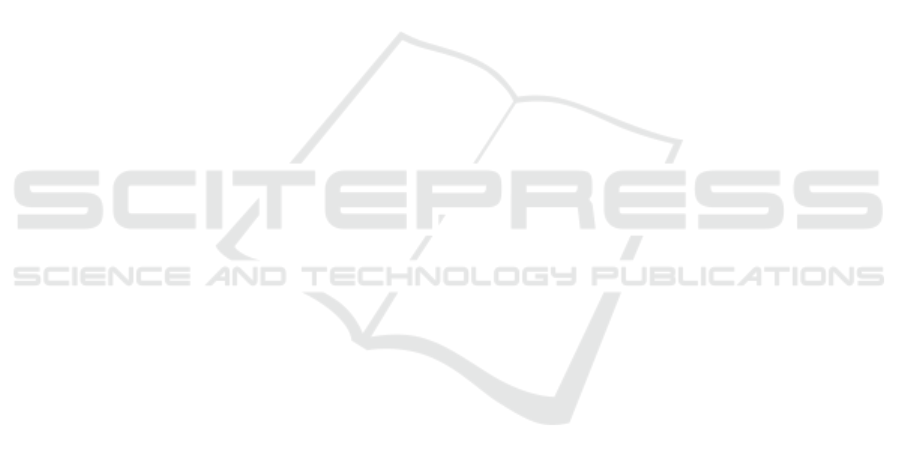
Kobsa, A., Koenemann, J. and Pohl, W. (2001),
“Personalised hypermedia presentation techniques for
improving online customer relationships”, The Know-
ledge Engineering Review, Vol. 16 No. 02, p. 111.
Koch, N. (2000), “Software Engineering for Adaptive
Hypermedia Systems. Reference Model, Modeling
Techniques and Development Process”, Dissertation,
Ludwig-Maximilians-Universität München, München,
20 October.
Krapp, A., Hidi, S. and Renninger, K.A. (1992), “Interest,
learning and development”, in Renninger, K.A., Hidi,
S. and Krapp, A. (Eds.), Role of interest in learning and
development, pp. 3–25.
Krömker, H., Hoffmann, M. and Huntemann, N. (2015),
Anwendungsorientierte Fachlandkarten: Fallbeispiel
Nanotechnologie., 10. Ingenieurpädagogische
Regionaltagung 2015 - Anwendungsorientierung und
Wissenschaftsorientierung in der Ingenieurbildung,
Eindhoven, Niederlande.
Krömker, H., Hoffmann, M. and Huntemann, N. (2017),
“Wissensstrukturierung für das Lernen in den
Ingenieurwissenschaften”, in Kammasch, G., Klaffke,
H. and Knutzen, S. (Eds.), Technische Bildung im
Spannungsfeld zwischen beruflicher und akademischer
Bildung: Die Vielfalt der Wege zu technischer Bildung
Referate der 11. Ingenieurpädagogischen
Regionaltagung 2016 an der Technischen Universität
Hamburg vom 23.-25. Juni 2016, IPW, Berlin, Berlin,
pp. 101–108.
Lyons, K. (2015), “What can a dumb watch teach a
smartwatch?”, in Mase, K., Langheinrich, M. and
Gatica-Perez, D. (Eds.), ISWC'15: Proceedings of the
2015 ACM International Symposium on Wearable
Computers September 7-11, 2015, Osaka, Japan,
Osaka, Japan, 7/9/2015 - 11/9/2015, Association for
Computing Machinery, New York, NY, pp. 3-10.
Min, C., Kang, S., Yoo, C., Cha, J., Choi, S., Oh, Y. and
Song, J. (2015), “Exploring current practices for battery
use and management of smartwatches”, in Mase, K.,
Langheinrich, M. and Gatica-Perez, D. (Eds.),
ISWC'15: Proceedings of the 2015 ACM International
Symposium on Wearable Computers September 7-11,
2015, Osaka, Japan, Osaka, Japan, 7/9/2015-
11/9/2015, Association for Computing Machinery, New
York, New York, pp. 11–18.
