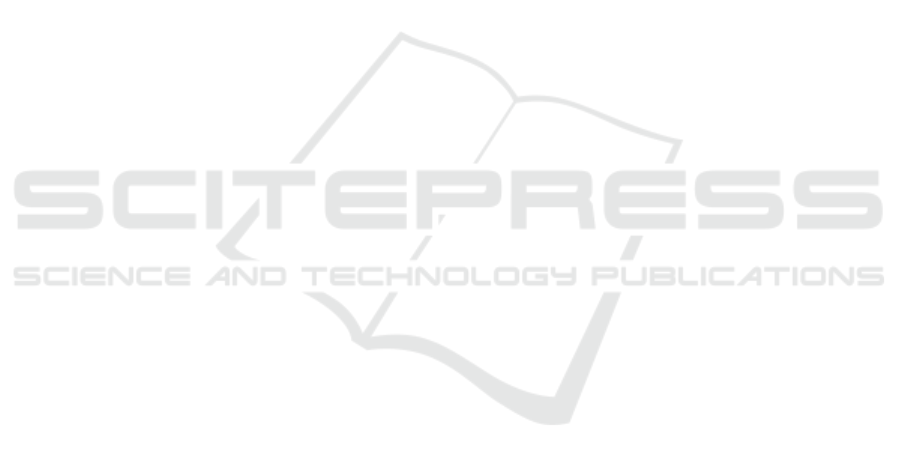
with equal distribution of examples in training would
be 1/50, i.e. only 2%. The accuracy achieved was
12.7%, that is substantially higher than the default.
Four clusters for which the evaluation was con-
siderably above average, were chosen for the sub-
sequent study of the temporality between news and
tweets. These were Brexit, Football - Euro 2016, Air
transport incidents and Politics. Regarding Football
- Euro 2016 the football national team was a rather
constant subject of discussion on social media in the
first semester of 2016, culminating in the final month
with its participation in the European Cup. However,
the same did not happen on the news side, where
the most frequent articles were published towards the
end of the period under observation. A similar pat-
tern was observed for Brexit. This indicates that, for
some texts, the press is more event-oriented, contrast-
ing with the more permanent focus of Twitter users.
The analysis Air transport incidents, which included
Brussels bombings, revealed that the press had a more
prominent role in the news diffusion, while comments
in social media appeared afterwards. Regarding the
cluster Politics, no pattern was identified. Perhaps,
with a more refined level of partitioning certain tim-
ing patterns would be more easily identified.
Regarding future work, we believe that the results
could be improved by adopting ontologies that would
enable to compute semantic distances between arti-
cles and tweets. Besides, tweets could also be ex-
panded with the use of synonyms or through word
embeddings (Mikolov et al., 2013). This would en-
hance the matching process of tweets to the lexicon
obtained from the articles.
ACKNOWLEDGMENT
This work has been supported by the project Centro-
01-0145-FEDER-000019 - C4 – Cloud Computing
Competences Centre” cofinanced, through the Sup-
port System for Scientific and Technological Re-
search - Integrated SR&TD Programs, by the Portugal
2020 Program (PT 2020), in the framework of the Re-
gional Operational Program of the Center (CENTRO
2020) and by the European Union through the Euro-
pean Regional Development Fund (ERDF).
REFERENCES
Bholowalia, P. and Kumar, A. (2014). EBK-means: A clus-
tering technique based on elbow method and k-means
in WSN. International Journal of Computer Applica-
tions, 105(9):17–24.
Cutting, D. R., Karger, D. R., Pedersen, J. O., and Tukey,
J. W. (1992). Scatter/Gather: A Cluster-based Ap-
proach to Browsing Large Document Collections. In
SIGIR 92, volume 51, pages 318–329, Copenhagen,
Denmark. ACM.
DVJ Insights and ING The Netherlands (2015). Impact of
social media on news (#SMING15). Technical report.
Hu, M., Liu, S., Wei, F., Wu, Y., Stasko, J., and Ma, K.-
L. (2012). Breaking news on twitter. In Proceedings
of the 2012 ACM annual conference on Human Fac-
tors in Computing Systems - CHI ’12, pages 275–279,
Austin, Texas, USA. ACM.
Kaplan, A. M. and Haenlein, M. (2010). Users of the world,
unite! The challenges and opportunities of Social Me-
dia. Business Horizons, 53(1):59–68.
Kwak, H., Lee, C., Park, H., and Moon, S. (2010). What is
Twitter, a Social Network or a News Media? In WWW
’10 Proceedings of the 19th International Conference
on World Wide Web, pages 591–600, Raleigh, North
Carolina, USA. ACM.
Mikolov, T., Sutskever, I., Chen, K., Corrado, G. S., and
Dean, J. (2013). Distributed representations of words
and phrases and their compositionality. In Advances in
neural information processing systems, pages 3111–
3119.
Newman, N. (2009). The rise of social media and its impact
on mainstream journalism. Technical Report Septem-
ber, Reuters Institute for the Study of Journalism, De-
partment of Politics and International Relations, Uni-
versity of Oxford.
Phuvipadawat, S. and Murata, T. (2010). Breaking news de-
tection and tracking in Twitter. In Proceedings - 2010
IEEE/WIC/ACM International Conference on Web In-
telligence and Intelligent Agent Technology - Work-
shops, WI-IAT 2010, pages 120–123, Toronto, ON,
Canada. IEEE.
Rocha, C., Jorge, A., Sionara, R., Brito, P., Pimenta, C., and
Rezende, S. (2016). PAMPO: using pattern matching
and pos-tagging for effective Named Entities recogni-
tion in Portuguese. arXiv preprint arXiv:1612.09535.,
pages 1–17.
Saleiro, P., Amir, S., Silva, M., and Soares, C. (2015).
POPmine: Tracking Political Opinion on the Web.
In 2015 IEEE International Conference on Computer
and Information Technology; Ubiquitous Computing
and Communications; Dependable, Autonomic and
Secure Computing; Pervasive Intelligence and Com-
puting (CIT/IUCC/DASC/PICOM), pages 1521–1526,
Liverpool, UK. IEEE.
Sankaranarayanan, J., Samet, H., Teitler, B. E., Lieberman,
M. D., and Sperling, J. (2009). TwitterStand: News in
Tweets. In Proceedings of the 17th ACM SIGSPATIAL
International Conference on Advances in Geographic
Information Systems - GIS ’09, pages 42–51, Seattle,
Washington, USA. ACM.
Sch
¨
utze, H., Manning, C. D., and Raghavan, P. (2008).
Evaluation in information retrieval, volume 39. Cam-
bridge University Press, New York, USA, 1st edition.
Zhao, W. X., Jiang, J., Weng, J., He, J., Lim, E.-p., Yan,
H., and Li, X. (2011). Comparing Twitter and Tradi-
tional Media using Topic Models. In Proceedings of
the 33rd European conference on Advances in infor-
mation retrieval (ECIR’11), pages 338–349. Springer,
Berlin, Heidelberg.
Association and Temporality between News and Tweets
507