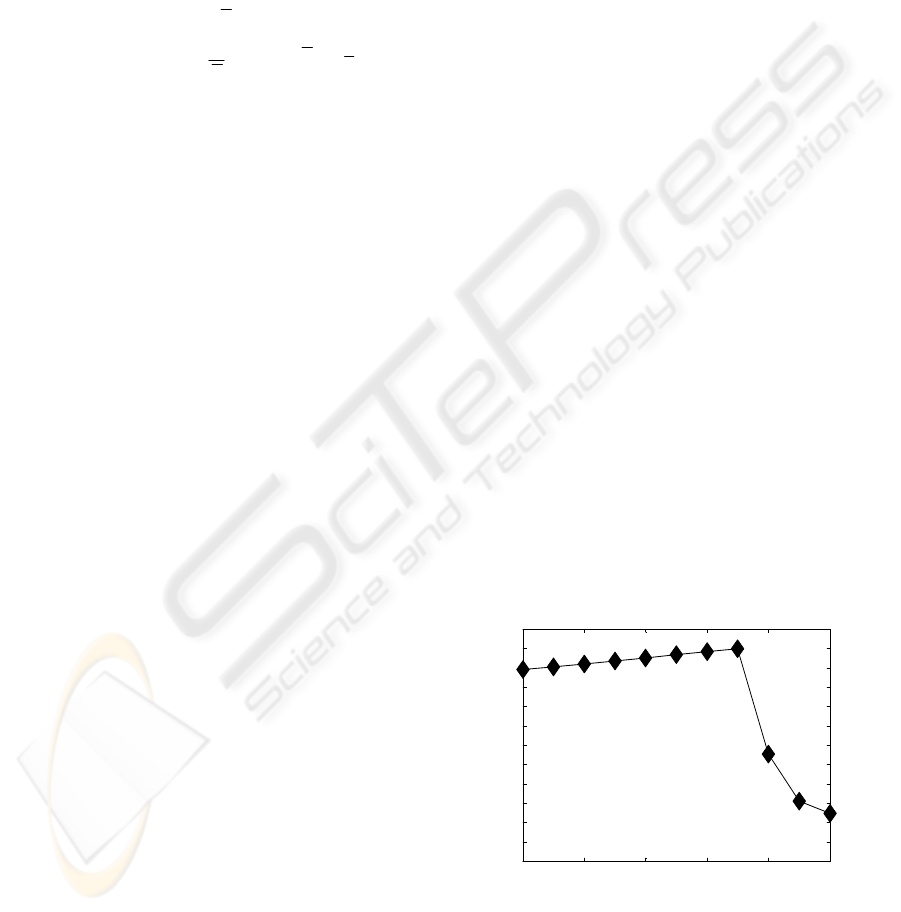
confidence in the estimate. When
0=
the filtered
data are equal to the prediction (complete confidence
in the estimate), when
1
no filtering is applied
to the measurement.
In applying the Smyth algorithm to the filtered
data of equation (5) one has to select the
regularization parameter. In order to investigate the
algorithm performance as a function of the
regularized parameter, the following procedure has
been applied: the regularization parameter
is
made varying from 0 to 1 in
1+h steps:
0
0
=
, hh
h
hh
,...,1,
1
1
=+=
−
αα
,
1
h
The noise free predicted data
from
the estimate obtained with parameter
1−h
)(
ˆ
1
ky
h−
α
are used
to generate the filtered data with parameter
h
,
accordingly to the equation:
)(
ˆ
)1()()(
1
kykyky
h
ahh
h
a
−
−+=
(6)
The Smyth algorithm is applied to this data; then
its results are used to compute a new data prediction,
and the procedure is iterated. The Smyth algorithm
is also intialized with the solution obtained at the
previous step. Substantially, the algorithm is
successively applied to convex combinations of
noise-free data estimates obtained from the previous
step estimation and measurements The procedure is
initialized at step 0 with the ESPRIT algorithm,
whose data prediction is employed to compute the
regularized data with
0
. The same procedure
described in the previous section has then been
applied to evaluate the performance of the Smyth
algorithm as a function of the regularization
parameter. The results presented report the value in
dB of 1/MSE (equation (4)) against the value of
from 0 to 1, at 0.1 steps. The maximum corresponds
to the optimal regularization parameter. The two
limit cases corresponds to the independent
application of ESPRIT (
0=
) and Smyth ( 1
)
algorithms. A sample of the results obtained are
reported in Figures 4, 5, 6: the figures refer to the
estimation of two sinusoids, at SNR of –5, -10, -20
dB respectively. The results reported show the
existence of an optimal value of the regularization
parameter; the Smyth algorithm, applied with the
initialization process described and with the optimal
regularization parameter, has a performance at low
SNR which is better than that of both the "pure
Smyth" algorithm and ESPRIT. The average
performance gain with respect to ESPRIT of the
regularization approach ranges from the 8 dB of the
–10 dB SNR case (Figure 5) to the 3 dB of the –20
dB SNR case (Figure 6). Note that the performance
gain with respect to the "pure Smyth" algorithm can
be much larger (up to 45 dB, Figure 4). This general
behaviour is systematic in all the simulations test
performed, with sometimes even larger performance
gains.
It is natural to ask what is the optimal
regularization parameter at high SNR. A typical
behaviour is shown in Figure 7, obtained in the case
of estimation of a single tone with SNR of 30 dB:
the optimal value is 1, indicating that the best
performance is given by the unregularized Smyth
algorithm.
It needs to be observed, though, that the
performance curve as a function of the regularization
parameter as determined through the pseudo Monte
Carlo approach does not exhibit any specific
behaviour that can be further exploited. In particular,
Figures 4-6 has been purposely chosen to illustrate
the several different behaviours observed. Figure 4
illustrates the situation in which the experimental
curve exhibits a unique maximum, with monotone
behaviour of the performance curve before and after
the extremal point; Figure 5 shows the presence of
multiple maxima and minima; Figure 6 presents a
case in which, though there does exist a unique
maximum, there is also a minimum within the
regularization interval, and the performance function
does not have a monotone behaviour before the
reaching of the maximum. Moreover, for some
values of the regularization parameter, the
performance is worse with respect to both ESPRIT
and unregularized Smyth. This diversity in the
performance curve behaviour may represent a
serious obstacle to an efficient implementation of a
computational scheme aimed at exploiting the better
performance of the regularized approach. Some
guidelines and critical evaluations toward this goal
are reported in the next section.
0 0.2 0.4 0.6 0.8 1
0
5
10
15
20
25
30
35
40
45
50
55
60
1/MSE (dB)
regularization parameter
SNR = -5 dB
2 sinusoids
Figure 4: Estimation results obtained from the Smyth
algorithm and the regularization procedure as a function of
the regularization parameter; SNR: -5 dB.
ICINCO 2004 - SIGNAL PROCESSING, SYSTEMS MODELING AND CONTROL
302