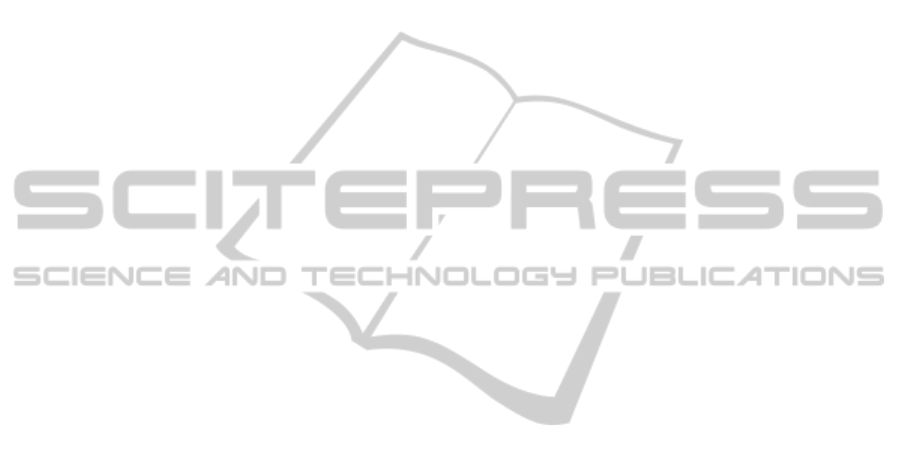
by several phenomena that happen inside the cars.
One of the causes is the time that takes for the
engine to reach its most fuel-efficient temperature,
warming up the vehicle before starting decreases the
efficiency as car is using fuel without moving.
Additionally, resources in comfort, such as heated
seats, window defrosters, and heater fans, requires
additional power (“Fuel Economy in Cold Weather,”
n.d.).
Authorities all over the globe are encouraging
users to switch to other modes through means of
promotion of energy efficient behaviour, including
energy efficient driving, car-pooling, car sharing,
and car-free zones/areas inside to cities (Danish
Ministry of Transport, 1996). But changes have to
break Travel Choice (TC) processes that are mainly
automatic, people only drive without considering
other alternatives and the cause of this is the
availability of a private vehicle, car ownership is the
principal determinant of car use (Sitlington, 1999;
Scheiner, 2010).
2.1.4 Infrastructure
Infrastructure refers to physical routes, buildings,
etc. that involve long-term capital investment and
determines the drive (car, bicycle etc.) conditions
during the whole year. Winter and spring are the
months for maintenance actions that influence
safety, accessibility, mobility and vehicle cost.
Winter maintenance operations represent a very
substantial portion of year-round maintenance costs
(Guo et al., 2007; Tyrinopoulos and Antoniou,
2013). In Canada $1,3 billion are used annually on
activities related with snow and ice control in public
roads (Leviäkangas et al., 2011). In Finland the cost
of maintenance during winter is 54% of the total
budget.
Additionally, the design of the infrastructure can
determinate the perception of the users. In the case
of PT, distance from start or destination point to
stops as well as the facilities during winter or
autumn (lights and shelter) can change travellers’
waiting and transfer experience. The greatest impact
of the infrastructure is in the mode choice. In
compact cities with high population density and low
available land, short trips are the main kind of trips
and use of PT, walking/cycling mode are the main
choices (Considine, 2000; Tyrinopoulos and
Antoniou, 2013; Scheiner and Holz-Rau, 2007).
2.1.5 Cost and Income
Relative cost of transport modes is an important
factor in TC. In the case of PT, the ticket price
usually reflects the cost of the system. Instead,
private car price is no clear, as it does not include all
their external cost, part of the unclearness comes
from the fact that most of that cost is subsidy for
local governments, representing 7.3% of the
European gross domestic product (Sitlington, 1999).
On the other hand, household income defines the
availability of private car. Results from Mobility
Management and housing program (2008) shows
that higher average income increases the number of
cars per house and their use by 34%. In comparison,
modal split with ALM and PT decreases in higher
proportion (de Jong and van de Riet, 2008;
Tyrinopoulos and Antoniou, 2013; Mobility
Management and housing, 2008).
2.1.6 Trip Characteristics
Some trip characteristics such as trip length, time
flexibility and trip purpose may affect the weather
impact on user’s TC. Long travel distances are more
sensitive to weather because of the exposure time.
Short trip times are less sensitive to weather
conditions. Important trip purpose (e.g. work) might
be more sensitive to weather than leisure ones (Guo
et al., 2007).
Trip length and time travelled are mainly defined
by infrastructure configurations. Basic facilities in
suburban areas such as the closest grocery, can
determinate the TC. If the perception of the distance
is high, car is generally accepted, in contrary, if the
distance is short, the use of bicycle or walk is
acceptable (Scheiner and Holz-Rau, 2007). M. Sabir
et.al. (2008) shows that an additional kilometre of
distance increases car use by 26,7% and PT with
2,2%, contrary to walking and cycling that decrease
by 23,1% and 7,4% respectively (Sabir et al., 2008)
Additionally, TC decisions are mainly done at home
and at work, so land design patterns between these
two destinations are crucial (Tyrinopoulos and
Antoniou, 2013; Mobility Management and housing,
2008; Frank et al., 2007).
2.1.7 Information
At the present time a considerable amount of
information is exchanged from transport system
consultation. Some of that information tried to
motivate car users to switch to PT by empowering
them with localised and advice information about
ALM/PT and leaving the choice to them
(Department of Transport, Australia, 2013). They
found that prioritized and effective distributed
information improves user’s perception (Sitlington,
1999; Department of Transport, Australia, 2013;
ParametersAffectingtheEnergyPerformanceoftheTransportSectorinSmartCities
85