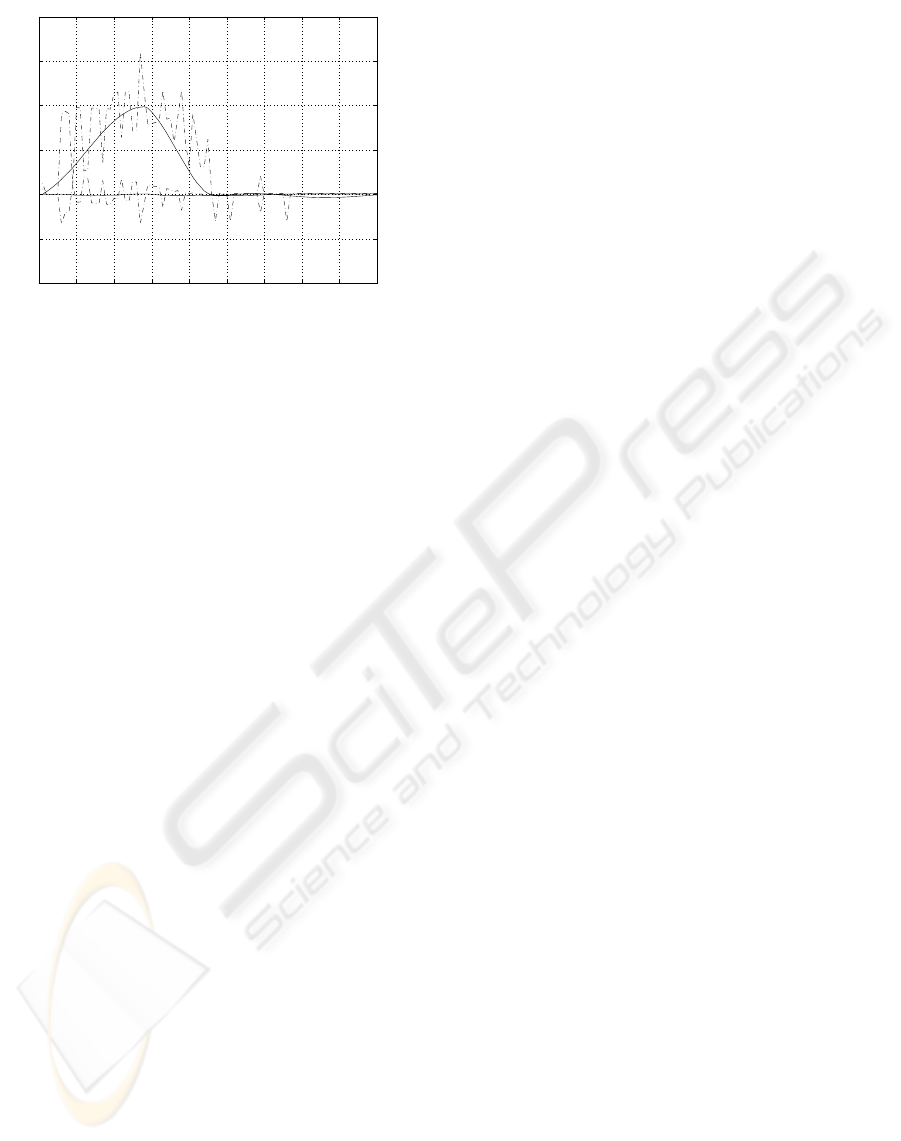
0 10 20 30 40 50 60 70 80 9
−2
−1
0
1
2
3
4
No samples [20 ms]
Joint Velocity [rad/s]
Comparison of Joint Velocities
Figure 11: Joint velocities, δq. Solid – fuzzy visual servo,
and dashed – classical visual servo.
ities are depicted in Fig. 11, where it is clear that the
classical controller presents undesirable and danger-
ous oscillations in the joint velocities. This fact is due
to the high proportional gain that the classical con-
troller must have to follow the trajectory, which de-
mands high velocity. This effect is easily removed by
slowing down the classical controller. But in this case,
the fuzzy controller clearly outperforms the classical
controller. The classical controller can only follow
the trajectory without oscillations in the joint veloc-
ities if the robot takes 1.5s to move from one point
to the other. In this case, the classical controller is
about 50% slower than the fuzzy model-based con-
troller proposed in this paper.
