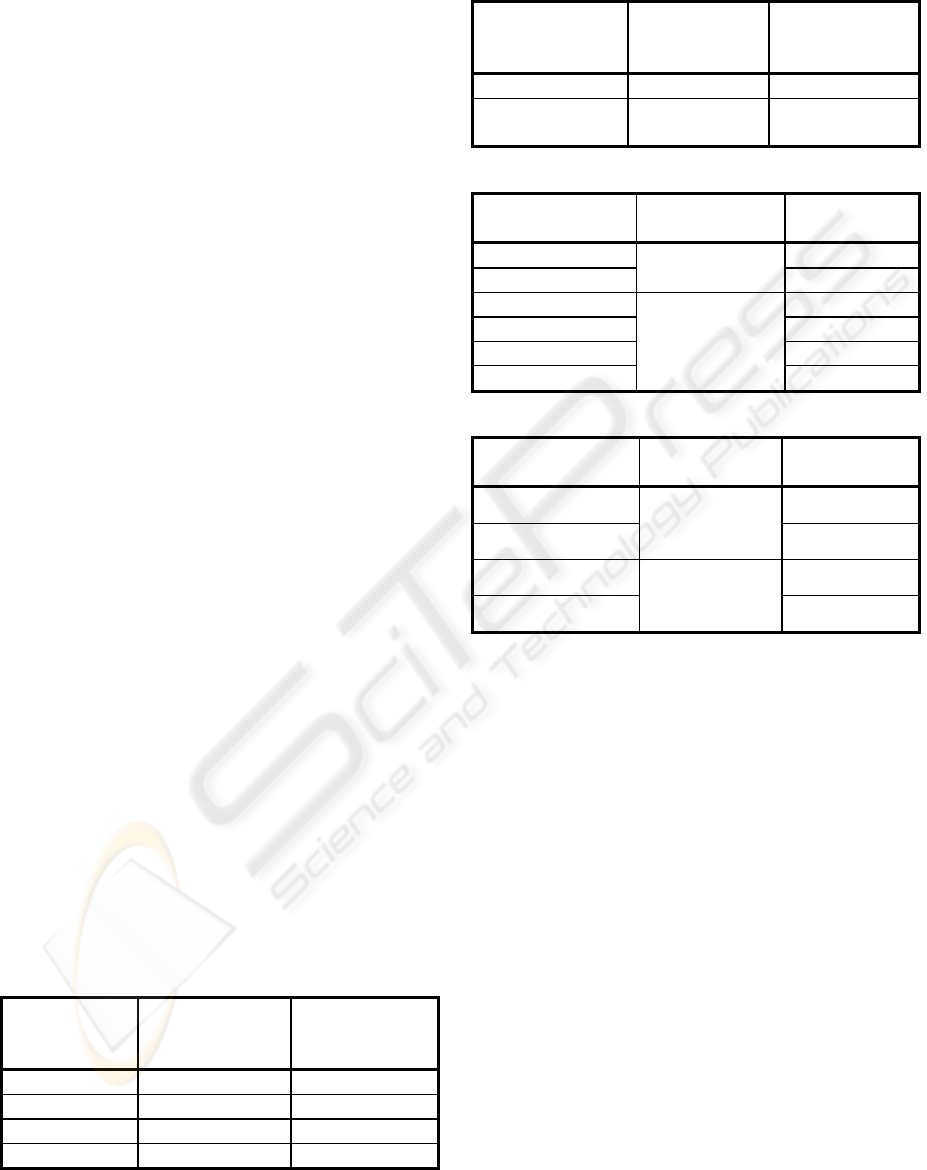
RxC matrix ( CnnCC <
= 2,
1
) representing the
initial image of L gray levels and I the distorted
variant resulted from
0
by superimposing noise
, , ,
1
()
Σ,0N
Ri ,...,1=∀
()
njjnk ,...,1−= ,...,1 Cj
,
jiji
,,
. The restoration process of the
image I is described as follows.
