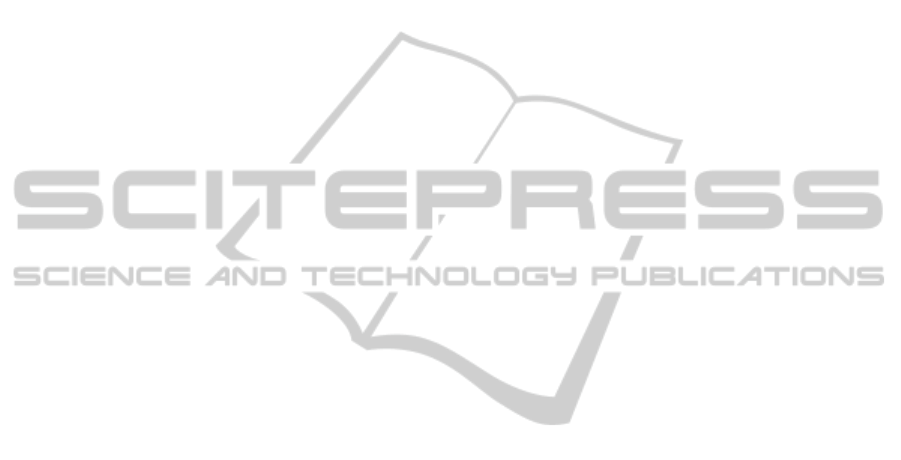
2012.Incorporating human behaviour in simulation
models of screening for breast cancer. European
Journal of Operational Research, 219(3), 491-507.
Brailsford, S.C., Hilton, N. A. 2001. A comparison of
discrete event simulation and system dynamics for
modeling healthcare systems. In: Riley J (ed).
Proceedings from ORAHS 2000. Glasgow: Scotland,
pp 18–39.
Chahal, K., Eldabi, T. 2008.Applicability of hybrid
simulation to different modes of governance in UK
healthcare. In Simulation Conference, 2008. Winter
pp. 1469-1477. IEEE.
Chen, Y. 2003. A system dynamicsbased study on
elderly non-acute service in Norway. In Proceedings
of the 21st International Conference of the System
Dynamics Society, System Dynamics Society, New
York.
Clark, D. N. 1999.Strategic Level MS/OR Tool Usage in
the United Kingdom and New Zealand: A
Comparative Survey. Asia-Pacific Journal of
Operational Research 16 (1): 35-51.
Cochran, K. J., Bharti, A. 2006.A Multi-stage Stochastic
Methodology for Whole Hospital Bed Planning Under
Peak Loading. International Journal of Industrial and
Systems Engineering (1 (1/2)), 8-35.
Denton, B.T., Miller, A.J., Balasubramanian, H.J.,
Huschka, T.R. 2010. Optimal Allocation of Surgery
Blocks to Operating Rooms Under Uncertainty.
Operations Research, 58(4), 802—816.
Diaz, R., Behr, J. G., Tulpule, M. 2012.A system
dynamics model for simulating ambulatory health care
demands. Simulation in Healthcare, 7(4), 243-250.
Dong, Y., Chbat, N. W., Gupta, A., Hadzikadic, M., Gajic,
O. 2012.Systems modeling and simulation
applications for critical care medicine. Annals of
intensive care, 2(1), 1-10.
Faezipour, M., Ferreira, S. 2011. Applying systems
thinking to assess sustainability in healthcare system
of systems. International Journal of System of Systems
Engineering, 2(4), 290-308.
Fialho, A. S., Oliveira, M. D., Sá, A. B. 2011.Using
discrete event simulation to compare the performance
of family health unit and primary health care centre
organizational models in Portugal. BMC health
services research,11(1), 274.
Fildes R., Ranyard, J.C. 1997.Success and Survival of
Operational Research Groups – A Review. Journal of
the Operational Research Society 48 (4): 336-360.
Fone, D., Hollinghurst, S., Temple, M., Round, A., Lester,
N., Weightman, A., et al. 2003. Systematic review of
the use and value of computer simulation modelling in
population health and health care delivery. J Public
Health, 25(4):325-335.
Forsberg, H. H., Aronsson, H., Keller, C., Lindblad, S.
2011. Managing health care decisions and
improvement through simulation modeling. Quality
Management in Healthcare, 20(1), 15-29.
Geranmayeh, S., Iyer, A. S. 2008. Capacity Analysis of
Critical Hospital Resources Using System Dynamics
Approach. Unpublished Manuscript.
Griffin, J., Xia, S., Peng, S., Keskinocak, P.
2012.Improving patient flow in an obstetric unit.
Health care management science, 15(1), 1-14.
Guizzardi, G., Wagner, G. 2012.Tutorial: Conceptual
simulation modeling with Onto-UML. In Simulation
Conference (WSC), Proceedings of the 2012 Winter
(pp. 1-15). IEEE.
Günal, M. M., Pidd, M. 2010.Discrete event simulation for
performance modelling in health care: a review of the
literature. Journal of Simulation, 4(1), 42-51.
Hafner, T., Shiffman, J. 2013.The emergence of global
attention to health systems strengthening. Health
policy and planning, 28(1), 41-50.
Hilton, N. A. 2001.Exploration into the behavior of
cardiac waiting lists (Doctoral dissertation, University
of Southampton).
Hirsch, G., Homer, J., Milstein, B., Scherrer, L., Ingersoll,
C., Landy, L., Fisher, E. 2012.ReThink Health
Dynamics: Understanding and influencing local health
system change. In 30th International Conference of
the System Dynamics Society, St. Gallen, Switzerland.
Homer, J., Jones, A., Seville, D., Essien, J., Milstein, B.,
Murphy, D. 2004.The CDC's diabetes systems
modeling project: Developing a new tool for chronic
disease prevention and control. In 22nd International
Conference of the System Dynamics Society, pp. 25-
29.
Hosking, M., Roberts, S., Uzsoy, R., Joseph, T. M.
2013.Investigating interventions for increasing
colorectal cancer screening: Insights from a simulation
model. Socio-Economic Planning Sciences, 47(2),
142-155.
Hsiao, C. T., Huang, H. H. 2012.A causal model for the
development of long-term facilities: a case in Taiwan.
Quality & Quantity, 46(2), 471-481.
Jahangirian, M., Eldabi, T., Naseer, A., Stergioulas, L. K.,
Young, T. 2010.Simulation in manufacturing and
business: A review. European Journal of Operational
Research, 203(1), 1-13.
Jeffrey, P., Seaton, R. 1995.The Use of Operational
Research Tools: A Survey of Operational Research
Practitioners in the UK. Journal of the Operational
Research Society 46 (7): 797-808.
Jun J.B., Jacobson S.H., Swisher J.R. 1999.Application of
discrete-event simulation in health care clinics: A
survey. J Oper Res Soc, 50:109-123.
Kadri F., Chaabane S., Tahon C. 2014. Simulation-based
decision support system to pre-vent and predict strain
situations in emergency department systems.
Simulation Modelling Practice and Theory, 42, 32–52.
Kuhl, M. E. 2012.A simulation study of patient flow for
day of surgery admission. In Simulation Conference
(WSC) Proceedings of the 2012 Winter (pp. 1-7).
IEEE.
Lane, D. C., Monefeldt, C., Rosenhead, J. V.
2000.Looking in the wrong place for healthcare
improvements: A system dynamics study of an
accident and emergency department. Journal of the
operational Research Society, 518-531.
Lattimer, V., Brailsford, S., Turnbull, J., Tarnaras, P.,
AReviewonDiscrete-eventSimulationandSystemDynamicsStudiesforHealthcareProblems
301