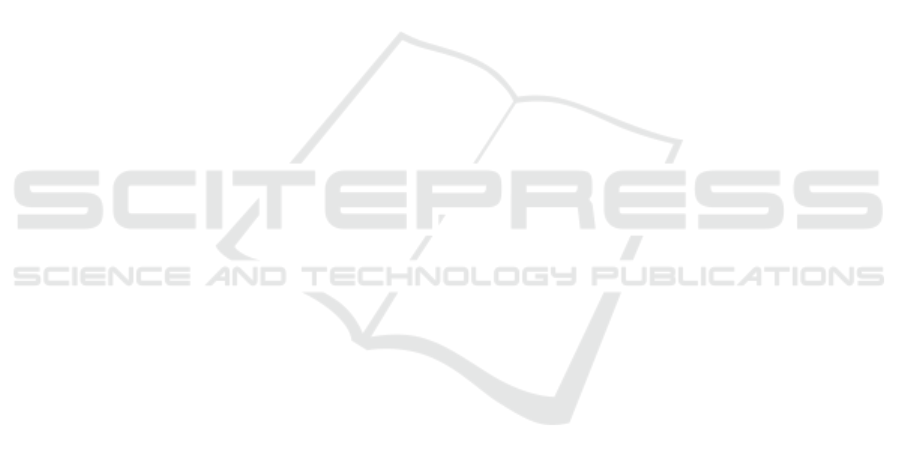
Bouchard D. and N. Badler, “Semantic Segmentation of
Motion Capture Using Laban Movement Analysis,” in
Proceedings of the 7th international conference on
Intelligent Virtual Agents, Berlin, Heidelberg, 2007,
pp. 37–44.
Carceroni, R.L. and K. N. Kutalakos, 2001. “Multi-view
scene capture by surfel sampling: from video streams to
non-rigid 3D motion, shape and reflectance,” in Eighth
IEEE International Conference on Computer Vision,
2001. ICCV 2001. Proceedings, 2001, vol. 2, pp. 60 –
67 vol.2.
Chen, Y.-L. and J. Chai, 2010. “3D Reconstruction of
Human Motion and Skeleton from Uncalibrated
Monocular Video,” in Computer Vision – ACCV 2009,
H. Zha, R. Taniguchi, and S. Maybank, Eds. Springer
Berlin Heidelberg, pp. 71–82.
Chi, D. M. Costa, L. Zhao, and N. Badler, “The EMOTE
model for effort and shape,” in Proceedings of the 27th
annual conference on Computer graphics and
interactive techniques, New York, NY, USA, 2000, pp.
173–182.
Cui, Y., S. Schuon, D. Chan, S. Thrun, and C. Theobalt,
2010. “3D shape scanning with a time-of-flight
camera,” in 2010 IEEE Conference on Computer
Vision and Pattern Recognition (CVPR), 2010, pp.
1173 –1180.
Davis, E., 2001. “BEYOND DANCE Laban's Legacy of
Movement Analysis,” Brechin Books, 2001
Dimitropoulos, K., P. Barmpoutis, A. Kitsikidis, and N.
Grammalidis, 2016. "Extracting dynamics from multi-
dimensional time-evolving data using a bag of higher-
order Linear Dynamical Systems", in Proc. 11th
International Conference on Computer Vision Theory
and Applications (VISAPP 2016), Rome, Italy.
Dobbyn, S., J. Hamill, K. O’Conor, and C. O’Sullivan,
2005. “Geopostors: a real-time geometry / impostor
crowd rendering system,” in Proceedings of the 2005
symposium on Interactive 3D graphics and games, New
York, NY, USA, 2005, pp. 95–102.
Furukawa, Y. and J. Ponce, 2010. “Dense 3D Motion
Capture from Synchronized Video Streams,” in Image
and Geometry Processing for 3-D Cinematography, R.
Ronfard and G. Taubin, Eds. Springer Berlin
Heidelberg, 2010, pp. 193–211.
Gall, J, C. Stoll, E. de Aguiar, C. Theobalt, B. Rosenhahn,
and H.-P. Seidel, 2009. “Motion capture using joint
skeleton tracking and surface estimation,” in IEEE
Conference on Computer Vision and Pattern
Recognition, CVPR 2009, 2009, pp. 1746 –1753.