() () ()
kkIkI η+=
0
Step 1. Compute the image I’ by decorrelating
the noise component,
,
~ ,
''
0
,,,
η+Φ=Φ=
ji
T
ji
T
ji
III
ηΦ=η
T
'
()
',0 ΣN Λ=
ΦΦ=Σ
T
' , where
{}
n
diag λλλ=Λ ,...,,
21
.
Step 2. The noise component is removed for
each pixel P of the image I’ using the
multirezolution support of I’ by the labeling method
of each wavelet coefficient of P, resulting I”.
'η
()
0
,,,
'"
ji
T
jiji
IIMSTI Φ≅=
,
Ri ,...,1=
, .
1
,...,1 Cj =
Step 3. An approximation
0
≅
of the initial
image
0
is produced by applying the inverse
transform of
to I”.
T
T
Φ
3 COMPARATIVE ANALYSIS ON
THE PERFORMANCE OF THE
NOISE REMOVAL
ALGORITHMS
A series of experiments were performed, different
256 gray level images being preprocessed aiming the
contrast enhancement, increasing enlightens and
noise removing by filtering them. Our experiments
use the averaging and respectively binomial filtering
techniques. The parameters involved in the
mentioned algorithms were tuned taking into
account the following factors: the distortion degree
of the inputs, the particular smoothing filter, the
volume of the resulting accepted data (Cocianu,
2002).
A synthesis of the comparative analysis on the
quality and efficiency corresponding to the
restoration algorithms presented in the paper is
supplied in Table 1, Table 2, Table 3 and Table 4.
Table 1
Restoration
algorithm
Mean
error/pixel
N(30,150)
Mean
error/pixel
N(50,200)
Mean 9.422317 12.346784
HRBA 9.333114 11.747860
HSBA 9.022712 11.500245
HBA 9.370968 11.484837
Table 2
Restoration
algorithm
Mean
error/pixel
N(50,200)
Mean
error/pixel
N(90,250)
Mean 12.346784 102.528893
The innovation
algorithm
11.647346 94.912895
Table 3
Restoration
algorithm
Type of
noise
Mean
error/pixel
MMSE 50.58
AMVR
U(40,70)
8,07
MMSE 46.58
AMVR 9.39
MNR2 12.23
NFPCA
N(50,100)
10.67
Table 4
Restoration
algorithm
Type of
noise
Mean
error/pixel
MNR
)
1
h
11.6
MNR
)
2
h
N(0,100)
9.53
MNR
)
1
h
14.16
MNR
)
2
h
N(0,200)
11.74
REFERENCES
Brockwell, P., Richard, A., 1985, Time Series: Theory
and Methods, Springer Verlag
Cocianu, C., State, L., Vlamos, P.,2002, On a Certain
Class of Algorithms for Noise Removal in Image
Processing: A Comparative Study, In Third IEEE
Conference on Information Technology ITCC-2002,
Las Vegas, Nevada, USA, April 8-10, 2002
Cocianu, C., State, L., Stefanescu, V., Vlamos, P., 2003,
Noise Removal Techniques Using the Multiresolution
Representation, In Proceedings of 32nd International
Conference on Computer&Industrial Engineering
(ICC&IE), Limerick, Ireland, August 11th-13th, 2003
Jain, A. K., Kasturi, R., Schnuck, B. G., 1995, Machine
Vision, McGraw Hill
Sonka, M., Hlavac, V., 1997, Image Processing, Analyses
and Machine Vision, Chapman & Hall Computing
Stark, J.L., Murtagh, F., Bijaoui, A., 1995, Multiresolution
Support Applied to Image Filtering and Restoration,
Technical Report
State, L, Cocianu, C, Vlamos, P.., 2001, Attempts in Using
Statistical Tools for Image Restoration Purposes, In
Proceedings of SCI2001, Orlando, USA, 2001
ON THE EFFICIENCY OF A CERTAIN CLASS OF NOISE REMOVAL ALGORITHMS IN SOLVING IMAGE
PROCESSING TASKS
321