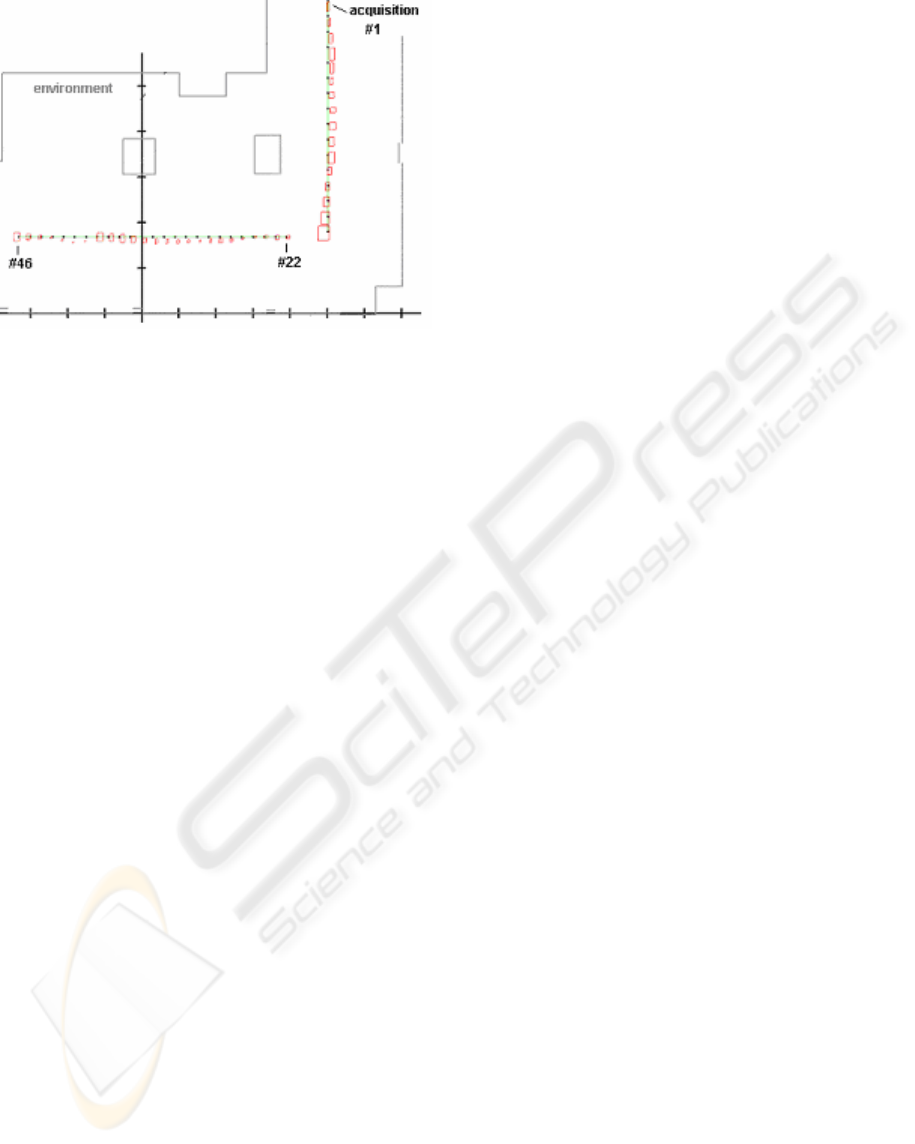
Figure 11: two dimensions localization results.
5 CONCLUSION
We have presented in this article a localization
method based on constraint propagation on intervals.
Indeed, the localization problem can be modelled as
a constraint satisfaction problem (CSP). In our case,
the imprecise information used to localize the robot
come from two sensors: two odometers and an
exteroceptive sensor. These two sensors give
measurements which are linked by some constraints.
These constraints induce a reduction of the
subpaving which represents the robot localization.
Another advantage of this method is its ability to
treat naturally and easily imprecise data: these data
are represented by intervals. So, the localization
imprecision quantification is intrinsically managed
by our algorithm.
The localization strategy is based on multi target
tracking. This strategy, which can be seen in our
case as a propagation of a matching during the
robot’s displacement, is less complex than classical
methods. Besides, our matching method, which is
based on the use of the TBM, gives us an uncertainty
value about each matching done. This value can
allow to estimate an uncertainty about each track,
and thus manage the problem of track cancel: if a
track is to uncertain, it will be cancelled. This track
uncertainty management is one of the main future
perspectives that will concern this work.
REFERENCES
Smets, Ph, 1998. The Transferable Belief Model for
Quantified Belief Representation, Handbook of
Defeasible Reasoning and Uncertainty Management
Systems, Kluwer Ed, pp 267-301.
Jaulin L, Braems I, Kieffer M, Walter E, 2001. Nonlinear
state estimation using forward-backward propagation
of intervals in an algorithm, In Scientific Computing,
Validated Numerics, Interval Methods, pp191-204,
Kluwer Academic Publishers.
Moore R.E, 1979. Methods and applications of interval
analysis, SIAM, Philadelphia.
Jaulin L, Kieffer M, Didrit O, Walter E, 2001. Applied
interval analysis, Springer-Verlag
Leonard J, Durrant-Whyte H, 1991. Mobile robot
localization by tracking geometric beacons, IEEE
Trans. on Robotics and Automation, Vol. 7, n°3, pp.
89-97.
Chung H, Ojeda L, Borenstein J, 2001. Sensor fusion for
Mobile Robot Dead-reckoning With a Precision-
calibrated Fiber Optic Gyroscope – proc. of the 2001
IEEE Int. Conf. on Robotics & Automation, pp.3588-
3593.
Bouron P, Meizel D, Bonnifait P, 2001. Set-membership
non-linear observers with application to vehicle
localisation, ECC'01 European Control Conference.
Clerentin A, Delahoche L, Brassart E, 2001.
Omnidirectional sensors cooperation for multi-target
tracking, IEEE Int . Conf. on Multisensor Fusion and
Integration for Intelligent Systems (MFI 2001).
Clerentin A, Delahoche L, Brassart E, Cauchois C, 2002.
An Uncertainty Propagation Architecture for the
Localization Problem, Workshop on Performance
Metrics for Intelligent Systems PerMIS2002, NIST,
Washington, USA, August 2002
Royere C, 2002. Contribution à la résolution du conflit
dans le cadre de la théorie de l’évidence, PhD thesis,
Université de Technologie de Compiègne, 2002
Clerentin A, Delahoche L, Brassart E, Drocourt C, 2003..
Uncertainty and error treatment in a dynamic
localization system, proc. of the 11th Int. Conf. on
Advanced Robotics (ICAR03), pp.1314-1319,
Coimbra, Portugal.
Preciado A, Meizel D, Segovia A, Rombaut M, 1991.
Fusion of multi-sensor data: a geometric approach”, in
proc. IEEE Int. Conf. On Robotics and Automation
(ICRA’91), vol 3, pp. 2806-2811, Sacramento, USA.
Hanebeck U.D, Schmidt G, 1996. Set theoretic
localization of fast mobile robots using an angle
measurement technique, in proc. IEEE Int. Conf. On
Robotics and Automation (ICRA’96), pp. 1387-1394.
Meizel D, Leveque 0, Jaulin L, Walter E, 2002. Initial
Localization by Set Inversion. IEEE trans. on Robotics
and Automation. Vol 18, n° 6, pp 966-971.
ICINCO 2004 - ROBOTICS AND AUTOMATION
242