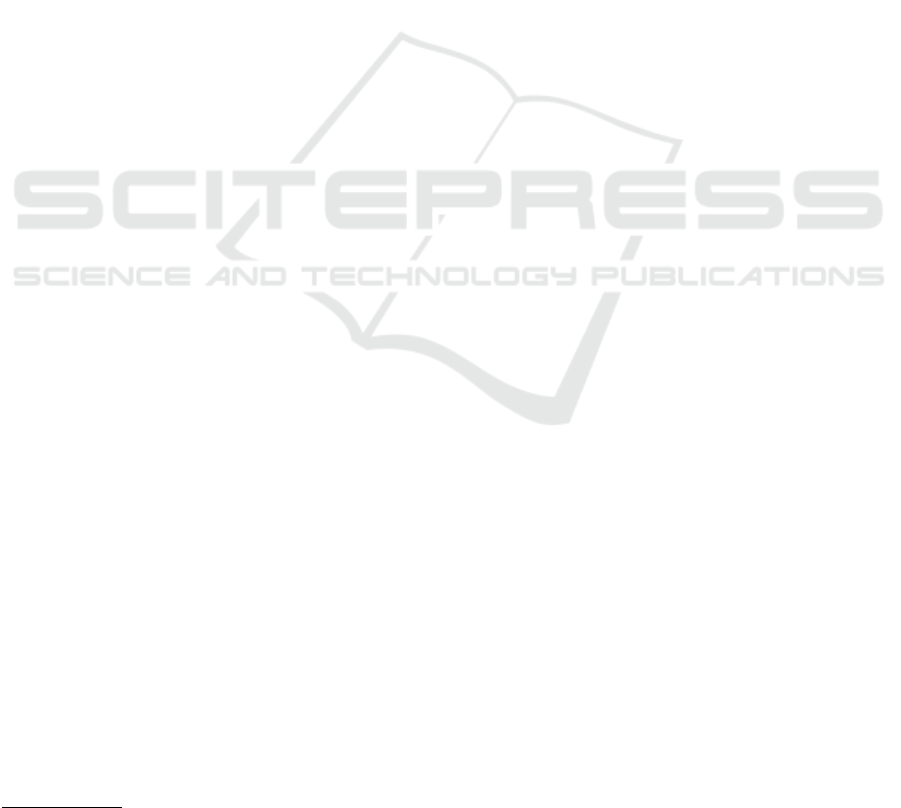
The spectral features were investigated with a
workload monitoring application, i.e. a BCI that is
able to discriminate between several levels of mental
effort. The system was trained using the N-back task,
a well-known task to induce workload by playing on
memory load and time pressure (see, e.g. (Mühl et al.,
2014)). The protocol we used was similar to (Mühl
et al., 2014), there were 360 trials presented during 6
blocks of alternate difficulty levels (0-back vs 2-back
conditions). The recording session lasted approxi-
mately 12 minutes.
The temporal features were investigated using an
oddball task directly implemented within OpenViBE
with a visual P300 speller. P300 spellers are well-
established BCI applications during which letters that
randomly flash on the screen can be used to spell
words with the sole brain activity. Indeed, when the
letter that the user wants to spell flashes, a particular
event-related potential (ERP) arises within the EEG,
which possess a positive “peak” around t=300 ms af-
ter the stimulus onset. This is commonly referred to
as the “oddball paradigm” since the occurrence of rare
stimuli is used to elicit brain responses. During the
recordings a matrix of 6 by 6 letters and digits was
displayed in full screen on a 24-inch display. Only the
calibration session occurred, during which one need
to focus one’s attention on a predefined sequence of
letters. 32 letters composing a pangram were men-
tally “spelled” this way. The sentence was, without
spaces, “pack my box with five dozen liquor jugs”.
Letters were flashing for 0.2s. There were 24 flashes
per letter (12 times the row, 12 times the column),
hence due to the matrix disposition there were in total
4608 trials, among which 768 were targets – “odd”
trials, i.e. the letters of the target sentence were flash-
ing. The recording session lasted approximately 30
minutes.
The acquisition of both amplifiers’ signals and
the P300 application occurred within the same Open-
ViBE scenario (script). The recordings of each am-
plifier were synchronized with the appropriate events
and exported in separate GDF files for later analyses.
There was also only one scenario involved in the syn-
chronization of all signals and events in the case of the
N-back task; stimulation from the python script sup-
porting this latter task were retrieved using the LSL
protocol, a network protocol dedicated to physiologi-
cal recordings which ensures accurate timings
4
.
2.2 Signal Processing
Two kinds of analyses were performed, using stan-
dard BCI signal processing pipelines. One aimed at
4
https://github.com/sccn/labstreaminglayer
assessing if and how the amplifiers differ in practice,
when used for classification. The second then looked
at the correlation between the acquired signals.
2.2.1 Classification
The signal processing of the data acquired during the
N-back task is analogous to (Mühl et al., 2014), i.e. 2s
time windows, 5 frequency bands – delta (1-3 Hz),
theta (4-6 Hz), alpha (7-13 Hz), beta (14-25 Hz) and
gamma (26-40 Hz) – and spatial filters. We used
common spatial patterns spatial filters to reduce the
16 channels to 6 “virtual” channels more discrimi-
nant between the workload conditions – see (Ramoser
et al., 2000). Additionally, we also tested a 3 fre-
quency bands version of our pipeline, that consider
only the lower frequencies, less prone to muscular ar-
tifacts – delta, theta and alpha.
Concerning the oddball task, we band-passed the
signal between 0.5Hz and 40Hz, downsampled it by a
factor 32 using the “decimate” Matlab function – by
a factor 8 for OpenBCI because of the reduced sam-
pling rate –, and applied regularized Eigen Fisher spa-
tial filters – a spatial filter specifically designed for
ERPs classification (Hoffmann et al., 2006) – to re-
duce channels’ dimension from 16 to 5. We used 1s
time windows after stimuli onsets – letters’ flashes –
to epoch (“slice”) our signal. However, in order to
prevent data to overlap between consecutive stimuli
due to the rapid pace of the flashes, after a first pass
of epoching we discarded overlapping time windows
from further analyses. This ensured that no part of the
signal could be seen twice by the classifier between
the training phase and the testing phase and bias the
accuracy. The procedure was automatic, the first non-
overlapping epoch in order of appearance being kept.
As a result, in the end we obtained 48 target trials
and 240 distractor trials for classification, identical
between the g.USBamp and the OpenBCI recordings.
Both for the workload and the P300 speller tasks,
we used shrinkage LDA (linear discriminant analysis)
for classification (Ledoit and Wolf, 2004). To assess
the classifiers’ performance on the calibration data,
we used 4-fold cross-validation. I.e. we split the col-
lected data into 4 parts of equal size, used 3 parts to
calibrate the classifiers and tested the resulting clas-
sifiers on the unseen data from the remaining part.
This process occurred 3 more times so that in the end
each part was used once as test data. Finally, we aver-
aged the obtained classification accuracies. The accu-
racy was measured using the area under the receiver-
operating characteristic curve (AUROCC). The AU-
ROCC is a metric that is robust against unbalanced
classes, as it is the case with oddball tasks. A score
of “1” means a perfect classification, a score of “0.5”
Comparison of an Open-hardware Electroencephalography Amplifier with Medical Grade Device in Brain-computer Interface Applications
107