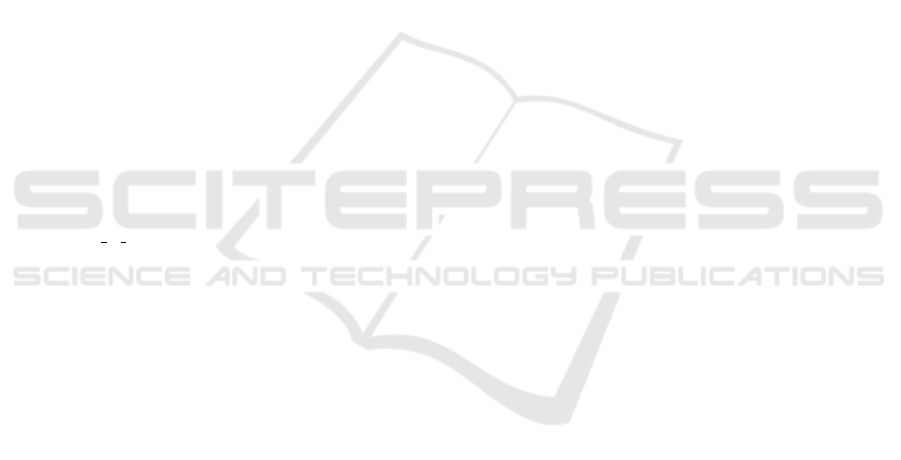
REFERENCES
Allder, S., Silvester, K., and Walley, P. (2010a). Managing
capacity and demand across the patient journey. Clin-
ical Medicine, 10(1):13 – 15.
Allder, S., Silvester, K., and Walley, P. (2010b). Under-
standing the current state of patient flow in a hospital.
Clinical Medicine, 10(5):441–444.
Audit-Commission (2003). Bed Management: Review of
National Findings.
Balaji, R. and Brownlee, M. (2009). Bed management op-
timization. Technical report, Infosys.
Baru, R. (2015). A decision support simulation model for
bed management in healthcare. Master’s thesis, Mis-
souri University of Science and Technology.
Boaden, R., Proudlove, N., and Wilson, M. (1999). An ex-
ploratory study of bed management. Journal of Man-
agement in Medicine, 13(4):234–250.
Bolt, S. and Sparks, R. (2013). Detecting and diag-
nosing hotspots for the enhanced management of
hospital emergency departments in queensland, aus-
tralia. BMC Medical Informatics and Decision Mak-
ing, 13(132).
Cho, H., Desai, N., Florendo, A., Marshall, C., Michalski,
J., and amd A. Dunn, N. L. (2017). E-dip: Early dis-
charge project: A model for throughput and early dis-
charge for 1-day admissions. https://bmjopen qual-
ity.bmj.com/content/5/1/u210035.w4128. Accessed
2017-11-16.
Eurostat (2017). Hospital beds by hospital ownership.
http://appsso.eurostat.ec.europa.eu/nui/show.do?data
set=hlth rs bds2&lang=eng. Accessed 2017-12-18.
Griffiths, J., Knight, V., and Komenda, I. (2013). Bed man-
agement in a critical care unit. IMA Journal of Man-
agement Mathematics, 24:137–153.
Husby, B. (2012). Integrating people, process and tech-
nology in lean healthcare. PhD thesis, University of
Michigan.
Kobis, D. and Kennedy, K. (2006). Capacity management
and patient throughput: putting the problem to bed.
Healthcare Financial Management, 60(10):88–92.
Lee, E., Shapoval, A., and Wang, Z. (2017). Inpatient bed
management to improve care delivery.
Mabire, C., Dwyer, A., Garnier, A., and Pellet, J. (2018).
Meta-analysis of the effectiveness of nursing dis-
charge planning interventions for older inpatients dis-
charged home. Journal of Advanced Nursing, 74(4).
