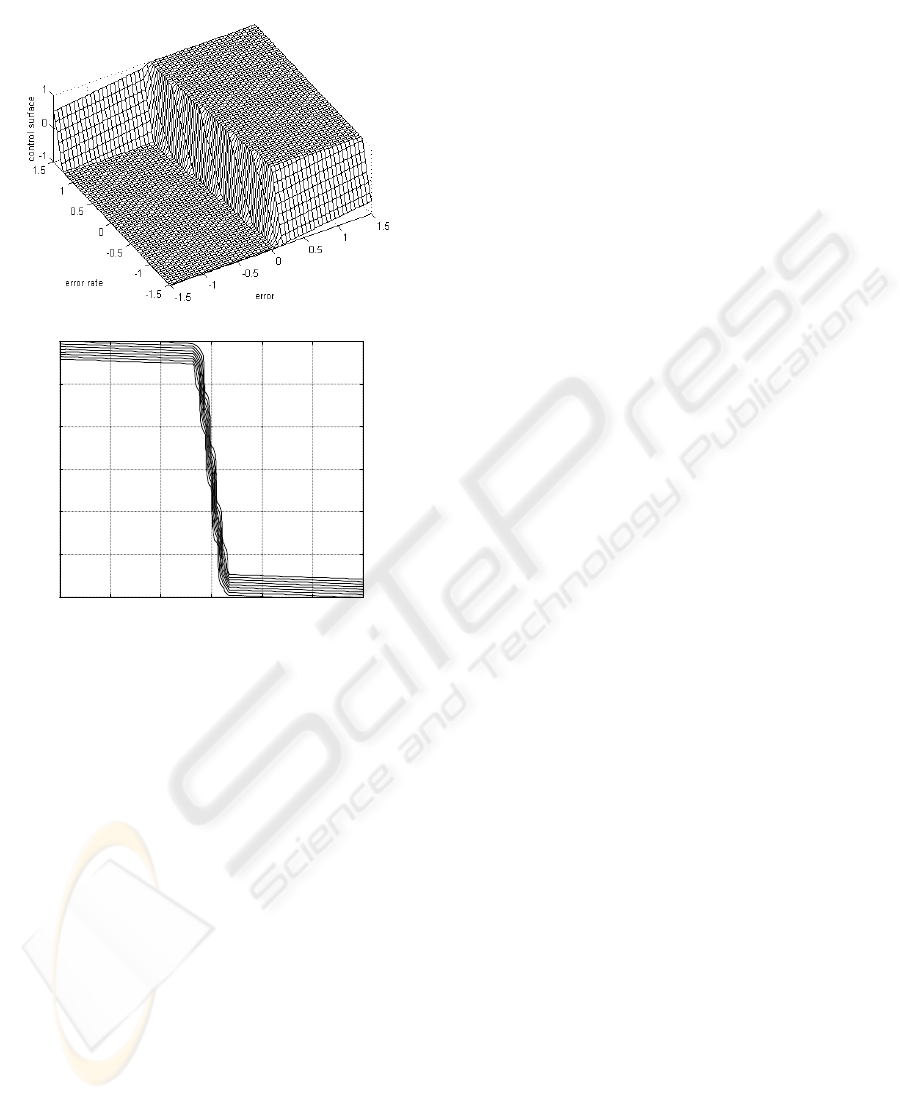
-1.5 -1 -0.5 0 0.5 1 1.5
-1.5
-1
-0.5
0
0.5
1
1.5
error
error rate
Figure 4: Control surface and sliding surface for an
ASMFC controller with only 9 fuzzy if-then rules.
5 CONCLUSIONS
In this short article an adaptive sliding mode fuzzy
control approach is proposed with some analysis of
its property for addressing nonlinear control
problems. This approach combines the concept of a
branch of nonlinear control theory, namely SMC,
and that of a fuzzy inference system that can
uniformly approximate any nonlinear function with
arbitrary degree of accuracy. In this sense, global
stability of the control system designed by this
approach can be mathematically established (Jang,
1992b; Hung, Gao and Hung, 1993; Slotine and Li,
1991). Nevertheless, be aware that the presented
approach seems only applicable to the class of
nonlinear systems over which the feedback
linearization technique can be performed.
REFERENCES
Chen, Y.-Y. and T.-C. Tsao, 1989. A description of the
dynamic behavior of fuzzy systems, IEEE Trans. Syst.
Man and Cybernet., vol. 19 (4), pp. 745-755.
Driankov, D., H. Hellendoorn, and M. Reinfrank, 1993.
An introduction to fuzzy control, Springer-Verlag,
Berlin/New York/Heidelberg.
Hoffmann, F. and O. Nelles, 2001. Genetic programming
for model selection of TSK-fuzzy systems, J. of
Information Sciences, vol. 136 (4), pp. 7-28.
Hung, J.Y., W. Gao and J.C. Hung, 1993. Variable
structure control: a survey, IEEE Transactions on
Industrial Electronics, vol. 40 (1), pp.2-21.
Hwang, G.-C. and S.-C. Lin, 1992. A stability approach to
fuzzy control design for nonlinear system, Fuzzy Sets
and Systems, vol. 48, pp.279-287.
Jang, J.-S. R., 1992a. Self-learning fuzzy controllers based
on temporal back propagation, IEEE Trans. Neural
Net., vol. 3 (5), pp. 714-723.
Jang, J.-S. R., 1992b. Neuro-fuzzy modeling: architecture,
analyses and applications, Ph.D. dissertation,
Department of Electrical Engineering and Computer
Science, University of California Berkeley, Berkeley,
CA.
Jang, J.-S. R., 1993. ANFIS: adaptive-network-based
fuzzy inference systems, IEEE Trans. Syst. Man and
Cybernet., vol. 23 (3), pp. 665-685.
Palm, R., 1992. Sliding mode fuzzy control, in Proc. of
IEEE International Conference on Fuzzy Systems,
pp.519-526.
Palm, R., D. Driankov, and H. Hellendoorn, 1996. Model-
based fuzzy control, Springer-Verlag, Berlin/New
York/Heidelberg.
Palm, R. and C. Stutz, 2003. Generation of control
sequences for a fuzzy gain scheduler, Int. J. of Fuzzy
systems, vol. 5 (1), pp. 1-10.
Passino, K.M. and S. Yurkovich, 1998. Fuzzy control,
Addison-Wesley Longman Inc..
Slotine, J.J.E. and W. Li, 1991. Applied nonlinear control,
Prentice-Hall, Englewood Cliffs, NJ.
Takagi, T. and M. Sugeno, 1983. Derivation of fuzzy
control rules from human operator’s control actions, in
Proc. of IFAC Symp. Fuzzy Inform., Knowledge
Representation and Decision Analysis, pp. 55-60.
Takagi, T. and M. Sugeno, 1985. Fuzzy identification of
systems and its applications to modeling and control,
IEEE Transactions on Syst. Man and Cybern., vol. 15,
pp.116-132.
Wang, L.-X., 1993. Stable adaptive fuzzy control of
nonlinear systems, IEEE Trans. Fuzzy Syst., vol. 1 (1),
pp. 146-155.
AN ADAPTIVE SLIDING-MODE FUZZY CONTROL (ASMFC) APPROACH FOR A CLASS OF NONLINEAR
SYSTEMS
197