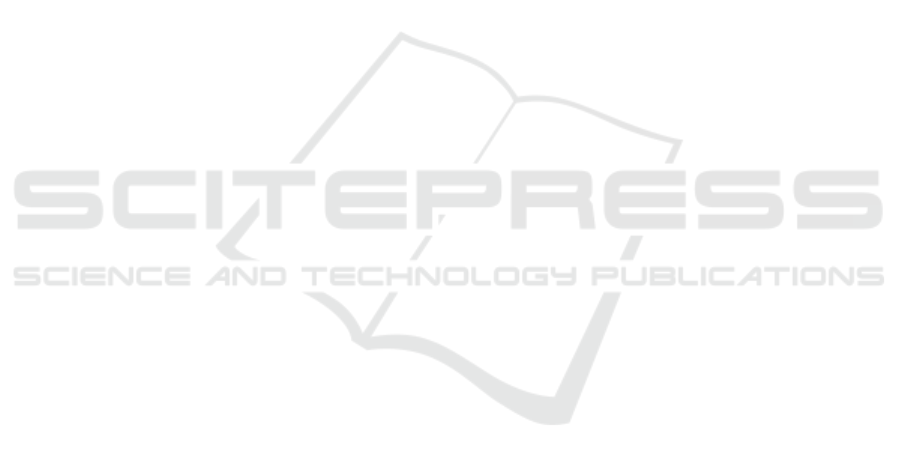
kinematics, a fact which is also observable in figure
6. HC shows a closer kinematic nature with respect to
PD, which results in some confusion and separation
difficulties. It may be observed that this similarity is
of aging nature, i.e., healthiness of healthy controls
cannot be assimilated to normative articulation. Age-
paired HC show certain similarities with PD patients
due to the effects of aging in articulation, although
this assumption must be proven. A comparison of PD
datasets with respect to normative sets may be not
resolving enough, as anticipated by the kinematic
analysis of PD and presbyphonic voice. It may be
argued that articulation kinematics is not sharp
enough to establish this differentiation, but it must be
taken into account that articulation instability is quite
well modelled by AKV pdf (Gómez, P., et al., 2017).
On the other hand, studies based on phonation
features, whether linear or non-linear, should be
subject to the same three-band tests to ensure that they
are sensitive to this separability problem. These
considerations raise immediate methodological
concerns regarding tests including PD patients and
healthy controls paired in age. It is unclear if this
separability problem is due to aging voice in healthy
controls, and in that case, if distortions found in PD
samples could be due also to aging, and not only to
pathology. The conclusion is that more tests with
larger number of samples should be conducted to
confirm or reject this observation, and that sharper
methods should be designed, both for the study of
vowel and speech dysarthria, as well as for studies
involving phonation, classically based on distortion
correlates as jitter, shimmer, signal-to-noise and non-
linear features. Especial care on this respect should be
observed regarding MFCC’s (mel-frequency cepstral
coefficients), as these features are known to be
sensitive both to dysphonia and to dysarthria. In this
sense, resolutive features are to be sought and tested
using three-band benchmarks in the way shown in the
present study.
5 CONCLUSIONS
From what has been discussed, the most relevant
conclusions to be summarized are the following:
• Paired tests show that articulation kinematics is
substantially different for PD and HC with respect
to normative subjects.
• This does not seem to be the case between PD and
HC subjects, as these subsets may share some
dysarthric features which may be due to aging
more than to neuromotor degeneration.
• This differentiation problem needs to be evaluated
as well in the case of phonation features,
otherwise there will not be full guarantee in using
phonation features to assess neuromotor
degeneration.
As a final remark, it must be stressed that these
conclusions are conditioned by the low size of the
datasets used, and require further validation with
larger number of subjects to be generalized.
ACKNOWLEDGEMENTS
Funded by grants TEC2016-77791-C4-4-R
(MINECO, Spain), CENIE_TECA-PARK_55_02
INTERREG V-A Spain – Portugal (POCTEP), 16-
30805A (CZ.1.05/2.1.00/03.0072), and LOl401 from
the Czech Republic Government.
REFERENCES
Anizah, S., et al. (2018). Objective Evaluation of
Bradykinesia in Parkinson’s Disease using
Evolutionary Algorithms. Proceedings of the 11th
International Joint Conference on Biomedical
Engineering Systems and Technologies (BIOSTEC
2018): 63-69. DOI: 10.5220/0006601700630069
Buchaillard, S., Perrier, P. and Payan Y., 2009. A
biomechanical model of cardinal vowel production:
muscle activations and the impact of gravity on tongue
positioning. Journal of the Acoustical Society of
America, 126(4): 2033-2051.
Brabenec, L., et al., 2017. Speech disorders in Parkinson's
disease: early diagnostics and effects of medication and
brain stimulation, J. Neural Transm., 124(3): 303–334.
Cantiniaux, S., et al., 2010. Comparative analysis of gait
and speech in Parkinson’s disease: hypokinetic or
dysrhythmic disorders? J. Neurol. Neurosurg.
Psychiatry, 81(2): 177–84.
Dauer, W. and Przedborski, S., 2003. Parkinson's disease:
Mechanisms and models. Neuron, 39(6): 889–909.
Deller J. R., Proakis J. G. and Hansen J. H. L., 1993.
Discrete-Time Processing of Speech Signals,
NewYork, Macmillan.
Dorsey, E. R., et al., 2007, 'Projected number of people with
Parkinson disease in the most populous nations, 2005
through 2030' Neurology, 68(5): 384-386.
Dromey, C., Jang, G. O. and Hollis, K., 2013. Assessing
correlations between lingual movements and formants,
Speech Communication, 55(2): 315-328.
Endres, D. M. and Schindelin, J. E., 2003. A New Metric
for Probability Distributions. IEEE Trans. on
Information Theory; 49(7): 1858-1860.
Georgiou T. and Lindquist, A., 2003. Kullback-Leibler
Approximation of Spectral Density Functions. IEEE
Trans. on Information Theory; 49(11): 2910-2917.
BIOSIGNALS 2019 - 12th International Conference on Bio-inspired Systems and Signal Processing
60