Matos, J. and Rodrigues, P. (2011). Modeling decisions for
hospital bed management: A review. HEALTHINF
2011 - International Conference on Health Informat-
ics.
Mustafa, A. and Mahgoub, S. (2016). Understanding and
overcoming barriers to timely discharge from the pe-
diatric units. https://bmjopenquality.bmj.com/ con-
tent/5/1/u209098.w3772. Accessed 2017-09-25.
NAO (2000). Inpatient admissions and bed management in
nhs acute hospitals. Technical report, National Audit
Office.
NHS (2004). Achieving timely ‘simple’ discharge from
hospital a toolkit for the multi-disciplinary team.
http://webarchive.nationalarchives.gov.uk/200411231
20000/http://www.dh.gov.uk/assetRoot/04/08/83/67/
04088367.pdf. Accessed 2017-09-19.
Ortiga, B., Salazar, A., Jovell, A., Escarrabil, J., Marca, G.,
and Corbella, X. (2012). Standardizing admission and
discharge processes to improve patient flow: A cross
sectional study. http://www.biomedcentral.com/1472-
6963/12/180. Accessed 2017-12-11.
Patel, H., Morduchowicz, S., and Mourad, M. (2017). Us-
ing a systematic framework of interventions to im-
prove early discharges. The Joint Commission Journal
on Quality and Patient Safety, 43(4):189–196.
Petitgout, J. M. (2015). Discharge coordinator to improve
the patient discharge experience. Journal of Pediatric
Health Care, 29(6):509–517.
Proudlove, N., Gordon, K., and Boaden, R. (2003). Can
good bed management solve the overcrowding in ac-
cident and emergency departments? Emergency
Medicine Journal, 20(1):149–155.
Roswo, E., Adam, J., Coulombe, K., Race, K., and Ander-
son, R. (2003). Virtual instrumentation and real-time
executive dashboards: Solutions for health care sys-
tems.Nursing Administration Quarterly, 27(1):58 – 76.
Sant, J., Abela, G., and Farrugla, D. (2015). Delayed dis-
charges and unplanned admissions from the day care
unit at mater dei hospital, malta. Malta Medical Jour-
nal, 27(2):26–30.
Schmidt, R., Geisler, S., and Spreckelsen, C. (2013). De-
cision support for hospital bed management using
adaptable individual length of stay estimations and
shared resources. BMC Medical Informatics and De-
cision Making, 13(3).
Sherer, S., Meyerhoefer, C., and Levick, D. (2017).
Challenges to aligning coordination technology with
organizations, people and processes in healthcare.
https://scholarspace.manoa.hawaii.edu/handle/10125/
41586. Accessed 2018-02-22.
Teraiya, Y. and Makwana, P. (2015). A study of bed man-
agement the model in a hospital. The Joint Commis-
sion Journal on Quality and Pateint Safety, 3(5):489–
490.
Tsai, P. and Lin, F. (2014). An application of multi-attribute
value theory to patient-bed assignment in hospital ad-
mission management: an empirical study.
Webber-Maybank, M. and Luton, H. (2009). Making ef-
fective use of predicted discharge dates to reduce the
length of stay in hospital. Nursing Times, 105(15).
Webster, J., Davies, H., Stankiewicz, M., and Fleming, L.
(2011). Estimating the time involved in managing the
’unoccupied bed’: a time and motion study. Nursing
Economics, 29(6):317–322.
Wertheimer, B., JHacobs, R., Bailey, M., Holstein, S., Chat-
field, S., Ohta, B., and amd K. Hochman, A. H.
(2014). Discharge before noon: An achievable hospi-
tal goal. Journal of Hospital Medicine, 9(4):210–214.
Zhu, Z. (2011). Impact of different discharge patterns on
bed occupancy rate and bed waiting time: a simulation
approach. Journal of Medical Engineering & Technol-
ogy, 35(6-7):338–343.
Hospital Bed Management Practices: A Review
331