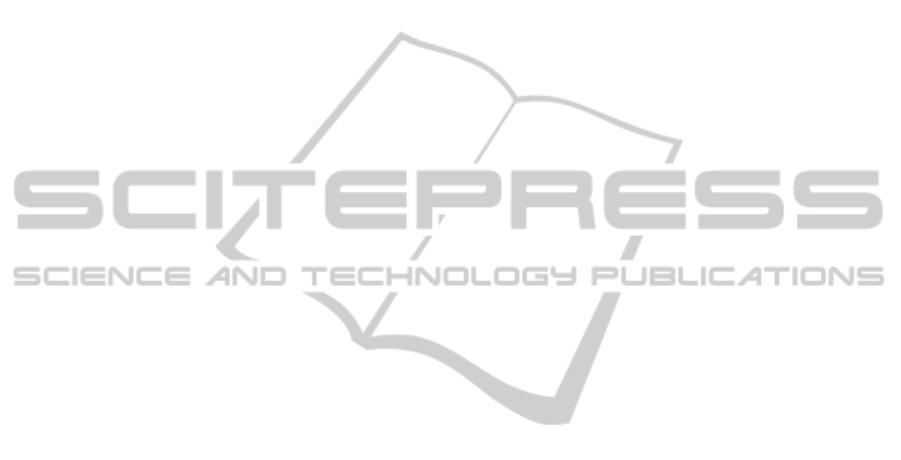
Finally, the algorithm to configure the virtual
power plant is partially completed. The first step, in
which the combination of energy generation plants
from fluctuating RES with the best match to the local
demand is selected, is to be perform analogously to
the decision algorithm presented by Ramirez
Camargo et al., (2015). The match of the demand is
not only determined with the amount of properly
supplied energy, but also with the excess energy
supplied to the local system. Plants contributing with
a high amount of properly delivered energy and a low
load of excess energy will be preferred. The sizing of
storage systems can also be performed, but the
algorithm for dimensioning the biomass heat-driven
combined heat and power plants as well as the
integration with the previous algorithms is still work
in progress.
The software developed with every part of the
methodology exists in a prototype version that
combines bash, python and a series of free and open
source software for geospatial applications. The aim
is to deliver a software tool that runs entirely on
python and the free and open source software for
geospatial applications, which should be available for
a wide range of users.
REFERENCES
Adhikari, R. S., Aste, N. and Manfren, M. (2012).
Optimization concepts in district energy design and
management – A case study. Energy Procedia, 14,
p.1386–1391. [Online]. Available at:
doi:10.1016/j.egypro.2011.12.1106 [Accessed: 19
February 2013].
Ai, B., Yang, H., Shen, H. and Liao, X. (2003). Computer-
aided design of PV/wind hybrid system. Renewable
Energy, 28 (10), p.1491–1512. [Online]. Available at:
doi:10.1016/S0960-1481(03)00011-9 [Accessed: 4
April 2013].
Angelis-Dimakis, A., Biberacher, M., Dominguez, J.,
Fiorese, G., Gadocha, S., Gnansounou, E., Guariso, G.,
Kartalidis, A., Panichelli, L., Pinedo, I. and Robba, M.
(2011). Methods and tools to evaluate the availability
of renewable energy sources. Renewable and
Sustainable Energy Reviews, 15 (2), p.1182–1200.
[Online]. Available at: doi:10.1016/j.rser.2010.09.049
[Accessed: 20 February 2013].
Asmus, P. (2010). Microgrids, Virtual Power Plants and
Our Distributed Energy Future. The Electricity Journal,
23 (10), p.72–82. [Online]. Available at:
doi:10.1016/j.tej.2010.11.001 [Accessed: 30 January
2013].
Biberacher, M., Gadocha, S., Gluhak, S., Dorfinger, N. and
Zocher, D. (2008a). Implementation of a GEOdatabase
to administrate global energy resources. In:
Proceedings of the International Congress on
Environmental Modelling and Software, Universitat
Politècnica de Catalunya, Barcelona, 2008. [Online].
Available at: http://www.iemss.org/iemss2008/uploads
/Main/S15-02-Biberacher_et_al-IEMSS2008.pdf
[Accessed: 8 March 2013].
Biberacher, M., Gadocha, S. and Zocher, D. (2008b). GIS
based Model to optimize possible self sustaining
regions in the context of a renewable energy supply. In:
Proceedings of the International Congress on
Environmental Modelling and Software, Universitat
Politècnica de Catalunya, Barcelona, 2008. [Online].
Available at: http://www.iemss.org/iemss2008/uploads
/Main/S15-12-Biberacher_1_et_al-IEMSS2008.pdf
[Accessed: 8 March 2013].
Borbely, A.-M. and Kreider, J. F. (2001). Distributed
generation: the power paradigm for the new
millennium, Mechanical engineering series. Boca
Raton: CRC Press.
Burgess, P. J., Rivas Casado, M., Gavu, J., Mead, A.,
Cockerill, T., Lord, R., van der Horst, D. and Howard,
D. C. (2012). A framework for reviewing the trade-offs
between, renewable energy, food, feed and wood
production at a local level. Renewable and Sustainable
Energy Reviews, 16 (1), p.129–142. [Online]. Available
at: doi:10.1016/j.rser.2011.07.142 [Accessed: 20
February 2013].
Calvert, K., Pearce, J. M. and Mabee, W. E. (2013). Toward
renewable energy geo-information infrastructures:
Applications of GIScience and remote sensing that
build institutional capacity. Renewable and Sustainable
Energy Reviews, 18, p.416–429. [Online]. Available at:
doi:10.1016/j.rser.2012.10.024 [Accessed: 19 February
2013].
Commission of the European communities. (2007).
Communication from the commission to the European
council and the European parliament: An Energy
Policy for Europe. [Online]. Available at: http://eur-
lex.europa.eu/LexUriServ/LexUriServ.do?uri=COM:2
007:0001:FIN:EN:PDF [Accessed: 2 April 2013].
European Commission (2011). Communication from the
commission to the European parliament, the council,
the European economic and social committee and the
committee of the regions: Energy Roadmap 2050.
[Online]. Available at: http://eur-
lex.europa.eu/LexUriServ/LexUriServ.do?uri=COM:2
011:0885:FIN:EN:PDF [Accessed: 1 April 2013].
Lambert, T., Gilman, P. and Lilienthal, P. (2006).
Micropower system modeling with HOMER.
Integration of alternative sources of energy, 1.
[Online]. Available at:
https://wwww.homerenergy.com/documents/Micropo
werSystemModelingWithHOMER.pdf [Accessed: 7
August 2013].
Lopes, J. A. P., Hatziargyriou, N., Mutale, J., Djapic, P. and
Jenkins, N. (2007). Integrating distributed generation
into electric power systems: A review of drivers,
challenges and opportunities. Electric Power Systems
Research, 77 (9), p.1189–1203. [Online]. Available at:
doi:10.1016/j.epsr.2006.08.016 [Accessed: 18
February 2013].
Spatio-temporalModelingforRenewableDistributedEnergyGenerationPlanningattheMunicipalScale
13