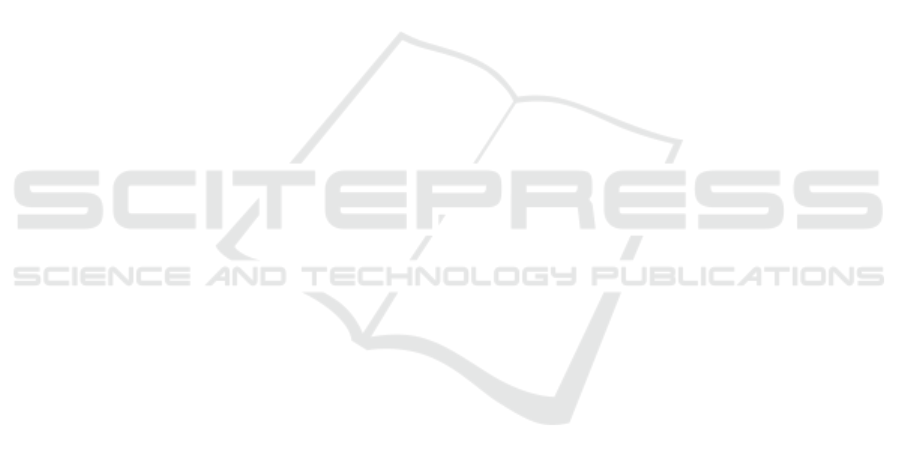
also shown better or comparable results than other
tools. After evaluation stage, both variants had not
large differences in their performance, and having
better results than the other tools except MACOED,
which although used older χ
2
test, shown better re-
sults for some datasets.
Further research will concern the evaluation stage,
as results after evaluation stage need to improve.
ACKNOWLEDGEMENTS
The authors would like to thank for financial contri-
bution from the STU Grant scheme for Support of
Young Researchers. This work was partially sup-
ported by the Scientific Grant Agency of The Slovak
Republic, Grant No. VG 1/0458/18, and APVV-16-
0484.
REFERENCES
Abdel-Basset, M. and Shawky, L. A. (2019). Flower polli-
nation algorithm: a comprehensive review. Artificial
Intelligence Review, 52(4):2533–2557.
Easton, D. F. et al. (2007). Genome-wide association study
identifies novel breast cancer susceptibility loci. Na-
ture, 447(7148):1087–1093.
Hindorff, L. A. et al. (2009). Potential etiologic and func-
tional implications of genome-wide association loci
for human diseases and traits. Proceedings of the Na-
tional Academy of Sciences, 106(23):9362–9367.
Jing, P.-J. and Shen, H.-B. (2014). MACOED: a multi-
objective ant colony optimization algorithm for SNP
epistasis detection in genome-wide association stud-
ies. Bioinformatics, 31(5):634–641.
Karaboga, D. and Basturk, B. (2007). A powerful and
efficient algorithm for numerical function optimiza-
tion: artificial bee colony (abc) algorithm. Journal
of Global Optimization, 39(3):459–471.
Kayabekir, A. E. et al. (2018). A Comprehensive Review
of the Flower Pollination Algorithm for Solving Engi-
neering Problems, pages 171–188. Springer Interna-
tional Publishing, Cham.
Mantegna, R. N. (1994). Fast, accurate algorithm for nu-
merical simulation of l
´
evy stable stochastic processes.
Phys. Rev. E, 49:4677–4683.
McDonald, J. H. (2014). G–test of goodness-of-fit. Hand-
book of biological statistics, pages 53–58.
Niel, C., Sinoquet, C., Dina, C., and Rocheleau, G. (2015).
A survey about methods dedicated to epistasis detec-
tion. Frontiers in genetics, 6:285–285.
Salgotra, R. and Singh, U. (2017). Application of muta-
tion operators to flower pollination algorithm. Expert
Systems with Applications, 79:112 – 129.
Sapin, E., Keedwell, E., and Frayling, T. (2015). An ant
colony optimization and tabu list approach to the de-
tection of gene-gene interactions in genome-wide as-
sociation studies [research frontier]. IEEE Computa-
tional Intelligence Magazine, 10(4):54–65.
Shang, J. et al. (2015). An improved opposition-based
learning particle swarm optimization for the detection
of snp-snp interactions. BioMed Research Interna-
tional, 2015:524821.
Sitar
ˇ
c
´
ık, J. and Luck
´
a, M. (2019). epibat: Multi-objective
bat algorithm for detection of epistatic interactions. In
2019 IEEE 15th International Scientific Conference
on Informatics, pages 000237–000242. IEEE.
Sun, Y., Shang, J., Liu, J.-X., Li, S., and Zheng, C.-H.
(2017). epiaco - a method for identifying epistasis
based on ant colony optimization algorithm. BioData
Mining, 10(1):23.
Tuo, S. (2018). Fdhe-iw: A fast approach for detect-
ing high-order epistasis in genome-wide case-control
studies. Genes, 9(9):435.
Tuo, S., Chen, H., and Liu, H. (2019). A survey on swarm
intelligence search methods dedicated to detection of
high-order snp interactions. IEEE Access, 7:162229–
162244.
Tuo, S., Zhang, J., Yuan, X., He, Z., Liu, Y., and Liu, Z.
(2017). Niche harmony search algorithm for detect-
ing complex disease associated high-order snp combi-
nations. Scientific Reports, 7(1):11529.
Tuo, S., Zhang, J., Yuan, X., Zhang, Y., and Liu, Z. (2016).
Fhsa-sed: Two-locus model detection for genome-
wide association study with harmony search algo-
rithm. PLOS ONE, 11(3):1–27.
Wan, X. et al. (2010). Boost: A fast approach to detecting
gene-gene interactions in genome-wide case-control
studies. The American Journal of Human Genetics,
87(3):325–340.
Wang, R., Zhou, Y., Qiao, S., and Huang, K. (2016). Flower
pollination algorithm with bee pollinator for cluster
analysis. Information Processing Letters, 116(1):1 –
14.
Wang, Y., Liu, X., Robbins, K., and Rekaya, R. (2010).
Antepiseeker: detecting epistatic interactions for case-
control studies using a two-stage ant colony optimiza-
tion algorithm. BMC Research Notes, 3(1):117.
Yang, X.-S. (2012). Flower pollination algorithm for global
optimization. In Durand-Lose, J. and Jonoska, N., ed-
itors, Unconventional Computation and Natural Com-
putation, pages 240–249, Berlin, Heidelberg. Springer
Berlin Heidelberg.
Yuan, L., Yuan, C.-A., and Huang, D.-S. (2017). Faacose:
A fast adaptive ant colony optimization algorithm for
detecting snp epistasis. Complexity, 2017.
Zhang, Y. and Liu, J. (2007). Bayesian inference of epistatic
interactions in case-control studies. Nature genetics,
39:1167–73.
BIOINFORMATICS 2021 - 12th International Conference on Bioinformatics Models, Methods and Algorithms
126