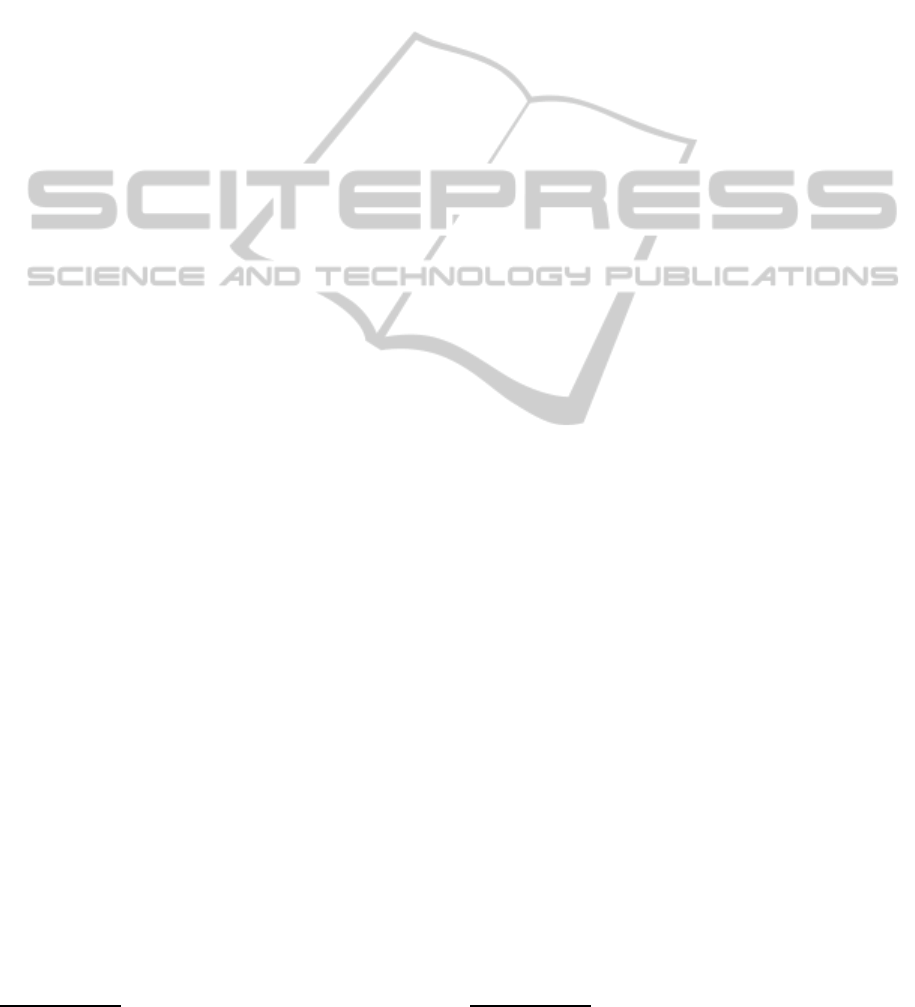
ment of the created platforms will be conducted.
The goal is to compare the effect of different feed-
back approaches on motivation and participation on
the platforms as well as measuring the effect on learn-
ing outcome of different LGC concepts.
3 STAGE OF THE RESEARCH
Currently, the development of appropriate question-
naires is ongoing. That includes performing inter-
views with representative users and building a model
of important factors influencing acceptance, motiva-
tion, contribution, quality and learning effect of LGC.
Development of PaperMesh, the LGC platform for
students to share scientific works, has started in win-
ter. An early beta version is expected for summer.
Also, a cooperation with the Berlin based startup
qLearning
3
has been established. The company is of-
fering a learning app, targeted at university students,
and wants to shift content creation to a learner gener-
ated model. The author consults in performing user
studies and will be granted access to study results and
usage data in return.
4 STATE OF THE ART
4.1 LGC Platforms
In classrooms and online courses, wikis and blogs are
a very common form of Learner Generated Content.
They can be used as a sandbox in which students sum-
marize what they learned or create articles on supple-
mental topics. Yet, wikis offer hardly any feedback
to contributors and don’t offer mechanisms to track
quality of content. Blogs usually have a comments
section but are very unidirectional.
To compare acceptance of Wikis with that of plat-
forms applying social feedback, the author will setup
off-the-shelf wikis for courses. Usage of these and of
other existing wikis will be evaluated as a baseline.
StackExchange.com is a collection of several
question and answer (Q&A) sites on topics such
as programming (stackoverflow.com), languages,
physics, math, cooking and many more. Questions
and answers are user generated, and a peer rating sys-
tem is used to rank questions and answers by qual-
ity. Users build up reputation points based on these
ratings and can follow a gamified path of levels and
badges awarded for their engagement. The StackEx-
change sites are very well implemented examples of
3
http://qlearning.de
how social feedback can be used to motivate users and
rank content by user voting.
While content is user generated, the Q&A tech-
nology behind this platform is held by stack exchange
inc. and is not publicly available. Accordingly this
platform cannot be customized or be used in closed
environments like classrooms. StackExchange plat-
forms can be used in institutional learning as a source
of information similar to Wikipedia. They are not pri-
marily designed or generally considered as eLearning
tools, though. Within this work, it is planned to test
the applicability of the Open Source Question An-
swer system (OSQA) in a course context. OSQA is
an open, but no longer maintained, reimplementation
of the StackExchange platform. It shall be measured
if social feedback in such a Q&A system yields better
acceptance or engagement than wikis.
On Graasp.eu
4
users can create private rooms for
their course or group. Within these rooms users pub-
lish text, images, videos or links and can rate other’s
content. It is intended for use in education contexts,
and its creators of EPFL Lausanne describe it as “a
social media platform, to setup a peer assessment ac-
tivity”. Graasp lacks any form of user profiles or gam-
ification and can be considered a content sharing plat-
form with ratings.
As part of this thesis the peer ranking platform
PearRank will be created, which will offer a similar
room based system as Graasp. In contrast to Graasp,
PearRank will put the social interaction and ranking
in focus. Graasp is more like a portfolio platform on
which users collect and share complementary infor-
mation. PearRank shall be more competitive as all
users upload similar content in response to a specific
task and vote to select the best.
4.2 Research
Wikis have been used and evaluated in various learn-
ing contexts and have become and essential part of
learning platforms such as Moodle. Some small stud-
ies have been done on the use of Wikis in higher edu-
cation courses, reporting positive feedback from stu-
dents, higher engagement and more social interaction
among participants. (Coutinho and Bottentuit Junior,
2007) (Wheeler et al., 2008)
Others argue, in these studies participation was
only achieved by pressure of points or grades, and
that students would not contribute voluntarily. (Ebner
et al., 2008)
Hardly any data is available on what students who
freely create content in Wikis or Blogs, or on how
those who don’t, can be motivated.
4
http://graasp.eu
LearnerGeneratedContent-FosteringandValuingUserGeneratedContentineLearningusingSocialFeedback
5