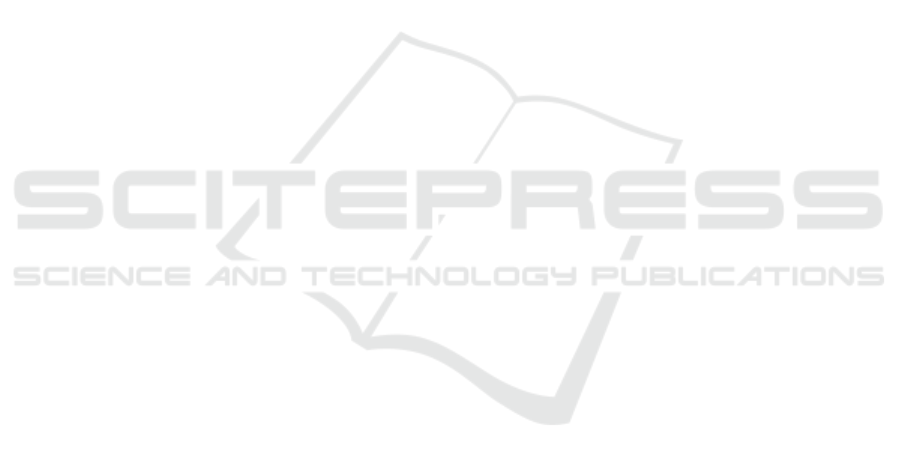
2 RELATED WORK
As mentioned before, several investigations about the
impact of environmental factors in public health are
already published. A relevant example is the
American Heart Association scientific statement on
"Air Pollution and Cardiovascular Disease" which
concluded that exposure to particulate matter (PM) air
pollution contributes to cardiovascular morbidity and
mortality (Brook et al., 2004). This study was updated
later giving new evidence of the impact of PM
exposure with cardiovascular diseases (Brook et al.,
2010). Moreover in D’Amato´s study (D’Amato et
al., 2010), urban air pollution and climate change
were demonstrated to be environmental risk factors of
respiratory diseases. A similar research indicates that
air pollution is a major preventable cause of increased
incidence and exacerbation of respiratory diseases
(Laumbach and Kipen, 2012).
Nevertheless, as said before, fewer studies in the
field of HF have been carried out, of which some most
relevant are analysed in the following lines. In 1995,
an article published in the American Journal of Public
Health investigated the association between hospital
admissions for congestive HF and air pollutants,
where ambient carbon monoxide levels were
positively associated with the admissions (Morris et
al., 1995). Two years later, another Canadian study
examined the role that ambient air pollution plays in
exacerbating cardiac disease (Burnett et al., 1997).
They found a positive association between daily
admissions fluctuations of congestive elderly HF
patients and variations in ambient concentrations of
carbon monoxide, nitrogen dioxide, sulfur dioxide,
ozone, and the coefficient of haze.
Additionally, other investigations about the effect
of meteorology in HF health status have been carried
out. In 2014, the International Journal of Cardiology
published a text where the relationships between
meteorological events and acute HF was globally
explored (Das et al., 2014). The results showed that
meteorological fluctuations appear most relevant in
the 3 days prior to the HF hospitalization with
temperature, demonstrating a relationship with HF. In
contrast, some authors demonstrated that the number
of hospitalizations for HF increases during winter
(Levin et al., 2018). Others concluded that there is a
substantial seasonal variation in HF hospitalizations
and deaths (Stewart et al., 2002).
However, to the best of our knowledge, there is still
further research to be done in order to better
determine the impact of a set of several
environmental factors on HF decompensations, being
the field where our work is focused on.
3 DATASETS
This study makes use of two different sets of data: one
related to the number of hospital admissions, and the
other one related to the environmental factors to
determine whether they have an impact on HF related
decompensations.
3.1 Hospital Admissions
The way to study HF decompensations is by means of
hospital admissions. Therefore, the first dataset
compiles the daily hospitalizations related to HF in
the public hospital OSI Bilbao-Basurto (Osakidetza),
located in the Basque Country (Spain). The hospital
has been gathering this information since 1994, but
only after 2010 this information started to be recorded
in Electronic Health Records. Due to the adaptation
to this new electronic system, the next two years the
information was not usable. Therefore, the usable
admissions dataset is from January of 2012 to August
of 2017.
The dataset consists of two attributes: (i) date of
admission for each patient, and (ii) date of discharge
of the patient. Nevertheless, only the first attribute
was used, being the date of discharge irrelevant for
this study. A total number of 8338 hospitalizations of
5343 different patients are available in this dataset,
with a mean of 4.02 admissions per day.
3.2 Environmental Data
This environmental dataset is separated in weather
information and air quality information. This
information was selected due to their demonstrated
impact on HF decompensations in previous studies
(Burnett et al., 1997; Das et al., 2014; Levin et al.,
2018; Morris et al., 1995; Stewart et al., 2002).
3.2.1 Weather
The Basque Agency of Meteorology (Euskalmet)
enables the possibility to access weather data
recorded since 2003, from the Open Data Euskadi
website (Basque Government, 2009). This
information is collected every ten minutes by each
station of Euskalmet distributed in Euskadi. The
different attributes that can be found in these datasets
are listed below (Table 1).
HEALTHINF 2019 - 12th International Conference on Health Informatics
52