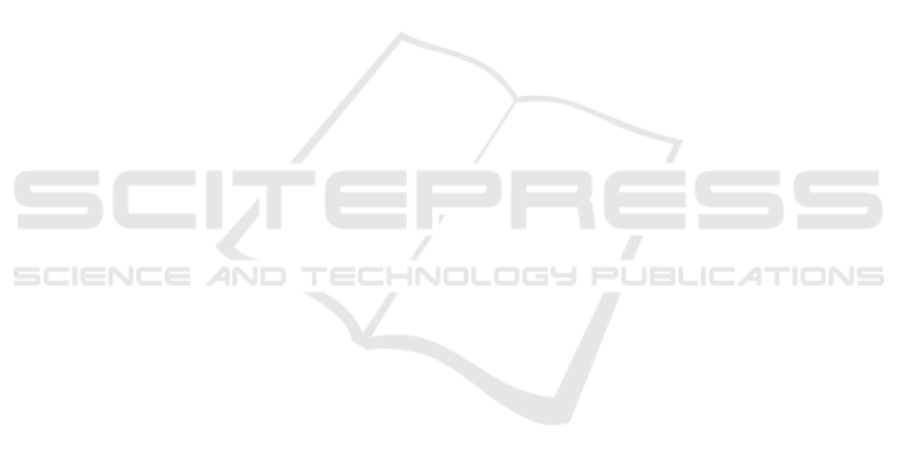
tion, where sharing, reusing and inferring new know-
ledge plays a central role.
In Section 2, some related works developed in
the field of higher education institutions are descri-
bed. In Section 3, the development of the proposal
is detailed indicating the methodology used (which
implies the specification of requirements for the on-
tology network), the reuse of resources, the reengi-
neering carried out to reuse the ontological resources
and the conceptualization of the ontological network
proposal based on the UPS datasets. Continuing, in
Section 4 we present the results of the experimenta-
tion, and Section 5 contains the conclusions of our
work.
2 RELATED WORK
There is a broad field of work related to knowledge
management in the academic domain using ontolo-
gies. Several authors have used them as a means to
represent different perspectives of the academic dom-
ain. For example, the authors of (Laoufi et al., 2011)
proposed an approach to establish an organizational
Memory of the University System (MUS). The MUS
is not only on pedagogical knowledge (topics, sub-
jects, courses, etc.) but also works with other know-
ledge used in the context of a university system, whet-
her administrative, educational or related to the intel-
lectual capital of the university. For the construction
of the ontology, the actors are trained to explain their
knowledge, promoting in this way a collaborative cul-
ture of creation and indexation of knowledge. The
captured knowledge provides a resource dedicated to
the representation of ontological knowledge, which
facilitates the search and navigation between concepts
related to the academic system. The architecture of
the system is able to integrate different ontologies,
and enables different types of reasoning and intelli-
gent information retrieval.
A similar approach was done by the Universidad
T
´
ecnica Particular de Loja (UTPL) research univer-
sity (Mora-Arciniegas et al., 2017). They propose
the use of semantic technologies to represent acade-
mic knowledge through ontologies, creating a set of
comprehensible and interoperable data. To fulfill their
objective, they developed an ontological network cal-
led Linked Academic Data (LDA) for the representa-
tion of the organizational structure and teaching plan-
ning. LDA was comprised of ontological resources
(FOAF, AIISO, VCARD, ORG, DCTERMS, VIVO),
non-ontological resources that required reengineering
to transform them into accessible resources, and the
creation of a new vocabulary.
A more recent proposal can be found at (Melgar
and Quilca, 2016), consisting in an architecture for
organizational memory systems in higher education
institutions. The proposed architecture for organiza-
tional memory is based on CESM model and Com-
monKADS methodology, concerned with the kno-
wledge representation for semantic search of docu-
ments. This approach allows adding semantic content
to the documents and to the information, enabling the
construction of knowledge bases. The semantic con-
tent added to the documents allows the retrieval of
information based on inference, unlike the relational
database that is based only on the content of a field.
To achieve this, they add semantic annotations to do-
cuments and link them to defined ontologies.
Finally, the authors of (Gasmi and Bouras, 2018)
proposed a system that allows to improve vocational
education, conducting an analysis of the gap between
education and industry. To this aum, they used a sy-
stem of semantic concordance based on ontological
models that allows to analyze these two domains. In
particular, the educational and industry profiles are re-
presented as profiles of O*NET competency frame-
works, which allows to analyze the gap according to
the structure of higher education and the peripherals
required by the industry in the social context.
3 DEVELOPMENT
In order to unify the scattered information in several
database repositories that occurs around the different
learning processes of higher education institutions,
we propose the construction of a new formal model
that captures the corresponding knowledge. We chose
the NeOn framework (Su
´
arez-Figueroa et al., 2012b)
as the basic was chosen for our implementation, be-
cause it offers features that allow to manage the life-
cycle of the ontological network very easily.
3.1 Elicitation of Requirements
In order to identify the purpose of creating the formal
model, who the end users would be and the features
it would enable, we went through a process of speci-
fying ontological requirements (Su
´
arez-Figueroa and
G
´
omez-P
´
erez, 2012). To fulfill the different tasks
specified by the NeOn methodology, a group of spe-
cialists was formed, composed of practitioners and
specialists from the academic side of higher educa-
tion institutions, who issued their criteria according
to their role in each task. Table 1 presents the group
of most frequent terms obtained from an interview for
KMIS 2018 - 10th International Conference on Knowledge Management and Information Sharing
162