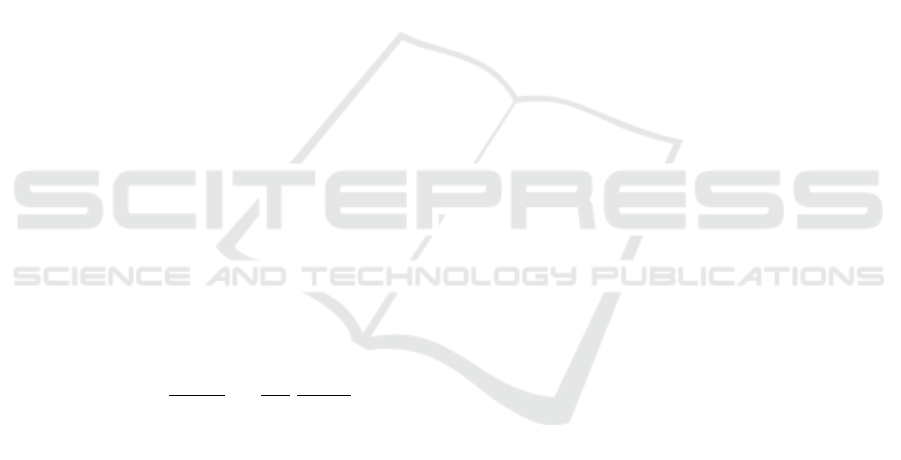
demic dynamics is therefore represented by the tran-
sitions S → I and I → R. In our implementation of
SIR model, the initial condition is a single infected
node (patient zero) in the network. At each step, an
infected individual is randomly selected and recov-
ers with probability p
SIR
. If this is not the case, one
of its susceptible neighbors is randomly chosen and
gets infected. The immunization takes place as a re-
action to the epidemic spreading when a given frac-
tion f (the epidemic threshold) of the total population
is infected. In the procedure, the fraction g of indi-
viduals to be vaccinated is selected according to some
score assigned to each node, which results in a prior-
ity list for the nodes to be vaccinated. The spreading
algorithm goes on as long as infected individuals are
present. Once the system reaches its final state, we
consider the final density d
R
of recovered individuals
as a measure of total number of individuals involved
in the epidemic. Clearly, an immunization strategy is
effective if it significantly reduces the density d
R
by
immunizing a relatively small fraction d
V
of the pop-
ulation. Since the evolution of the system is stochas-
tic, we perform a large number of simulations and the
effectiveness of a given strategy is measured in terms
of the averages hd
R
i and hd
V
i.
2.2 A New Immunization Strategy
Motivated by the issues discussed in the introduction,
we propose a local refinement of TI. Specifically, our
score takes into account both static knowledge of the
network geometry and dynamical information about
the epidemics, and it is given by
s
i
= d
i
+
∑
j∈N
i
β
δ
j,I
(d
j
)
1/2
+ γ
δ
j,S
d
i
d
i
− d
j
d
i
+ d
j
. (1)
Here, N
i
is the i-th node neighborhood, d
i
its con-
nectivity degree and δ
j,I
and δ
j,S
are Kronecker deltas
selecting respectively infected or susceptible nodes.
Finally, β and γ are tunable free parameters. Clearly,
the β-term favors the vaccination of nodes near the
epidemic front, where the factor (d
j
)
−1/2
is justified
by the fact that less connected neighbors constitute
bottlenecks for the epidemic spreading. The γ-term is
a further improvement based on the leverage central-
ity (Joyce et al., 2010).
2.3 Standard Immunization Strategies
In our numerical tests, we extensively compare our
proposal with the following standard immunization
strategies, suitably modified in the reactive setting:
• Targeted Immunization (TI). Originally de-
signed for scale-free networks, this scheme se-
lects nodes to be immunized among the suscep-
tible ones according to their degree.
• Acquaintance Immunization (AI). In this case,
random neighbors of randomly selected nodes are
vaccinated (if susceptible). This is a degree-based
vaccination strategy using only local information
about the network.
• High Risk Immunization (HR). Nodes to be im-
munized are chosen among the susceptible neigh-
bors of infected nodes.
3 COMPLEX NETWORKS
In this section, we briefly describe the networks we
considered as background for the epidemic spreading.
They range from the standard theoretical cases to a
collection of real world systems.
3.1 Theoretical Networks
In the first class, we consider the Barab
`
asi-Albert
(Albert and Barab
´
asi, 2002; Bornholdt and Schuster,
2006) and the Watts-Strogatz (Albert and Barab
´
asi,
2002; Bornholdt and Schuster, 2006; Watts and Stro-
gatz, 1998) models, which are respectively the proto-
types of scale-free and small-world graphs. We de-
note with BA[Q] the Barab
`
asi-Albert graph obtained
adding Q new links at each step of the growth algo-
rithm. On the other hand, we indicate as WS[Q] the
Watts-Strogatz graph obtained starting with a regu-
lar network with each node connected to 2Q adja-
cent sites. The rewiring probability in constructing
WS graphs is denoted by θ. In this paper, we con-
sider BA[2] and WS[2] networks with two different
rewiring probabilities θ = 0.1,0.5 for the WS case.
Since some real networks are believed to be approxi-
mately or locally scale-free, we also propose two vari-
ants of Barab
`
asi-Albert model. The first one is re-
alized starting with a BA graph and then randomly
rewiring R of its links. This variant can be thought as
a model that interpolates, depending on R , between
the pure scale-free networks and the completely ran-
dom graphs. We start with a BA[2] network and con-
sider R = 500, 1000,2000 rewiring events. The sec-
ond variant is realized starting with a network with
N nodes equally distributed in m BA centers. The
centers are then further randomly connected adding
new links between individuals in different clusters. In
this case, we start with m = 5,10,20 BA clusters with
N = 5000 total nodes and add k = 100,500,2000 new
edges.
Probing Complexity with Epidemics: A New Reactive Immunization Strategy
117