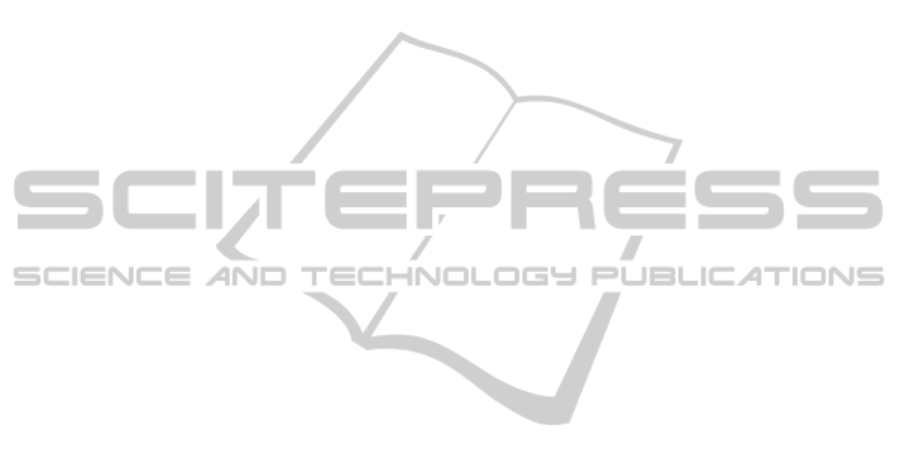
account operating conditions and design parameters.
This makes them not very suited for a good
performance analysis.
Dimensional analysis is a mathematical system
using conversion factor to move one unit of
measurement to a different unit of measurement
(Langhaar, 1951). The basic idea of dimensional
analysis is that physical laws do not depend on the
arbitraries in the choice of units of physical
quantities. Every physical equation or relation
between variables and/or dimensioned constants
should be dimensionally consistent. In other words,
each term of the equation or relation should have the
same dimensions. Dimensional consistency imposes
a certain number of constraints that are functional
relations between the variables. This constitutes the
main principle for dimensional analysis.
Manipulating variables to create dimensionless
groups or numbers to describe the physical
phenomenon has widely been used in the chemical
engineering or fluid mechanics field such as
Reynolds number (Re) to describe the type of flows
in all types of fluid problems, Froude number (Fr),
for modeling flow with a free surface, or Nusselt
(Nu), Biot (Bi), Peclet (Pe) for heat transfers or
Carnot (ƞ) for energy efficiency. Hence, it is a
pertinent idea to create performance indicators based
on dimensional analysis.
Pulp and paper industries are driven by steam,
water and chemicals which makes them suitable for
exergy studies. Exergy analysis is a valuable tool to
evaluate the efficiency of a process. However, it has
not evolved into a systematic method, such as Pinch
Analysis or Water Pinch and has not been applied on
a real Canadian Kraft mill, in combination with
other tools for equipment performance analysis.
Moreover, traditional energy studies only consider
thermal energy. Exergy analysis considers all forms
of energy and also the internal energy of the matter
called chemical exergy.
Most published studies on performance
evaluation analysis or energy improvement methods
are based on computer simulation models. A
recurrent problem of process simulation is the lack
of explanation or information of how the data, used
for all analyses, were gathered or treated. The
simulation models are often not based on real
reconciled mill data. There is no incentive in seeking
to optimize a model, when it does not match the
actual behavior of the real plant. A representative
model based on reconciled data is a prerequisite step
to any optimization or evaluation measure.
However, lack of data redundancy in real Kraft mills
has made data reconciliation complicated or
unfeasible. No data reconciliation of a complete
operating Canadian Kraft mill has been published.
There have been studies on data reconciliation on
Canadian newsprint mills, but never on a real Kraft
mill (Bellec et al., 2007); (Jacob and Paris, 2003).
4 METHODOLOGY
To perform a complete equipment performance
evaluation, the overall unified methodology shown
in figure 8 is developed and applied. It consists of 6
main steps. The first step is to obtain a coherent
model simulation that represents a steady-state of the
process. To do so, real mill data collection, gross
error detection and data reconciliation have been
performed. Mill measurements data are collected for
a chosen period of time. Since measurements
inherently contain random errors due to sensors
noise, the mass and balance around unit operations
often do not balance. Data reconciliation is an
optimization problem that aims to minimize the
weighted sum of squared differences between the
measured and the reconciled values under
constraints that correspond to mass and heat balance
(Bagajewicz, 2000); (Leibman et al., 1992); (Maquin
et al., 2000); (Maquin et al., 1989). On the other
hand, while DR is meant to correct random errors,
gross errors due to a sensor failure should be
detected first (Maronas and Arcas, 2009). This is
done by verifying that all measurements remain
within acceptable data range. Many statistical tests
have been developed. However, they have never
been applied on a real operating mill (Dewulf et al.,
2008); (Gong and Wall, 1997); (Gong and Wall,
2001); (Regulagadda et al., 2010); (Sato, 2004). The
results of the GED and DR show largely adjusted
areas. This helps identify possible process leaks or
biases present in the system (Krishnan-Dumitrescu,
2008). DR allows getting a coherent process model
that represents a steady-state of the studied mill and
also identifies a preliminary list of suspected
problematic unit operations. Largely adjusted areas
are highlighted for further analysis.
From the coherent steady-state of the process,
exergy analysis of individual unit operations and of
entire departments of the process has been
performed. Exergy is a measure of both quality and
quantity of the energy involved in transformations
within and across the boundaries of a system. Unlike
energy, exergy can be destroyed or lost, and thus
unavailable for future transformation with the
process system. Hence exergy analysis allows
SystematicEquipmentPerformanceAnalysisofCanadianKraftMillThroughNewandAdaptedKeyPerformance
Indicators-DoctoralConsortiumContributions
5