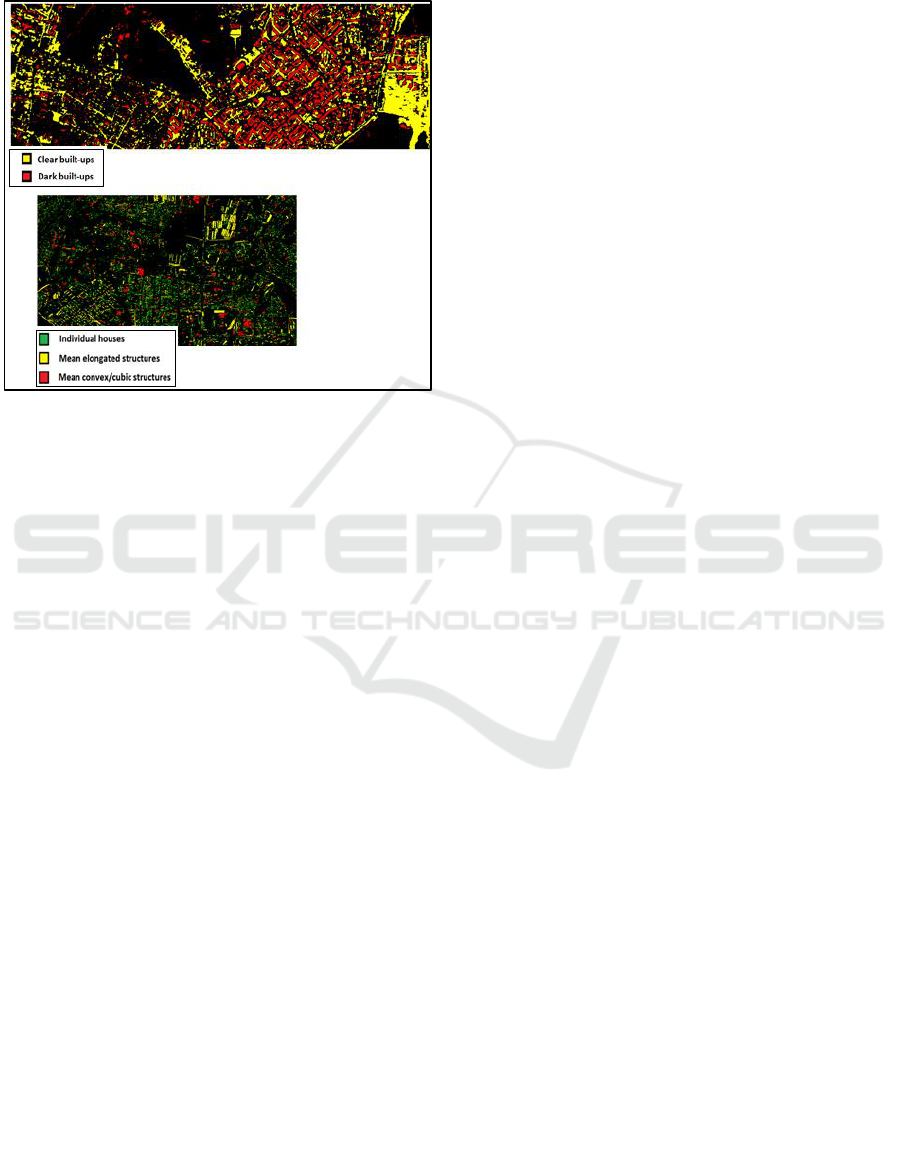
accuracy. Several studies have been already made in
the literature; nevertheless, the used knowledge
model was limited in terms of components.
Figure 8: Upper figure: urban frame identification over
Yakutsk city, lower figure: urban function extraction by
morphological attributes.
4 CONCLUSIONS
In this study, we presented an original
methodological scheme based on the joint use of a
hybrid knowledge model and artificial intelligence
for remote sensing application purpose. Several
application were already made using simple
knowledge model composed of biophysical or
morphological features or both at best. The idea
behind the methodology is to enrich the knowledge
model with several sources of information, which
could be very different at first sight, but will better
model the problem. The main issue will be related to
the standardisation of such sources of information, so
that the joint use of all the available sources could be
easily considered by the end-user.
The field of application related to such knowledge
model could be varied including objects mapping by
remote sensing imagery, objects characterisation and
identification, simulation of different environmental
phenomenon (e.g. urban sprawl, urban degrowth,
change detection, environmental phenomenon’s
management). Such knowledge model, will requires
the introduction of intelligence in both the structuring
procedure of the model and in the processing
sequence, including machine learning, deep learning
and neuronal networks methods. The joint use of
hybrid knowledge model coupled to the development
of intelligent rules and powerful training models will
contribute to the enhancement of image processing
and modelling methods and will constitute a new
exploration field.
ACKNOWLEDGEMENTS
French National Agency through Polar Urban Centers
(PUR, grant number ANR-15-CE22-0006) and
through Hyperspectral Imagery for Urban and
Environmental planning (HYEP, grant number ANR-
14-CE22-0016) supported this research.
SPOT 6 and Sentinel 2 data got the support of the
EQUIPEX GEOSUD (ANR-10-EQPX-20).
REFERENCES
Breen, C., Khan, L., and Ponnusamy. A., 2002. Image
Classification Using Neural Networks and Ontologies.
In Proc. 13th International Workshop on Database and
Expert Systems Applications, co-located with DEXA
2002, pages 98–102, Aix en-Provence, France.
Blaschke, T, Lang, S., and Hay, G.J., 2008. Object-Based
Image Analysis: Spatial Concepts for Knowledge-
Driven Remote Sensing Applications, New York, NY,
USA:Springer-Verlag.
Chi, M., Plaza, A., Benediktsson, J.A., Sun, Z. Shen, J. and
Zhu, Y., 2016. Big Data for Remote Sensing:
Challenges and Opportunities. Proceedings of the
IEEE, vol. 104, no. 11, pp. 2207-2219.
Durand, N. et al, 2007. Ontology-based object recognition
for remote sensing image interpretation, In 19th IEEE
international conference on tools with artificial
intelligence (ictai 2007), Patras, Greece, pp. 472-479.
Huang, X., and Jensen, J. R., 1997. A machine-learning
approach to automated knowledge-base building for
remote sensing image analysis with GIS data.
Photogrammetric Engineering and Remote Sensing, 63,
1185–1194.
Gadal, S., 2012. Geographic Space Ontology, Locus-
Object, and Spatial Data Representation Semantic
Theory. Universal Ontology of Geographic Space:
Semantic Enrichment for Spatial Data, IGI Global,
pp.28-52, 9786-1-4666-032-27-1.
Gadal, S., and Ouerghemmi, W., 2015. Morpho-Spectral
Recognition of Dense Urban Objects by Hyperspectral
Imagery. In ISPRS Geospatial week, 28 Sept – 02 Oct,
La Grande-Motte, France.
Gadal, S., and Ouerghemmi. W., 2016. Morpho-spectral
objects classification by hyperspectral airborne
imagery. In Proceedings of the 8th Workshop on
Hyperspectral Image and Signal Processing: Evolution
in Remote Sensing (WHISPERS), Los Angeles, CA.
Gadal, S., and Ouerghemmi. W., 2018. Multi-Level
Morphometric Characterisation of Built-ups in Siberian
Knowledge Models and Image Processing Analysis in Remote Sensing: Examples of Yakutsk (Russia) and Kaunas (Lithuania)
287