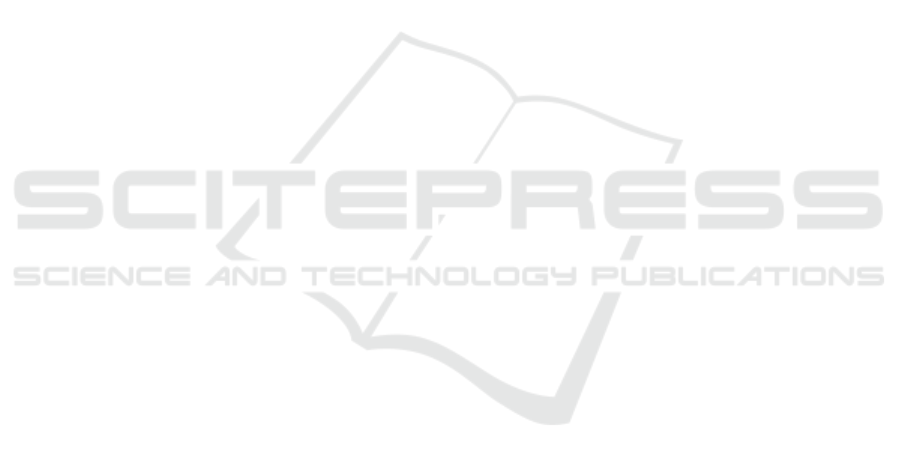
provement of the characterization phase based on the
RPs and the evaluation of other classification strate-
gies, that can take even more advantage of the new
features. It is clear also that the proposed method-
ology must be evaluated on large data sets as those
resources become available.
REFERENCES
Altschul, S. F., Madden, T. L., Sch
¨
affer, A. A., Zhang,
J., Zhang, Z., Miller, W., and Lipman, D. J. (1997).
Gapped blast and psi-blast: a new generation of pro-
tein database search programs. Nucleic acids re-
search, 25(17):3389–3402.
Baldi, P., Brunak, S., Chauvin, Y., Andersen, C. A., and
Nielsen, H. (2000). Assessing the accuracy of predic-
tion algorithms for classification: an overview. Bioin-
formatics, 16(5):412–424.
Baruah, A., Rani, P., and Biswas, P. (2015). Conforma-
tional entropy of intrinsically disordered proteins from
amino acid triads. Scientific reports, 5.
Berkholz, D. S., Krenesky, P. B., Davidson, J. R., and
Karplus, P. A. (2009). Protein Geometry Database:
a flexible engine to explore backbone conformations
and their relationships to covalent geometry. Nucleic
acids research, page gkp1013.
Bulashevska, A. and Eils, R. (2008). Using Bayesian multi-
nomial classifier to predict whether a given protein se-
quence is intrinsically disordered. Journal of theoret-
ical biology, 254(4):799–803.
Campen, A., Williams, R. M., Brown, C. J., Meng, J.,
Uversky, V. N., and Dunker, A. K. (2008). TOP-
IDP-scale: a new amino acid scale measuring propen-
sity for intrinsic disorder. Protein and peptide letters,
15(9):956.
Chou, K.-C. (2001). Prediction of protein cellular attributes
using pseudo-amino acid composition. Proteins:
Structure, Function, and Bioinformatics, 43(3):246–
255.
Das, R. K., Ruff, K. M., and Pappu, R. V. (2015). Relating
sequence encoded information to form and function
of intrinsically disordered proteins. Current opinion
in structural biology, 32:102–112.
DeForte, S. and Uversky, V. N. (2016). Order, disorder, and
everything in between. Molecules, 21(8):1090.
Deng, X., Eickholt, J., and Cheng, J. (2012). A compre-
hensive overview of computational protein disorder
prediction methods. Molecular BioSystems, 8(1):114–
121.
Dosztnyi, Z., Csizmok, V., Tompa, P., and Simon, I. (2005).
IUPred: web server for the prediction of intrinsically
unstructured regions of proteins based on estimated
energy content. Bioinformatics, 21(16):3433–3434.