Horn B. K. P. and B. G. Schunck, 1981. “Determining
optical flow,” Artificial Intelligence, vol. 17, no. 1–3,
pp. 185–203.
Izadi, S., D. Kim, O. Hilliges, D. Molyneaux, R.
Newcombe, P. Kohli, J. Shotton, S. Hodges, D.
Freeman, A. Davison, and A. Fitzgibbon, 2011.
“KinectFusion: real-time 3D reconstruction and
interaction using a moving depth camera,” in
Proceedings of the 24th annual ACM symposium on
User interface software and technology, New York,
NY, USA, 2011, pp. 559–568.
Kahol, K., P. Tripathi, and S. Panchanathan, “Automated
gesture segmentation from dance sequences,” in Sixth
IEEE International Conference on Automatic Face and
Gesture Recognition, 2004. Proceedings, 2004, pp. 883
– 888.
Kavan, L., S. Dobbyn, S. Collins, J. \vZára, and C.
O’Sullivan, 2008. “Polypostors: 2D polygonal
impostors for 3D crowds,” in Proceedings of the 2008
symposium on Interactive 3D graphics and games, New
York, NY, USA, pp. 149–155.
Kyriakaki, G., Doulamis, A., Doulamis, N., Ioannides, M.,
Makantasis, K., Protopapadakis, E., Hadjiprocopis, A.,
Wenzel, K., Fritsch, D., Klein, M., Weinlinger, G.,
2014. "4D Reconstruction of Tangible Cultural
Heritage Objects from web-retrieved Images,"
International Journal of Heritage in Digital Era, vol. 3,
no. 2, pp. 431-452.
Laumond, and D. Thalmann, 2006. “Real-time navigating
crowds: scalable simulation and rendering,” Computer
Animation and Virtual Worlds, vol. 17, no. 3–4, pp.
445–455, 2006.
Li, R., T. Luo, and H. Zha, 2010. “3D Digitization and Its
Applications in Cultural Heritage,” in Digital Heritage,
M. Ioannides, D. Fellner, A. Georgopoulos, and D. G.
Hadjimitsis, Eds. Springer Berlin Heidelberg, pp. 381–
388.
Li, R. and S. Sclaroff, 2008. “Multi-scale 3D scene flow
from binocular stereo sequences,” Computer Vision
and Image Understanding, vol. 110, no. 1, pp. 75–90,
2008.
Linaza, M, Kieran Moran, Noel E. O’Connor, 2013.
"Traditional Sports and Games: A New Opportunity for
Personalized Access to Cultural Heritage", 6th
International Workshop on Personalized Access to
Cultural Heritage (PATCH 2013), Rome, Italy.
Matsuyama, T., X. Wu, T. Takai, and S. Nobuhara, 2004.
“Real-time 3D shape reconstruction, dynamic 3D mesh
deformation, and high fidelity visualization for 3D
video,” Computer Vision and Image Understanding,
vol. 96, no. 3, pp. 393–434.
Menier, C., E. Boyer, and B. Raffin, 2006. “3D Skeleton-
Based Body Pose Recovery,” in Proceedings of the
Third International Symposium on 3D Data Processing,
Visualization, and Transmission (3DPVT’06),
Washington, DC, USA, 2006, pp. 389–396.
Mitra, N.J, A. Nguyen, and L. Guibas, 2014. “Estimating
surface normal in noisy point cloud data,” International
Journal of Computational Geometry & Applications,
vol. 14, no. 04n05, pp. 261–276.
Moon, K. and V. Pavlovic, “3D Human Motion Tracking
Using Dynamic Probabilistic Latent Semantic
Analysis,” in Canadian Conference on Computer and
Robot Vision, 2008. CRV ’08, 2008, pp. 155 –162.
Pettré, P. de H. Ciechomski, J. Maïm, B. Yersin, J.-P.
Laumond, and D. Thalmann, 2006. “Real-time
navigating crowds: scalable simulation and rendering,”
Computer Animation and Virtual Worlds, vol. 17, no.
3–4, pp. 445–455.
Pforsich, J., 1977. “Handbook for Laban Movement
Analysis", New York: Janis Pforsich, 1977.