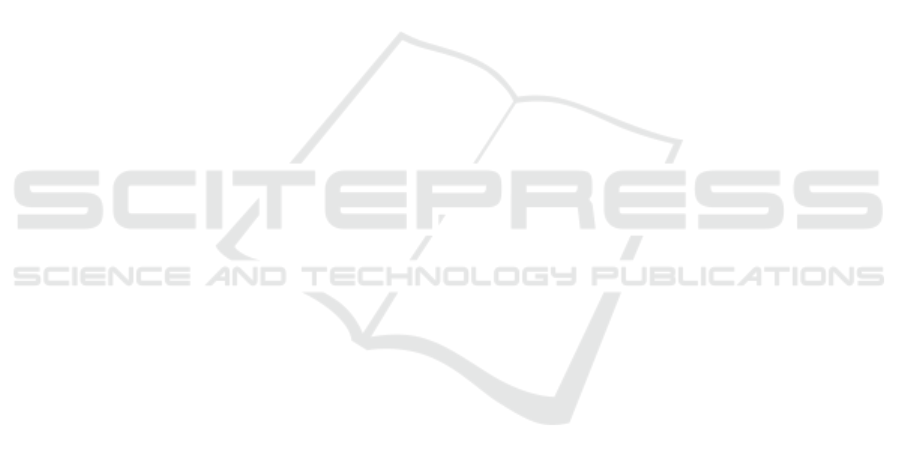
Geographically Weighted Regression. Appl. Geogr.,
31(1): 292-302.
Guan, Q. & Clarke, K. C. (2010). A general-purpose
parallel raster processing programming library test
application using a geographic cellular automata model.
Int J Geographical Inf. Sci, 24(5): 695-722.
Haase, D., Schwarz, N., Strohbach, M., Kroll, F. & Seppelt,
R. (2012). Synergies, Trade-offs, and Losses of
Ecosystem Services in Urban Regions: an Integrated
Multiscale Framework Applied to the Leipzig-Halle
Region, Germany. Ecology and Society, 17(3): 22.
Haregeweyn, N., Fikadu, G., Tsunekawa, A., Tsubo, M. &
Meshesha, D. T. (2012). The dynamics of urban
expansion and its impacts on land use/land cover
change and small-scale farmers living near the urban
fringe: A case study of Bahir Dar, Ethiopia. Landscape
Urban Plan., 106(2): 149-157.
Hietel, E., Waldhardt, R. & Otte, A. (2007). Statistical
modelling of land-cover changes based on key
socio-economic indicators. Ecol. Econ., 62: 496-507.
Hu, Z. & Lo, C. P. (2007). Modeling urban growth in
Atlanta using logistic regression. Comput. Environ.
Urban., 31(6): 667-688.
Jantz, C. A., Goetz, S. J., Donato, D. & Claggett, P. (2010).
Designing and implementing a regional urban
modeling system using the SLEUTH cellular urban
model. Comput. Environ. Urban., 34(1): 1-16.
Jat, M. K., Choudhary, M. & Saxena, A. (2017). Urban
growth assessment and prediction using RS, GIS and
SLEUTH model for a heterogeneous urban fringe. The
Egyptian Journal of Remote Sensing and Space Science.
http://dx.doi.org/10.1016/j.ejrs.2017.02.002.
Kantakumar, L. N., Kumar, S. & Schneider, K. (2016).
Spatiotemporal urban expansion in Pune metropolis,
India using remote sensing. Habitat Inter., 51: 11-22.
Kuang, W., Chi, W., Lu, D. & Dou, Y. (2014). A
comparative analysis of megacity expansions in China
and the U.S.: Patterns, rates and driving forces.
Landscape Urban Plan., 132: 121-135.
Li, C., Zhao, J. & Xu, Y. (2017). Examining
spatiotemporally varying effects of urban expansion
and the underlying driving factors. Sustainable Cities
and Society, 28: 307-320.
Li, X., Zhou, W. & Ouyang, Z. (2013). Forty years of urban
expansion in Beijing: What is the relative importance
of physical, socioeconomic, and neighborhood factors?
Appl. Geogr., 38: 1-10.
Lin, J., Huang, B., Chen, M. & Huang, Z. (2014). Modeling
urban vertical growth using cellular automata:
Guangzhou as a case study. Appl. Geogr., 53: 172-186.
Liu, R., Zhang, K., Zhang, Z. & Borthwick, A. G. (2014).
Land-use suitability analysis for urban development in
Beijing. J Environ Manage, 145: 170-179.
Long, Y., Gu, Y. & Han, H. (2012). Spatiotemporal
heterogeneity of urban planning implementation
effectiveness: Evidence from five urban master plans of
Beijing. Landscape Urban Plan., 108: 103-111.
Luo, J. & Wei, Y. H. D. (2009). Modeling spatial variations
of urban growth patterns in Chinese cities: The case of
Nanjing. Landscape Urban Plan., 91(2): 51-64.
Poelmans, L. & Rompacy, A. V. (2009). Detecting and
modellling spatial patterns of urban sprawl in highly
fragmented areas: a case study in the Flanders-Brussels
region. Landscape Urban Plan., 93(1): 10-19.
Poyil, R. P. & Misra, A. K. (2015). Urban agglomeration
impact analysis using remote sensing and GIS
techniques in Malegaon city, India. International
Journal of Sustainable Built Environment, 4(1):
136-144.
Qi, Y., Henderson, M., Xu, M., Chen, J., Shi, P., He, C. &
Skinner, W. (2004). Evolcing core-periphery
interactions in a rapidly expanding urban landscape: the
case of Beijing. Landscape Ecology, 19: 491-497.
Santé, I., García, A. M., Miranda, D. & Crecente, R. (2010).
Cellular automata models for the simulation of
real-world urban processes: A review and analysis.
Landscape Urban Plan., 96(2): 108-122.
Shan, J., Alkheder, S. & Wang, J. (2008). Genetic
algorithms for the calibration of cellular automata
urban growth modeling. Photogrammetric Engineering
and Remote Sensing, 74: 1267-1277.
Silva, E. A. & Clarke, K. C. (2002). Calibration of the
SLEUTH urban growth model for Lisbon and Porto,
Portugal. Comput. Environ. Urban., 26: 525-552.
Singh, P., Kikon, N. & Verma, P. (2017). Impact of land use
change and urbanization on urban heat island in
Lucknow city, Central India. A remote sensing based
estimate. Sustainable Cities and Society, 32: 100-114.
Su, S., Xiao, R. & Zhang, Y. (2012). Multi-scale analysis of
spatially varying relationships between agricultural
landscape patterns and urbanization using
geographically weighted regression. Appl. Geogr, 32(2):
360-375.
Thapa, R. B. & Murayama, Y. (2012). Scenario based urban
growth allocation in Kathmandu Valley, Nepal.
Landscape and Urban Planning, 105: 140-148.
Zhang, C., Tian, H., Chen, G., Chappelka, A., Xu, X., Ren,
W., Hui, D., Liu, M., Lu, C., Pan, S. & Lockaby, G.
(2012). Impacts of urbanization on carbon balance in
terrestrial ecosystems of the Southern United States.
Environ Pollut, 164: 89-101.
GISTAM 2018 - 4th International Conference on Geographical Information Systems Theory, Applications and Management
166