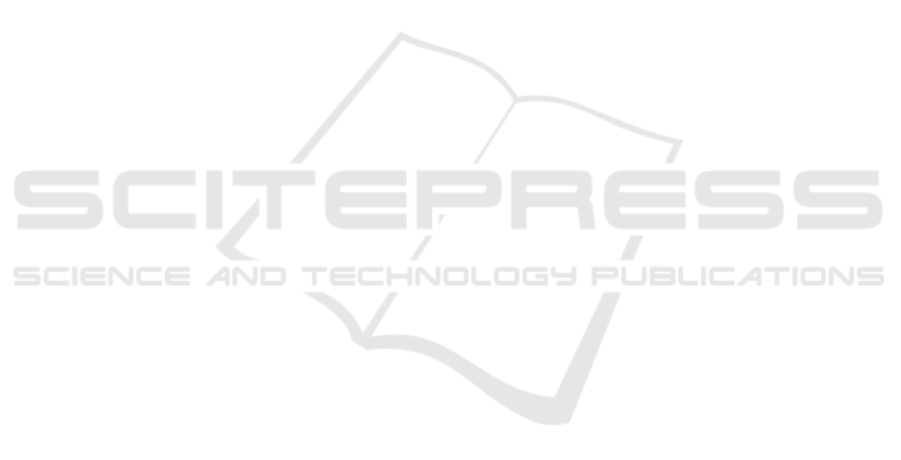
proof reading of the pre-final version of this article.
Last, I thank Gert-Jan de Vries for his invitation to
present this article at his special session.
REFERENCES
˚
Aborg, C. and Billing, A. (2003). Health effects of ‘the
paperless office’ – evaluations of the introduction of
electronic document handling systems. Behaviour &
Information Technology, 22(6):389–396.
Adams, R. A., Huys, Q. J. M., and Roiser, J. P. (2016).
Computational psychiatry: towards a mathematically
informed understanding of mental illness. Journal of
Neurology, Neurosurgery & Psychiatry, 87(1):53–63.
Bartol, T. (2016). Recreating healthcare: The next genera-
tion. The Nurse Practitioner, 41(11):10–11.
Beun, R. J. (2013). Persuasive strategies in mobile in-
somnia therapy: alignment, adaptation, and motiva-
tional support. Personal and Ubiquitous Computing,
17(6):1187–1195.
Blobel, B., Lopez, D. M., and Gonzalez, C. (2016). Patient
privacy and security concerns on big data for person-
alized medicine. Health and Technology, 6(1):75–81.
Cacioppo, J. T. and Hawkley, L. C. (2003). Social isolation
and health, with an emphasis on underlying mecha-
nisms. Perspectives in Biology and Medicine, 46(3
Supplement):S39–S52.
Carver, C. S. and Connor-Smith, J. (2010). Personality and
coping. Annual Review of Psychology, 61:679–704.
Chen, Y.-R. R. and Schulz, P. J. (2016). The effect of
information communication technology interventions
on reducing social isolation in the elderly: A system-
atic review. Journal of Medical Internet Research,
18(1):e18.
de Jong, E., Visscher, T. L. S., HiraSing, R. A., Heymans,
M. W., Seidell, J. C., and Renders, C. M. (2013).
Association between TV viewing, computer use and
overweight, determinants and competing activities of
screen time in 4- to 13-year-old children. International
Journal of Obesity, 37(1):47–53.
Dietz, W. H., Douglas, C. E., and Brownson, R. C.
(2016). Chronic disease prevention: Tobacco avoid-
ance, physical activity, and nutrition for a healthy
start. Journal of the American Medical Association
(JAMA), 316(16):1645–1646.
Donnelly, E. and Kuss, D. J. (2016). Depression among
users of social networking sites (SNSs): The role of
SNS addiction and increased usage. Journal of Addic-
tion and Preventive Medicine, 1(2):#107.
