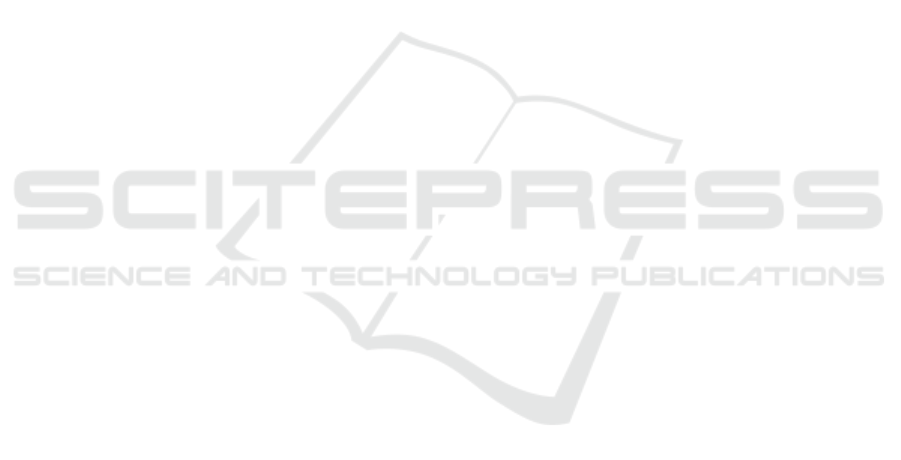
based on insights. The ultimate goal of BI is to
improve business decision-making and provide
actionable information in the right form for decision
makers at the right time and location (Sabherwal and
Becerra-Fernandez, 2011).
BI has a centric approach to data and therefore
relies heavily on various advanced data collection,
extraction, and analysis technologies. Experts often
consider data warehousing as the foundation of BI.
Organizations use dashboards for business performan-
ce management (BPM) making it easy to analyze and
visualize various performance metrics. The latest
phenomenon, Business Intelligence 2.0 (BI 2.0) allows
organizations to gather information from both enter-
prise databases and the Web. The querying of real-time
corporate data is in contrast to previous proprietary
querying tools that characterize previous BI software.
Web analytics tools such as Google Analytics can
analyze logs containing customer’s clickstream data,
which provide information about the trail of the user’s
online activities, thereby revealing the browsing and
purchasing patterns of the user. Organizations can use
web analytics for better website design, use heat maps
for optimizing product placement, analyze the
customer transactions better, and enable better product
recommendations. Organizations are also capable of
organizing and visualizing data by using multilingual
retrieval techniques such as knowledge mapping.
Business Analytics (BA) is the practice of
iterative, methodical exploration of an organization's
data, with an emphasis on statistical analysis.
Companies committed to data-driven decision-
making use business analytics. Data management is
the key infrastructure of BA. BA finds intelligence
within the organization's large volumes of data about
its products, services, customers, manufacturing,
sales, purchasing, and so on. Thus, the data stored in
the various corporate databases serve as inputs to BA
activities. Most of these data in the databases are
transaction oriented, which is not suitable for analysis
and report unless some processes manage the data.
Thus, the organization's data from various sources go
through integration or transformation through ETL
(extract, transform, and load) process and the
organizations often load this data into a data
warehouse, which is a centralized storage location of
data. The organization now has to use the stored data
to create business value, which requires data mining
or knowledge discovery techniques and analytical
techniques like mathematical optimization. These
techniques help the analysis of data and enable
finding useful information such as sales forecasts,
business constraints, and others. The data mining
techniques can be predictive modeling, clustering,
and association. Predictive modeling or analytics uses
statistical regression or artificial intelligence based
technologies for predicting future events upon
historical data. Prescriptive analytics, on the other
hand, involves mathematical optimization,
simulation, and so on. All these predictive and
optimization analytics are available in analytical
supply chain planning technologies such as advanced
planning scheduling (APS). Business process
management (BPM), which is similar to feedback in
open systems and a crucial component of BA, enables
monitoring, reporting, and correcting, which are the
three broad sets of business activities. Companies use
KPIs and other metrics to monitor the SC
performance. While seemingly similar, there is a
major difference between business intelligence vs.
business analytics: BI uses past and current data to
optimize the present operation while BA uses the past
and analyzes the present to prepare companies for the
future. Since both depend heavily on data analytics,
one can combine business intelligence and analytics
(BIA) as the preferred combined term.
Furthermore, SCM needs information technology
(IT) for coordination, monitoring, and optimization
of SC performance. It also needs management
processes like identifying metrics, objectives, goals,
parameters, targets, planning, defining communica-
tion methods, reporting, and feedback. These
functions are available as part of different information
system environments including SAP and Oracle.
Organizations, using IT, could institute performance
measurement processes, which could help decision-
makers to increase the effectiveness and efficiency of
their SC by focusing on the appropriate metrics (Cai
et al., 2009). In general, performance measurement is
vital in SCs. Gunasekaran and Kobu (2007) argued
that performance measurement could help the
organization identify the needs of the customer and
increase the product or service fulfillment as the
bottlenecks and opportunities are detected and
improved. The bottom line is making decisions based
on data, and enhancing process communication and
coordination is the key to success in SCM (Lim et al.,
2013). So, what is business analytics?
4 APPLICATION OF ANALYTICS
IN SCM
SCM developed quickly over time from traditional
procurement and supply management to the
integration from raw materials to end user
management. Analyzing large amounts of data and
Analytics in Supply Change Management: Is There a Dark Side?
249