Dunker, A. K., Oldfield, C. J., Meng, J., Romero, P., Yang,
J. Y., Chen, J. W., Vacic, V., Obradovic, Z., and Uver-
sky, V. N. (2008). The unfoldomics decade: an update
on intrinsically disordered proteins. BMC genomics,
9(Suppl 2):S1.
Faraggi, E., Yang, Y., Zhang, S., and Zhou, Y. (2009). Pre-
dicting continuous local structure and the effect of its
substitution for secondary structure in fragment-free
protein structure prediction. Structure, 17(11):1515–
1527.
Faraggi, E., Zhang, T., Yang, Y., Kurgan, L., and Zhou, Y.
(2012). SPINE X: improving protein secondary struc-
ture prediction by multistep learning coupled with
prediction of solvent accessible surface area and back-
bone torsion angles. Journal of computational chem-
istry, 33(3):259–267.
He, B., Wang, K., Liu, Y., Xue, B., Uversky, V. N., and
Dunker, A. K. (2009). Predicting intrinsic disorder in
proteins: an overview. Cell research, 19(8):929–949.
Hollingsworth, S. A. and Karplus, P. A. (2010). A fresh look
at the Ramachandran plot and the occurrence of stan-
dard structures in proteins. Biomolecular concepts,
1(3-4):271–283.
Huang, F., Oldfield, C. J., Xue, B., Hsu, W.-L., Meng,
J., Liu, X., Shen, L., Romero, P., Uversky, V. N.,
and Dunker, A. K. (2014). Improving protein order-
disorder classification using charge-hydropathy plots.
BMC bioinformatics, 15(Suppl 17):S4.
Jones, D. T. and Cozzetto, D. (2014). DISOPRED3: precise
disordered region predictions with annotated protein-
binding activity. Bioinformatics, page btu744.
Kawashima, S. and Kanehisa, M. (2000). AAindex:
amino acid index database. Nucleic acids research,
28(1):374–374.
Lafferty, J., McCallum, A., and Pereira, F. (2001). Con-
ditional random fields: Probabilistic models for seg-
menting and labeling sequence data. In Proceedings of
the 18h International Conference on Machine Learn-
ing - ICML 2001, pages 282–289.
Lieutaud, P., Canard, B., and Longhi, S. (2008). MeDor: a
metaserver for predicting protein disorder. BMC ge-
nomics, 9(Suppl 2):S25.
McGuffin, L. J., Bryson, K., and Jones, D. T. (2000). The
PSIPRED protein structure prediction server. Bioin-
formatics, 16(4):404–405.
Oates, M. E., Romero, P., Ishida, T., Ghalwash, M.,
Mizianty, M. J., Xue, B., Dosztnyi, Z., Uversky, V. N.,
Obradovic, Z., Kurgan, L., and others (2013). D2p2:
database of disordered protein predictions. Nucleic
acids research, 41(D1):D508–D516.
Peng, Z., Yan, J., Fan, X., Mizianty, M. J., Xue, B., Wang,
K., Hu, G., Uversky, V. N., and Kurgan, L. (2015).
Exceptionally abundant exceptions: comprehensive
characterization of intrinsic disorder in all domains of
life. Cellular and Molecular Life Sciences, 72(1):137–
151.
Potenza, E., Di Domenico, T., Walsh, I., and Tosatto, S. C.
(2015). MobiDB 2.0: an improved database of intrin-
sically disordered and mobile proteins. Nucleic acids
research, 43(D1):D315–D320.
Sickmeier, M., Hamilton, J. A., LeGall, T., Vacic, V.,
Cortese, M. S., Tantos, A., Szabo, B., Tompa, P.,
Chen, J., Uversky, V. N., and others (2007). DisProt:
the database of disordered proteins. Nucleic acids re-
search, 35(suppl 1):D786–D793.
BIOINFORMATICS 2017 - 8th International Conference on Bioinformatics Models, Methods and Algorithms
50