Fang, R., Pouyanfar, S., Yang, Y., Chen, S.-C., and Iyengar,
S. S. (2016). Computational health informatics in the
big data age: A survey. ACM Computing Surveys
(CSUR), 49(1):Article No. 12.
Ferlie, E., Montgomery, K., and Pedersen, A. R. (2016).
The Oxford Handbook of health care management.
Oxford, UK: Oxford University Press, 1st edition.
Fine, G. A. and Elsbach, K. D. (2000). Ethnography and ex-
periment in social psychological theory building: Tac-
tics for integrating qualitative field data with quantita-
tive lab data. Journal of Experimental Social Psychol-
ogy, 36(1):51–76.
Follmer, E. (2016). What we do to fit in: personality, cop-
ing, and Person-Environment fit. PhD thesis, Graduate
College, The University of Iowa, Iowa City, IA, USA.
Georga, E. I., Protopappas, V. C., Bellos, C. V., and Fo-
tiadis, D. I. (2014). Wearable systems and mobile ap-
plications for diabetes disease management. Health
and Technology, 4(2):101–112.
Gowrisankaran, S. and Sheedy, J. E. (2015). Computer Vi-
sion Syndrome: A review. Work, 52(2):303–314.
Huys, Q. J. M., Maia, T. V., and Frank, M. J. (2016).
Computational psychiatry as a bridge from neuro-
science to clinical applications. Nature Neuroscience,
19(3):404–413.
Jarvis, C. (2016). Physical examination and health assess-
ment. St. Louis, MO, USA: Elsevier, Inc., 7th edition.
Kalisch, R., M
¨
uller, M. B., and T
¨
uscher, O. (2015). A con-
ceptual framework for the neurobiological study of re-
silience. Behavioral and Brain Sciences, 38:e92.
Kaplan, J. (2017). Artificial Intelligence: Think again.
Communications of the ACM, 60(1):36–38.
Kreuter, M., Farrell, D., Olevitch, L., and Brennan, L.
(2000/2012). Tailoring health messages: Customiz-
ing communication with computer technology. LEA’s
Communication Series. Mahwah, NJ, USA: Rout-
ledge, Taylor & Francis Group / Lawrence Erlbaum
Associates, Inc., reprint edition.
Kulikowski, C. A., Shortliffe, E. H., Currie, L. M., Elkin,
P. L., Hunter, L. E., Johnson, T. R., Kalet, I. J., Lenert,
L. A., Musen, M. A., Ozbolt, J. G., Smith, J. W.,
Tarczy-Hornoch, P. Z., and Williamson, J. J. (2012).
AMIA Board white paper: definition of biomedi-
cal informatics and specification of core competen-
cies for graduate education in the discipline. Jour-
nal of the American Medical Informatics Association,
19(6):931–938.
Kun, L. (2016). Introductory remarks to the special issue on
social implications of technology, in honor of the late
dr. Lodewijk Bos. Health and Technology, 6(1):1–4.
Liu, D. and Baumeister, R. F. (2016). Social networking
online and personality of self-worth: A meta-analysis.
Journal of Research in Personality, 64:79–89.
Lundberg, U. and Cooper, C. L. (2011). The science of
occupational health: Stress, psychobiology, and the
new world of work. Chichester, West Sussex, UK:
Wiley-Blackwell / John Wiley & Sons Ltd.
Mandl, K. D. and Kohane, I. S. (2016). Time for a patient-
driven health information economy? The New Eng-
land Journal of Medicine, 374(3):205–208.
McCusker, K. and Gunaydin, S. (2015). Research using
qualitative, quantitative or mixed methods and choice
based on the research. Perfusion, 30(7):537–542.
Minen, M. T., Torous, J., Raynowska, J., Piazza, A.,
Grudzen, C., Powers, S., Lipton, R., and Sevick,
M. A. (2016). Electronic behavioral interventions
for headache: A systematic review. The Journal of
Headache and Pain, 17(1):Article 51.