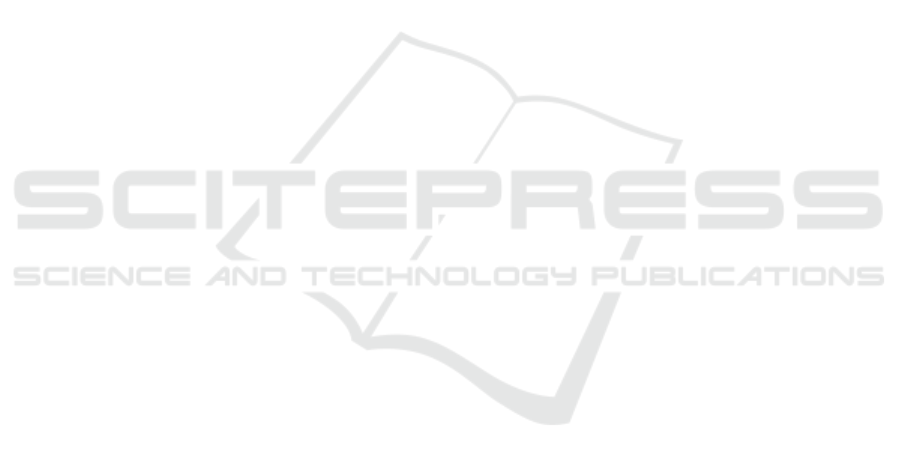
Rural extension and technical assistance have a
direct positive effect on income. Improvement of the
social indicator will tend to facilitate the access of
technical assistance creating, this way, a synergic
positive effect on income.
Environment in our study was measured in two
ways: non-degraded areas and the proportion of
forested areas. Keeping non-degraded areas relates
to technology and has a positive impact on
production. On the other hand, keeping a relative
large area of uncultivated land in the farm will have
a negative effect on income. Extension and technical
assistance may be the key factor to extract value
from forests and properly preserve these areas.
REFERENCES
Alves, E., Souza, G.S., 2015. Pequenos estabelecimentos
em termos de área também enriquecem? Pedras e
tropeços. Revista de Política Agrícola 24:7–21.
Alves, E., Souza, G.S., Marra, R., 2017, Uma viagem
pelas regiões e estados guiada pelo Censo
Agropecuário 2006. Revista de Política Agrícola
26:113–150.
Alves, E., Souza, G.S., Rocha, D.P., 2013. Desigualdade
nos campos sob a ótica do censo agropecuário 2006.
Revista de Política Agrícola 22:67–75.
Amsler, C., Prokhorov, A., Schmidt, P., 2016.
Endogeneity in stochastic frontier models. Journal of
Econometrics 190: 280–288.
Ferreira, C.R., Souza, S.C.I., 2007. As aposentadorias e
pensões e a concentração dos rendimentos
domiciliares per capita no Brasil e na sua área rural:
1981 a 2003. Revista de Economia e Sociologia Rural,
45(4):985–1011.
Helfand, S.M., Moreira, A.R.B., Bresnyan, Jr, E.E., 2015.
Agricultural Productivity and Family Farms in Brazil:
Creating opportunities and closing gaps. Available at
https://economics.ucr.edu/people/faculty/helfand/Helfa
nd%20Ag%20Productivity%20and%20Family%20Far
ms%20in%20Brazil%202015.pdf. Accessed 25 Oct
2017.
IBGE, 2012a. Censo Agropecuário 2006. Available at
http://www.ibge.gov.br/home/estatistica/economia/agr
opecuaria/censoagro/. Accessed 24 Jan 2012.
IBGE, 2012b. Censo Demográfico 2010.
http://censo2010.ibge.gov.br/. Acessed 24 Jan 2012.
INEP, 2012. Nota Técnica do Índice de Desenvolvimento
da Educação Básica. http://ideb.inep.gov.br/
resultado/. Accessed 24 Jan 2012.
Karakaplan, M.U., 2017. Fitting endogenous stochastic
frontier models in Stata. The Stata Journal 17(1):39–
55.
Karakaplan, M.U., Kutlu, L., 2013. Handling endogeneity
in stochastic frontier analysis. Available at
http://www.mukarakaplan.com/Karakaplan%20-
%20EndoSFA.pdf Accessed 10 March 2017.
Ministério da Saúde, 2011. IDSUS – Índice de
Desempenho do SUS. Ano 1.
http://portal.saude.gov.br/. Acessed 2 March 2012.
Neder, H.D., Silva, J.L.M., 2004. Pobreza e distribuição
de renda em áreas rurais: uma abordagem de
inferência. Revista de Economia e Sociologia Rural,
42(3):469-486.
Ney, M.G., Hoffmann, R., 2008. A contribuição das
atividades agrícolas e não-agrícolas para a
desigualdade de renda no Brasil rural. Economia
Aplicada, 12(3):365-393.
Ney, M.G., Hoffmann, R., 2009. Educação, concentração
fundiária e desigualdade de rendimentos no meio rural
brasileiro. Revista de Economia e Sociologia Rural,
47(1):147–181.
Souza, G.S., Gomes, E.G., Alves, E.R.A., 2017a.
Conditional FDH efficiency to assess performance
factors for Brazilian agriculture. Pesquisa Operacional
37:93–106.
Souza, G.S., Gomes, E.G., Alves, E.R.A., 2017b. Market
imperfections and income concentration: Global and
regional perspectives on Brazilian agricultural
production performance. Proceedings of the 21st
IFORS Triennial Conference. Quebec, 222–222.
Souza, G.S., Gomes, E.G., Alves, E.R.A., Magalhães, E.,
Rocha, D.P., 2013. Um modelo de produção para a
agricultura brasileira e a importância da pesquisa da
Embrapa. In: Alves, E.R.A., Souza, G.S., Gomes, E.G.
(eds.). Contribuição da Embrapa para o
desenvolvimento da agricultura no Brasil, p. 49–86.
ICORES 2018 - 7th International Conference on Operations Research and Enterprise Systems
20