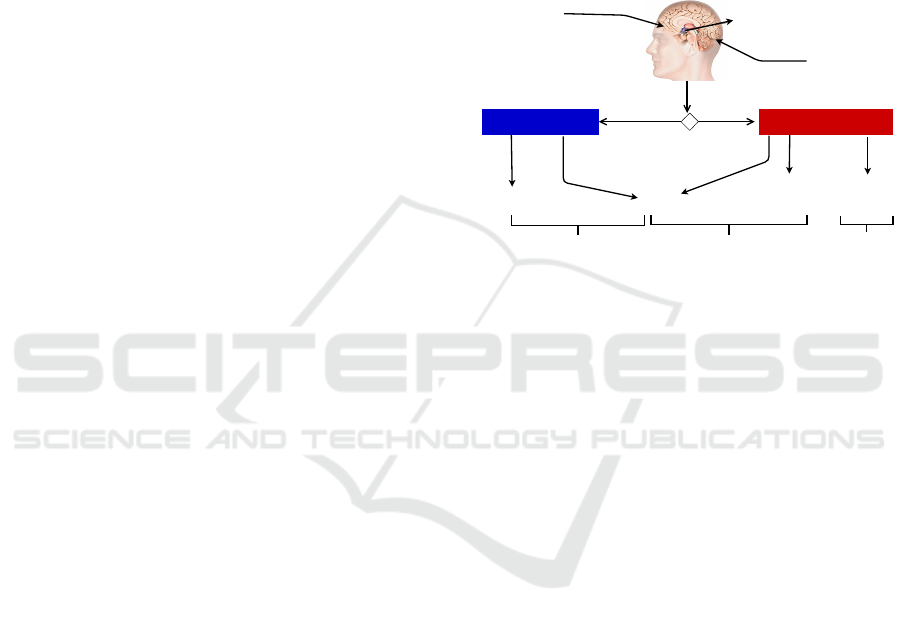
ing thermal environment and discussed the benefits
and approaches to designing a personalized, real-time
and energy efficient thermal comfort delivery appara-
tus that is based on the person’s estimated thermal
comfort sensation (Nkurikiyeyezu et al., 2018). Our
system (Lopez et al., 2018; Nkurikiyeyezu and Lopez,
2018) consists of an unobtrusive wrist-wearable de-
vice that records a person’s photoplethysmogram
(PPG) signal. The person’s heart rate variability (HRV)
is calculated from the PPG signal and is used to pre-
dict, in real-time, the person’s thermal comfort with an
accuracy greater than 90%. There were, however, con-
cerns about the system
'
s performance in real-world set-
tings. Certainly, HRV can change dues to other reasons
including physiological, pathological, neuropsycho-
logical, lifestyle factors, and, most importantly, stress
(Kim et al., 2018). This is because both thermal com-
fort and stress are regulated via a complementary ac-
tion of the sympathetic nervous system (SNS) and the
parasympathetic nervous system (PSN). Succinctly,
when the brain perceives a danger, the SNS ushers
a release of hormones to prepare the body to swiftly
react to life-threatening situations (for example, faster
heartbeat and an increase in airways for easier breath-
ing) and inhibits some non-urgent physiological needs
(e.g., digestion and sexual arousal). When the per-
ceived danger finally recedes, the PSN restores the
normal hormonal balance (Harvard Health, 2011). In
the case of thermal comfort, the brain’s hypothala-
mus serves as a “thermostat” of the body (Figure 1).
In a nutshell, the hypothalamus receives sensory in-
puts from thermo-receptors located in the skin, liver
and skeletal muscles and initiate appropriate processes
to keep constant the body
'
s core temperature. For
instance, when it is hot, the hypothalamus activates
heat dissipating and body cooling mechanisms such as
sweating and vasodilation. Conversely, when it is cold,
the hypothalamus activates thermogenesis mechanism
(e.g., shivering in skeletal muscle and heat generation
in brown adipose tissues) and other mechanisms to re-
duce heat dissipation (e.g.,cutaneous vasoconstriction
and piloerection) (Morrison, 2011). The human ther-
moregulation can be indirectly monitored via e.g., the
person’s heart rate variability (HRV). There is indeed
evidence that the very-low-frequency (VLF) band of
the HRV power spectra mirrors thermoregulatory vaso-
motor control activities (Thayer et al., 1997) and that
thermal discomfort generally alters heartbeat patterns
(Liu et al., 2008). Likewise, when in a stressful situa-
tion, the brain’s amygdala decodes the stress and sends
an appropriate message to the hypothalamus. The hy-
pothalamus, in turn, facilitates the release of hormones
such as adrenaline in the blood; thus, increases the per-
son’s heart rhythms, his respiration rate, his blood pres-
sure, and his pulse rate. Due to this hormonal changes,
the person becomes alert, his hearing and sight be-
come sharper and his blood sugar increases (Harvard
Health, 2011). The PNS restores this hormonal imbal-
ance when the stressor abates. However, if the stressor
persists for a long time, the hypothalamus keeps the
person on an enduring high alert by activating the cen-
tral stress response system —the hypothalamic pitu-
itary adrenal (HPA) axis —which releases cortisol, a
well-known biomarker of chronic stress (Schulz et al.,
1998).
Hypothalamus
Central
thermoreceptors
Peripheral
thermoreceptors
[Cold][Hot]
Thermal comfort decision
Temperature reduction
mechanisms
Temperature generation
mechanisms
Sweat glands
Vascular smooth
muscles
Brown adipose
tissues
Somatic
muscles
Transpiration VasodilatationVasoconstriction Heat production Shiver
Cholinergic neurons of the
sympathetic nervous system
Noradrenergic neurons of the
sympathetic nervous system
Somatic motor
neurons
Figure 1: A simplified process of human thermal regulation
—the hypothalamus checks the body’s core temperature and
starts necessary thermogenesis or heat dissipating processes
to maintain the core temperature [adapted from (Wikilivres,
2018)].
Over the past decade, there have been numerous
attempts to automate stress recognition. (Lopez et al.,
2014) developed an adaptive method that uses phys-
iological indices integrated into an intelligent multi-
steps discrimination process to predict stress occur-
rence and other workplace stress type. Their meth-
ods predicts workplace stress type with an accuracy
of 64%. In their review article, Alberdi and his coau-
thors (Alberdi et al., 2016) discuss various modalities
for stress recognition. They further propose a frame-
work for an automatic stress recognition system that
records a person’s physiological signals (e.g., elec-
troencephalogram (EEG), electrocardiography (ECG),
blood volume pulse(BVP), pupil diameter, eye gaze,
blinking, electrodermal activity (EDA), electromyo-
gram (EMG), respiration, etc..), behavioral (keystroke,
mouse usage, posture, facial expression, speech, mo-
bile phone usage, etc), environmental and contextual
(calendar events, location and ambiance) data to pre-
dict the likelihood of the person’s stress. Koldijk
(Koldijk et al., 2018) analogously used an amalgam
of sensor data (e.g., computer logging, posture, facial
expression and physiological features) and developed
a stress classifier that achieves a 90% accuracy. This is
an important achievement—especially since the stress
prediction was based on data recorded in realistic of-
fice settings. Nevertheless, in their paper, they failed
Thermal Comfort and Stress Recognition in Office Environment
257