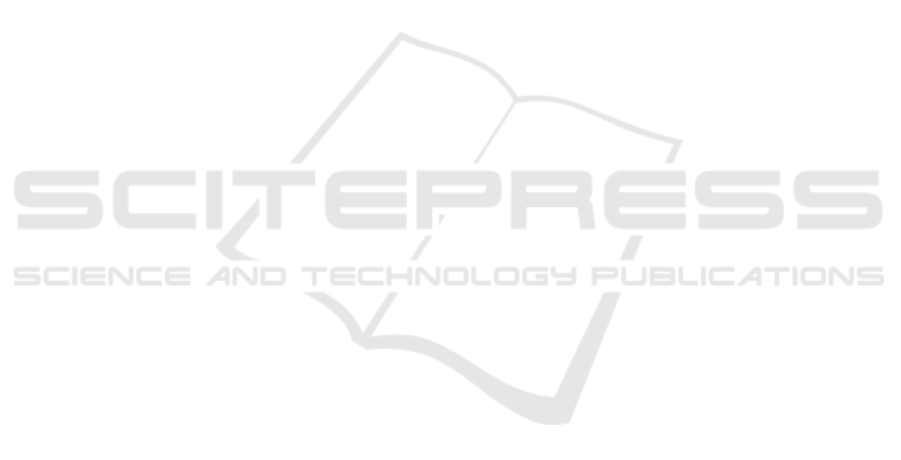
integration based on shoe-mounted sensors achieved
excellent results in distance estimation (Loose and
Orlowski, 2015b; Loose and Orlowski, 2015a).
Singleton et al. (Singleton et al., 1992) published a
study where they have shown that the prediction of
step time from step length and average velocity is
not a good choice for asymmetric gait patterns. In
contrast to the step length / duration, the stride length
and stride duration used in our algorithm are largely
the same for both legs. Following the effect described
by Singleton has no impact on our algorithm. Based
on the achieved results in the present validity study
using the average velocity and the stride duration for
the determination of stride length, a valid method
is found. Furthermore, the use of average velocity
could affect negatively if the walking velocity is not
constant during the walking sequence. Regarding
further investigations in the clinical environment with
patients having different asymmetries, the problem
depicted by Singleton et al. (Singleton et al., 1992)
should be kept in mind and a valid step length
algorithm should be used in order to examine the
asymmetric gait pattern of the patients.
5 CONCLUSION
At the end of these investigations we can conclude
that the gait parameters determined by the inertial-
sensor based gait analysis system InvestiGAIT show
partly excellent agreement with those parameters de-
termined by the reference system. Based on the re-
sults of the Wilcoxon test the agreement of four pa-
rameters have be presented. Furthermore, other pa-
rameters show a good agreement between the systems
in terms of a strong correlation. Small MOD, SEM
and LoA show similar effects. Consequently, the gait
parameter determined by InvestiGAIT are assumed as
valid and the system can be used in follow-up exami-
nations.
Nevertheless, further investigation are planned with
an adapted attachment of sensors on the subjects’
shoe in order to get a higher conformity of the gait
parameters. Furthermore, these measurements should
be conducted with a group of subjects without any
gait limitations in order to exclude the impact of the
disease. In this connection another method for calcu-
lating stride length proposed by (Mercer and Chona,
2015) should be investigated regarding validity in re-
lation to the reference system. They use the stride
length as the ratio of velocity and stride frequency
which is the number of foot contacts per second mea-
sured in Hertz (Magness, 2010).
ACKNOWLEDGEMENT
The authors would like to thank all the participants
taking part in that preliminary study.
REFERENCES
Atkinson, G. and Nevill, A. M. (1998). Statistical methods
for assessing measurement error (reliability) in vari-
ables relevant to sports medicine. Sports Medicine,
26(4):217–238.
Azad, P. (2009). Visual Perception for Manipulation and
Imitation in Humanoid Robots. Springer.
Bland, J. Martin; Altman, D. G. (1986). Statistical meth-
ods for assessing agreement between two methods of
clinical measurement. The Lancet, 8:307–309.
B
¨
ohme, B. (2012). Studie zur Untersuchung der Va-
lidit
¨
at des neuen Ganganalysesystems RehaWatch der
Firma Hasomed an gesunden Probanden. PhD thesis,
Friedrich-Schiller-Universit Jena.
Cloete, T. and Scheffer, C. (2008). Benchmarking of a full-
body inertial motion capture system for clinical gait
analysis. 30th Annual International IEEE EMBS Con-
ference.
Cohen, J. (1988). Statistical power analysis for the behav-
ioral sciences. (2nd ed.). Hillsdale, NJ: Erlbaum.
Cuesta-Vargas, A. I., Galan-Mercant, A., and Williams,
J. M. (2010). The use of inertial sensors system for
human motion analysis (systematic review). Physical
Therapy Reviews, 15(6):462–473.
Derlien, S., B
¨
ohme, B., and Smolenksi, U. (2010). Va-
lidit
¨
atsuntersuchung zum neuen, innovativen gang-
analysesystem rehawatch von hasomed. Manuelle
Medizin, 48(254-259).
Greene, B. R., McGrath, D., ONeill, R., ODonovan, K. J.,
Burns, A., and Caul?eld, B. (2010). An adaptive
gyroscope-based algorithm for temporal gait analysis.
Med Biol Eng Comput, 48:1251–1260.
Hundza, S. R., Hook, W. R., Harris, C. R., Mahajan, S. V.,
Leslie, P. A., Spani, C. A., Spalteholz, L. G., Birch,
B. J., Commandeur, D. T., and Livingston, N. J.
(2014). Accurate and reliable gait cycle detection in
parkinson’s disease. IEEE transactions on neural sys-
tems and rehabilitation engineering : a publication of
the IEEE Engineering in Medicine and Biology Soci-
ety, 22(1):127–137.
Latt, W. T., Veluvolu, K. C., and Ang, W. T. (2011).
Drift-free position estimation of periodic or quasi-
periodic motion using inertial sensors. Sensors
(Basel), 11(6):5931–5951.
Loose, H. and Orlowski, K. (2015a). Gait patterns in stan-
dard scenarios - using xsens mtw inertial measure-
ment units. 16th International Conference on Re-
search and Education in Mechatronics REM2015.
Loose, H. and Orlowski, K. (2015b). Model based determi-
nation of gait parameters using imu sensor data. Pro-
ceeding of Meachatronic Systems and Material (MSM
2015), 7-9 July 2015, Kaunas, Lithuania.
Evaluation of Gait Parameters Determined by InvestiGAIT against a Reference System
261