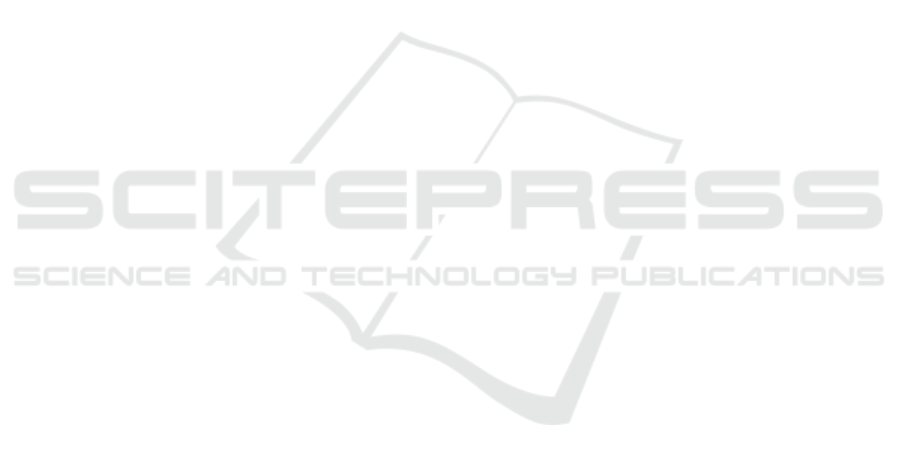
give immediate information on the device itself and
the second information source, which usually more
detailed is gathered on a website. With the first
information source (short look), usually creates
immediate awareness with the user on their
behavior. The barriers in this phase, not all users can
give meaning to their data, due because of lack of
time, not the ability to have a holistic view of the
collected data or technical limitations. Finally,
during the action phase users will use the personal
information to change behavior, to set goals or
adjust goals set during the previous process.
(Epstein D. , 2015) proposed a model for lived
informatics for personal informatics, comprising of
three stages, initially starting with the decision to
track and decide on the selection of tools to track.
Choosing or deciding to track oneself could be for
many reasons and include: to improve one’s health,
to improve one’s lifestyle or to find a new life
experience/activity (Choe, 2014). Deciding on the
selection of tools involves comparing devices or
mobile apps such as Runkeeper (running mobile
application) or Human (a physical and calorie
tracker) or decide to wear a wristband such as Fitbit
or Jawbone to name a few options.
Stage two relates to the ‘tracking and acting’
process which is ‘an ongoing process of collecting,
integrating and reflecting’ (Epstein, 2015), (Choe,
2014) notes three activities; ‘collecting, integrating
and reflecting’ which are distinct and dependent
upon data. Self-trackers learn about their behavior
during the process of collecting and monitoring the
data, “the main importance however, is to get
meaningful insights and reflect on data to make
positive change” (Choe, 2014, p. 10).
Stage three relates to the ‘lapsing stage’, which is
associated to individuals/users who choose to stop
self-tracking for a set amount of time or completely.
Based upon recent research the dropout rate is quite
high for several reasons, including: technology
failure, lack of interest, curiosity is gone, or the cost
of tracking in terms of time (Endeavour Partners,
2014), (Fritz, 2014), (Karapanos, 2015), (Shih,
2015) (Epstein, 2016).
Finally, there is ‘the resuming phase’, these can
be short breaks, where a self-tracker has gone on
holiday and forgotten to take their wearable device
or they choose to have a longer break. In the latter,
the self-tracker might start again by reflecting first
on the older data, and then decides later to start
tracking again and collecting more data depending
on the tracking activity (Epstein, 2015).
Both Li and Epstein looked at the usage of
Personal Informatics from a user perspective and the
different phases a user goes using these
technologies. Epstein added and refined the stage
model by adding ‘lapsing’ and ‘resuming’ to the
tracking. Considering the challenge of people not
managing to sustain self-tracking for long, we argue
that resuming has a specific importance for long-
term self-tracking. It helps the user to recollect
previous information of the system that has been
gathered before, to evaluate, to look for confirmation
that they achieved in certain goals they have set.
The user can pick up where they left off, set
different goals, sometimes higher goals, or
sometimes in a different way, in a different routine.
They can compare the past achievements with the
new information when picking up a certain activity
again and working towards their personal best result.
In addition, there is also the notion of the need of
tracking, long-term user, use the past gathered
information to motivate themselves again to start a
new. The need to track themselves to be able to
follow and evaluate their progress in a specific
program or activity they have setup.
Next we will look at the personal informatics
from a sociological lens where researchers have
proposed typologies to define Personal Informatics
users.
2.3 Types of Self-trackers
Taking a sociological perspective Lupton argues that
‘The practices, meanings, discourses and
technologies associated with self-tracking are
inherently and inevitably the product of a broader
social, cultural and political process’, (Lupton,
2016). Lupton underlines the sociological dimension
of self-tracking, distinguishing five types: Private,
Communal, Pushed, Imposed and Exploited. Here
we focus mainly on the private and communal
modes of self-tracking, as the users we interviewed
and surveyed are using the devices or tools by
choice. In a private mode, self-tracking is mainly a
private activity by one’s own choice, where at the
communal mode, one shares tracking results within
a community or others like family, friends and so
forth. The remaining modes are not by choice and
are a main concern in the whole movement of self-
tracking: pushed, imposed or exploited self-tracking.
It is known that the data we gather can also be used
by others, as a surveillance tool or for commercial
reasons (Lupton, 2014). Furthermore, pushed and
imposed modes, are increasingly a concern as
Personal Informatics enters the workplace, and the
insurance space, where it is used as an incentive to
stay healthy or to personalize insurances. These last
three modes are therefore important user design
aspects from a design ethics (Cummings, 2006)
perspective. In all of these modes, a Value Sensitive
Design (Cummings, 2006) should focus on
Exploring Quantified Self Attitudes
255