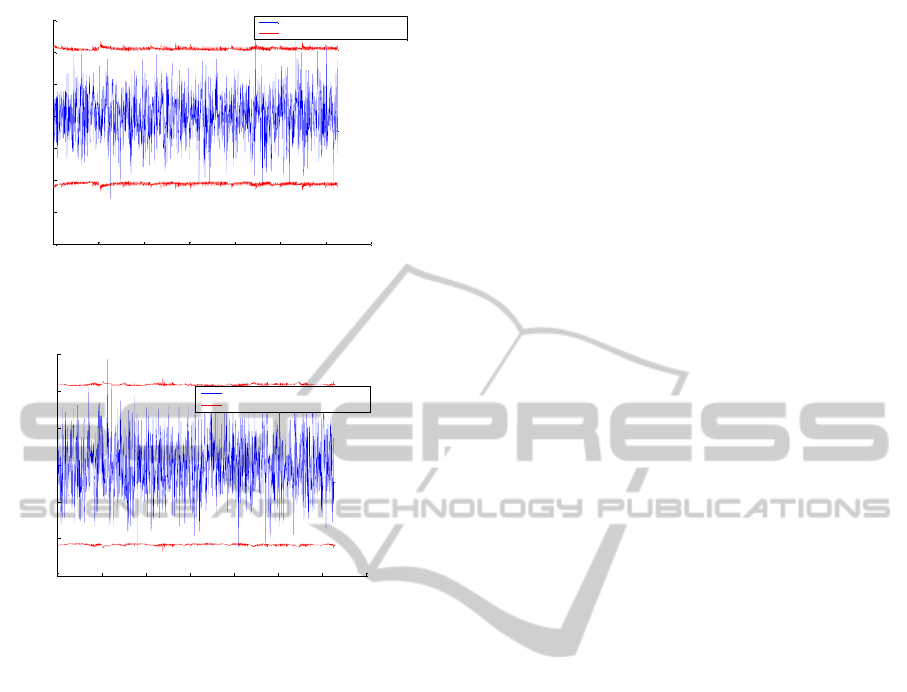
Figure 13: Standard deviation vs. distance innovation.
Figure 14: Standard deviation vs. angle innovation.
even though the loop is completed. Results show, as
long as the ambiguity of data association is reduced
by the UHS, a compact map configuration does not
affect the performance of the filter, and the results
would be identical to a SLAM case with a regular
loop closing case. This is important since filters such
as EKF fail to fulfill the navigation task in such
circumstances.
5 CONCLUSIONS
The main contribution of this study is a thorough
investigation and the validity check of Unscented
HybridSLAM. Simulations of the vehicle trajectory
and the estimation accuracy are investigated based
on proposed algorithm. The map accuracy and path
estimation are compared to currently used
algorithms, in particular, resulting in high quality of
the produced map and reducing error in estimated
position and robot heading. Results from different
scenarios indicate that the proposed algorithm is be
able to handle hundreds of nearby landmarks with
minimum data association ambiguity and a high
level of accuracy in the path estimation when
compared to currently used algorithms.
ACKNOWLEDGEMENTS
An academic version of a free software for robotics
research was used in this study. The software in
form of both MATLAB and C++ codes is available
at http://www.lasmea.univ-bpclermont.fr/ftp/pub/
trassou/SLAM/SLAM_Summer_School2002/SLAM
%20Summer%20School%202002.htm
REFERENCES
Smith R., Cheeseman P., 1986, The estimation and
representation of spatial uncertainty, International
Journal of Robotics Research, Vol. 5, Issue 4, pp. 56-
68.
Durrant-Whyte H., Bailey T., 2006, Simultaneous
localization and mapping (SLAM): part I the essential
algorithms, IEEE Robatics and Automation Magazine,
Vol. 13, No. 2, pp. 99-108, June.
Monjazeb A., Sasiadek J. Z., Necsulescu D., 2012, An
approach to autonomous navigation based on
Unscented HybridSLAM, Proc. of 17th International
Conference on Methods and Models in Automation
and Robotics, pp. 369-374, Miedzyzdroje, Poland.
Montemerlo M., Thrun S., 2003, Simultaneous
localization and mapping with unknown data
association using FastSLAM, Proceedings of the IEEE
International Conference on Robotics and Automation,
pp. 1985-1991, Vol. 2, September.
Bailey T., Mobile robot localization and mapping in
extensive outdoor environments, 2002, PhD Thesis,
Australian Centre for Field Robotics, University of
Sydney, Sydney, Australia.
Brooks A., Bailey T., 2009, HybridSLAM: Combining
FastSLAM and EKF-SLAM for reliable mapping,
Springer Tracts in Advanced Robotics, Volume 57,
pp. 647-661.
Williams S. B., Dissanayake G., Durrant-Whyte H., 2002,
An Efficient Approach to the Simultaneous
Localisation and Mapping Problem, Proceedings of
the IEEE International Conference on Robotics &
Automation, Washington DC.
Monjazeb A., Sasiadek J. Z., Necsulescu D., 2014,
Navigation of an Autonomous Mobile Robot Using
Data Association Method, Proc. of 11th International
Conference on Informatics in Control, Automation and
Robotics (ICINCO), pp. 304 - 311, Vienna, Austria.
Monjazeb A., Sasiadek J. Z., Necsulescu D., 2011,
Autonomous navigation among large number of
nearby landmarks using FastSLAM and EKF-SLAM; a
comparative study, Proc. of 16th International
Conference on Methods and Models in Automation
0 550 1100 1650 2200 2750 3300 3850
-0.4
-0.3
-0.2
-0.1
0
0.1
0.2
0.3
Distance Innovations
Time (s)
Deviation (m)
Innovations
Standard Deviation (95%)
0 550 1100 1650 2200 2750 3300 3850
-0.045
-0.030
-0.015
0
0.015
0.030
0.045
Angle Innovations
Time (s)
Angle Innovation (radians)
Inno vations
Standard Devication (95%)
HybridSLAM:ARobustAlgorithmforSimultaneousLocalizationandMapping
273