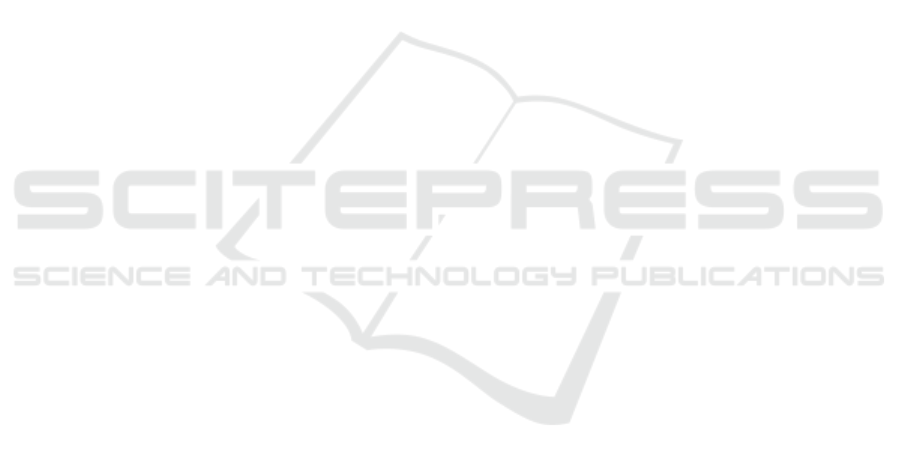
• Behavior : The Behavior of a System is represen-
ted as a sequence of States and transitions among
them. The States together with transitions spe-
cify the evolution in the values of the Parame-
ters. For defining this class, we used the definition
introduced in (Goel et al., 2009), under the fra-
mework of the Structure, Behavior and Function
(SBF) model. In the SBF model, a complex Sy-
stem could be described by its Structure, Behavior
and Function, and the Behavior of a System is re-
flected by the States and States transitions. Based
on the definition, the alignment
CM −core : Behavior v UFO−B : Complex Event
(5)
was proposed, in which Complex Event in UFO is
a subclass of Event (Perdurant). An Event repre-
sents possible transformations from a portion of
reality to another, and it is categorized into Atomic
Event and Complex Event, where a Atomic Event
has no integral parts, and a Complex Event is com-
posed of at least two events. In CM-core, the Be-
havior of a System stands for a complex compo-
sition of events which happen at different times
points, and within different contexts. Thus, the
above alignment was proposed.
3.2.3 Layer 3: Domain Ontologies for Condition
Monitoring in Manufacturing
A domain ontology represents specific domain know-
ledge and is only applicable to a certain domain. In
this study, the CM-core ontology will be specialized
into domain ontologies using the Top-Down appro-
ach. Figure 3 shows the whole ontological frame-
work, in which the domain ontologies are presented
at the bottom level. In the figure, rectangles are diffe-
rent ontologies or conceptual models, solid lines are
subsumption relations, and rectangles with dashed li-
nes indicate different levels of domain scope.
In the ontological framework, the Manufacturing
Condition Monitoring Ontology plays the central role
among all the domain ontologies. This ontology ma-
kes use of elements from other domain ontologies and
represents knowledge from both manufacturing and
condition monitoring domains. The other domain on-
tologies are included in the framework development
phase, but we do not introduce them in details in this
paper. We shortly discuss their main usage here: the
Product Ontology aims to provide a comprehensive
representation of manufacturing products and product
components. The Manufacturing Process Ontology
gives a formal representation of manufacturing pro-
cesses, such as cutting, drilling, milling, and casting.
The Manufacturing Resource Ontology provides kno-
wledge about manufacturing resources which are phy-
sical objects used for executing a range of operations
during different manufacturing processes. The Sensor
Ontology structures knowledge about sensors. This
ontology is specialized from the core reference on-
tology SSN. The Context Ontology contains the re-
presentations of contextual knowledge, including the
formal definitions of context entities such as person,
activity, location and time.
The CM-core ontology consists of 42 classes and
15 object properties. In the context of smart fac-
tories, the ontological framework was evaluated by
domain experts for its expressiveness and quality. It
paves the way for the development of an intelligent
system, which aims to perform condition monitoring
and maintenance tasks automatically.
4 CONCLUSIONS AND FUTURE
PERSPECTIVES
This paper presents an ontological framework which
is the basis for the development of an intelligent con-
dition monitoring system in manufacturing. The onto-
logical framework was developed using a Middle-Out
approach. It includes the CM-core ontology, which
is a core reference ontology for representing gene-
ral condition monitoring concepts and relations, and a
set of domain ontologies for gathering and structuring
knowledge in both condition monitoring and manu-
facturing domains. The CM-core ontology was alig-
ned with the foundational ontology UFO, for obtai-
ning a rigorous conceptualization. Also, the CM-core
ontology will be specialized into domain ontologies,
for integrating and representing domain-specific kno-
wledge.
Currently, we are in the process of developing the
domain ontologies that are at the bottom level of the
ontological framework. These domain ontologies can
be categorized into two types: (i) the ontologies for
structuring knowledge in the manufacturing domain;
and (ii) the ontologies for structuring knowledge in
the condition monitoring domain. The first category
of ontologies will provide the formalization of key
concepts in manufacturing, such as Product, Process,
Manufacturing Resource and Context. The second ca-
tegory of ontologies will focus on the representation
of concepts that are relevant to machinery prognos-
tics and health management (PHM), especially on the
conceptualization of Fault, Error and Failure. Future
perspectives include the integration of these domain
ontologies with the CM-core ontology, to provide se-
mantic capabilities for an intelligent condition moni-
toring system. On the other hand, a rule base will be
KEOD 2018 - 10th International Conference on Knowledge Engineering and Ontology Development
316