8 CONCLUSIONS
This paper introduces an eye-in-hand image-based vi-
sual servoing scheme based on fuzzy modeling and
control. The fuzzy modeling approach was applied
to obtain an inverse model of the mapping between
image features velocities and joints velocities. This
inverse model is added to a fuzzy compensator to be
directly used as controller of a robotic manipulator
performing visual servoing, for a given image features
velocity profile.
The obtained results showed that both the classi-
cal and the fuzzy controllers can follow the image
features velocity profile. However, the proportional
gain of the classic visual servoing must be very high.
This fact justifies the oscillations verified in the joint
velocities, which are completely undesirable in robot
control. For that reason, the inverse fuzzy control pro-
posed in this paper performs better.
As future work, the proposed fuzzy model based
control scheme will be implemented in the experi-
mental test-bed. Note that an off-line identification
of the inverse fuzzy model must first be performed.
The complete automation of this identification step is
also under study.
ACKNOWLEDGEMENT
This work is supported by the “Programa de Financia-
mento Plurianual de Unidades de I&D (POCTI) do Quadro
Comunit
´
ario de Apoio III”, by program FEDER, by the
FCT project POCTI/EME/39946/2001, and by the “Pro-
grama do FSE-UE, PRODEP III, acc¸
˜
ao 5.3, no
ˆ
ambito do
III Quadro Comunit
´
ario de apoio”.
REFERENCES
Babu
ˇ
ska, R. (1998). Fuzzy Modeling for Control. Kluwer
Academic Publishers, Boston.
Baptista, L., Sousa, J. M., and da Costa, J. S. (2001). Fuzzy
predictive algorithms applied to real-time force con-
trol. Control Engineering Practice, pages 411–423.
Espiau, B., Chaumette, F., and Rives, P. (1992). A new
approach to visual servoing in robotics. IEEE Trans-
actions on Robotics and Automation, 8(3):313–326.
Gonc¸alves, P. S. and Pinto, J. C. (2003a). Camera con-
figurations of a visual servoing setup, fora2dof
planar robot. In Proceedings of the 7th Interna-
tional IFAC Symposium on Robot Control, Wroclaw,
Poland., pages 181–187, Wroclaw, Poland.
Gonc¸alves, P. S. and Pinto, J. C. (2003b). An experi-
mental testbed for visual servo control of robotic ma-
nipulators. In Proceedings of the IEEE Conference
on Emerging Technologies and Factory Automation,
pages 377–382, Lisbon, Portugal.
Gustafson, D. E. and Kessel, W. C. (1979). Fuzzy clustering
with a fuzzy covariance matrix. In Proceedings IEEE
CDC, pages 761–766, San Diego, USA.
Hutchinson, S., Hager, G., and Corke, P. (1996). A tuto-
rial on visual servo control. IEEE Transactions on
Robotics and Automation, 12(5):651–670.
Reis, J., Ramalho, M., Pinto, J. C., and S
´
a da Costa, J.
(2000). Dynamical characterization of flexible robot
links using machine vision. In Proceedings of the 5th
Ibero American Symposium on Pattern Recognition,
pages 487–492, Lisbon, Portugal.
Sousa, J. M., Silva, C., and S
´
a da Costa, J. (2003). Fuzzy
active noise modeling and control. International Jour-
nal of Approximate Reasoning, 33:51–70.
Suh, I. and Kim, T. (1994). Fuzzy membership function
based neural networks with applications to the visual
servoing of robot manipulators. IEEE Transactions on
Fuzzy Systems, 2(3):203–220.
Tahri, O. and Chaumette, F. (2003). Application of moment
invariants to visual servoing. In Proceedings of the
IEEE Int. Conf. on Robotics and Automation, pages
4276–4281, Taipeh, Taiwan.
ICINCO 2004 - INTELLIGENT CONTROL SYSTEMS AND OPTIMIZATION
150