Mödritscher, F. (2007), “Implementation and Evaluation of
Pedagogical Strategies in Adaptive E-Learning
Environments”, Dissertation, Graz University of
Technology, Graz, 05.2007.
Niegemann, H.M., Domagk, S., Hessel, S., Hein, A.,
Hupfer, M. and Zobel, A. (2008), Kompendium
multimediales Lernen, X.media.press, Springer, Berlin,
New York.
Park, S., Chung, K. and Jayaraman, S. (2014), “Wearables”,
in Sazonov, E. (Ed.), Wearable Sensors, Academic
Press, pp. 1–23.
Rachbauer, T. (2009), Adaption von E-Learningsystemen -
Moodle im Vergleich zu anderen Open Source
Learning Content Management Systemen, Recht -
Wirtschaft - Steuern, Igel, Hamburg.
Rheinberg, F. (2010), “Intrinsische Motivation und Flow-
Erleben”, in Heckhausen, J. and Heckhausen, H. (Eds.),
Motivation und Handeln, Springer-Lehrbuch, 4.,
überarbeitete und erw. Aufl., Springer-Verlag, Berlin,
pp. 365–387.
Rheinberg, F. and Vollmeyer, R. (2012), Motivation,
Urban-Taschenbücher, Vol. 555, 8., aktual. Aufl.,
Kohlhammer, Stuttgart.
Rheinberg, F., Vollmeyer, R. and Burns, B. D. (2001),
“FAM. Ein Fragebogen zur Erfassung aktueller
Motivation in Lern- und Leistungssituationen”,
Diagnostica, Vol. 47 No. 2, pp. 57–66.
Rheinberg, F., Vollmeyer, R. and Rollett, W. (2000),
“Motivation and action in self-regulated learning”, in
Boekaerts, M., Pintrich, P.R. and Zeidner, M. (Eds.),
Handbook of Selfregulation, Academic Press, London,
pp. 503–529.
Schallberger, U. (2005), Kurzskalen zur Erfassung der
Positiven Aktivierung, Negativen Aktivierung und
Valenz in Experience Sampling Studien (PANAVA-
KS): Theoretische und methodische Grundlagen,
Konstruktvalidität und psychometrische Eigenschaften
bei der Beschreibung intra- und interindividueller
Unterschiede., Forschungsberichte aus dem Projekt
"Qualität des Erlebens in Arbeit und Freizeit" No. 6,
Zürich (accessed 27 Nov 2017).
Schiefele, U. (2009), “Situational and individual interest”,
in Wentzel, K.R. and Miele, D.B. (Eds.), Handbook of
Motivation at School, Educational Psychology
Handbook, Taylor & Francis, pp. 197–222.
Schiefele, U. and Wild, K.P. (1994), “Lernstrategien im
Studium Ergebnisse zur Faktorenstruktur und
Reliabilität eines neuen Fragebogens. Learning
strategies of university students: Factor structure and
reliability of a new questionnaire”, Zeitschrift für
Differentielle und Diagnostische Psychologie, Vol. 15
No. 4, pp. 185–200.
Schneider, O., Martens, T., Bauer, M., Ott-Kroner, A.,
Dick, U. and Dorochevsky, M. (2017), “SensoMot –
Sensorische Erfassung von Motivationsindikatoren zur
Steuerung adaptiver Lerninhalte”, in Igel, C., Ullrich,
C. and Wessner, M. (Eds.), Bildungsräume 2017,
Gesellschaft für Informatik, Bonn, pp. 267-272.
Sullivan, S. (2017), Designing for wearables: Effective UX
for current and future devices, First edition, O'Reilly
Media, Inc, Sebastopol, CA.
Trepte, S. and Reinecke, L. (2013), Medienpsychologie,
Kohlhammer-Urban-Taschenbücher, Vol. 726, 1. Aufl.,
Kohlhammer, Stuttgart.
Adaptive E-Learning Technologies for Sustained Learning Motivation in Engineering Science
425