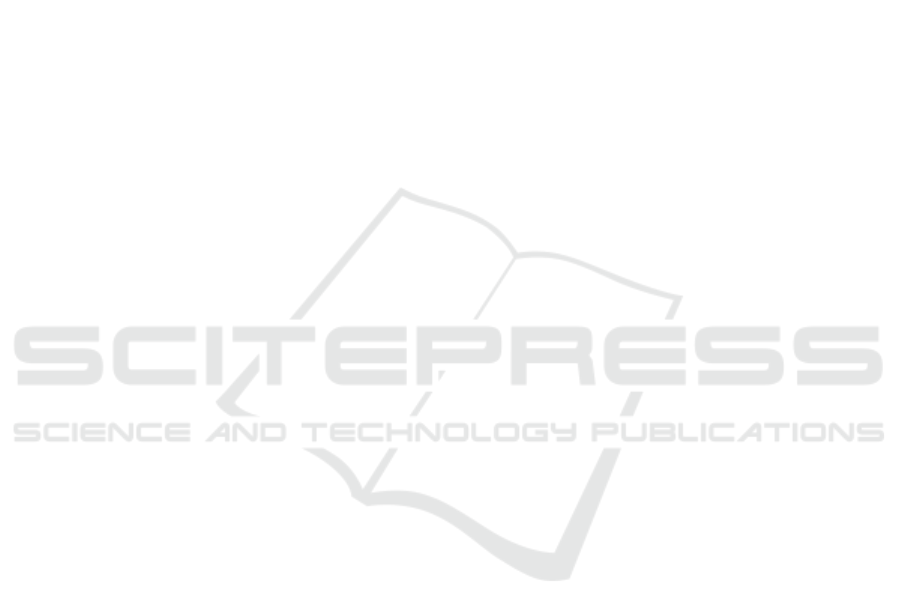
Modeling (Multi-)Morbidity and (Poly-)Pharmacy in Outpatient
Treatment with Gamma Distributions
Reinhard Schuster
1
, Thomas Ostermann
2
and Timo Emcke
3
1
Chair of Department of Health Economics, Epidemiology and Medical Informatics, Medical Advisory Board of Statutory
Health Insurance in Northern Germany, Katharinenstraße 11, 23554 L
¨
ubeck, Germany
2
Chair of Research Methodology and Statistics in Psychology, Witten/Herdecke University,
Alfred-Herrhausen-Straße 50, 58448 Witten, Germany
3
Chair of Department of Prescription Analysis, Association of Statutory Health Insurance Physicians,
Bismarckallee 1-6, 23812 Bad Segeberg, Germany
Keywords:
Multimorbidity, Polypharmacy, Outpatient Treatment, Big Data, Gamma Distribution, Shannon Entropy.
Abstract:
Polypharmacy is often direcly causes by age and gender dependent multimorbidity. Todays treatment con-
cepts, the individual decisions taken by physicians and the administration have to adress the complex needs of
multimorbid patients. For modeling those phenomena on a collective level of an entire federal state a sufficient-
ly large data repository is essential. The administrative bodies of the statutory health insurance in Germany
have the data necessary and built up an extensive skill-set and inexpensive free-software tool-set for those
evaluations. This study analyses the complete patient data of all outpatient treatments and drug prescriptions
in Schleswig-Holstein (Northern German federal state) in the first quarter of 2017. Well adopted probability
distributions for the frequency of diseases and drug groups decreasingly ordered within the classification sy-
stem for all patients and age/gender partitions are estimated. Subsequently the levels of multimorbidity and
polypharmacy (level of ICD-10/ATC-codes per quarter) are analysed in the same way. As a main result gamma
distributions provide a well-adjusted model class for ICD and ATC code frequencies in the present very large
routine dataset. The goodness-of-fit (full range of magnitudes of measurements) is much better than using
mean values and variances.
1 INTRODUCTION
Multimorbidity and polypharmacy are major challen-
ges for healthcare systems cf. (Dormann, H., Sonst,
F., Vogler, R., Patapovas, A., Pfistermeister, B., Plank-
Kiegle, B., Kirchner, M., Hartmann, N., Burkle, T.
Maas, R., 2013; Fortin, M., Hudon, C., Haggerty, J.,
Akker, M., Almirall, J., 2010; Islam, M. M., Valderas,
J. M., Yen, L., Dawda, P., Jowsey, T., McRae, I. S.,
2014; Jeschke, E., Ostermann, T., Vollmar, HC, Taba-
li, M., Matthes, H., 2012; Glynn, L.G., Valderas, J.M.,
Healy, P., Burke, E., Newell, J., Gillespie, P., Mur-
phy, A. W., 2011; Maher, R. L., Hanlon, J., Hajjar,
E. R., 2014; Mitty, E., 2009; Salwe, K. J., Kalyan-
sundaram, D., Bahurupi, Y., 2016). Costs and com-
plications for chronic patients usually increase accor-
ding to the number of comorbidities. Multimorbidity
and polypharmacy are often defined by the number of
diseases, disease groups, drugs or drug groups abo-
ve a certain threshold value using a low number of
categories. Adverse drug events, drug-drug and drug-
disease interactions are strongly connected with po-
lypharmacy cf. (Maher, R. L., Hanlon, J., Hajjar, E.
R., 2014). Polypharmacy can also increase the risk
of non-adherence, resulting in a suboptimal medicati-
on effectiveness and clinical consequences cf. (Glynn,
L.G., Valderas, J.M., Healy, P., Burke, E., Newell, J.,
Gillespie, P., Murphy, A. W., 2011). If the medication
non-adherence is not identified by the provider, they
either increase the initial dose or add a second agent
which in turn raises the health care costs and risk of
adverse drug events cf. (Jeschke, E., Ostermann, T.,
Vollmar, HC, Tabali, M., Matthes, H., 2012). The fre-
quency distributions for very large populations (big
data) are still mostly unknown because most publi-
cations consider special diseases with sample sizes
of a few hundred and up to thousand patients. Regi-
ster Studies usually address special aspects with den-
sity distribution analysis cf. (Johnell, K., Klarin, I.,
2007). The geriatric population is an example for high
prevalence of polypharmacy associated with multiple
comorbidities and advanced age cf. (Subeesh, V. K.,
Schuster, R., Ostermann, T. and Emcke, T.
Modeling (Multi-)Morbidity and (Poly-)Pharmacy in Outpatient Treatment with Gamma Distributions.
DOI: 10.5220/0007396503530358
In Proceedings of the 12th International Joint Conference on Biomedical Engineering Systems and Technologies (BIOSTEC 2019), pages 353-358
ISBN: 978-989-758-353-7
Copyright
c
2019 by SCITEPRESS – Science and Technology Publications, Lda. All rights reserved
353