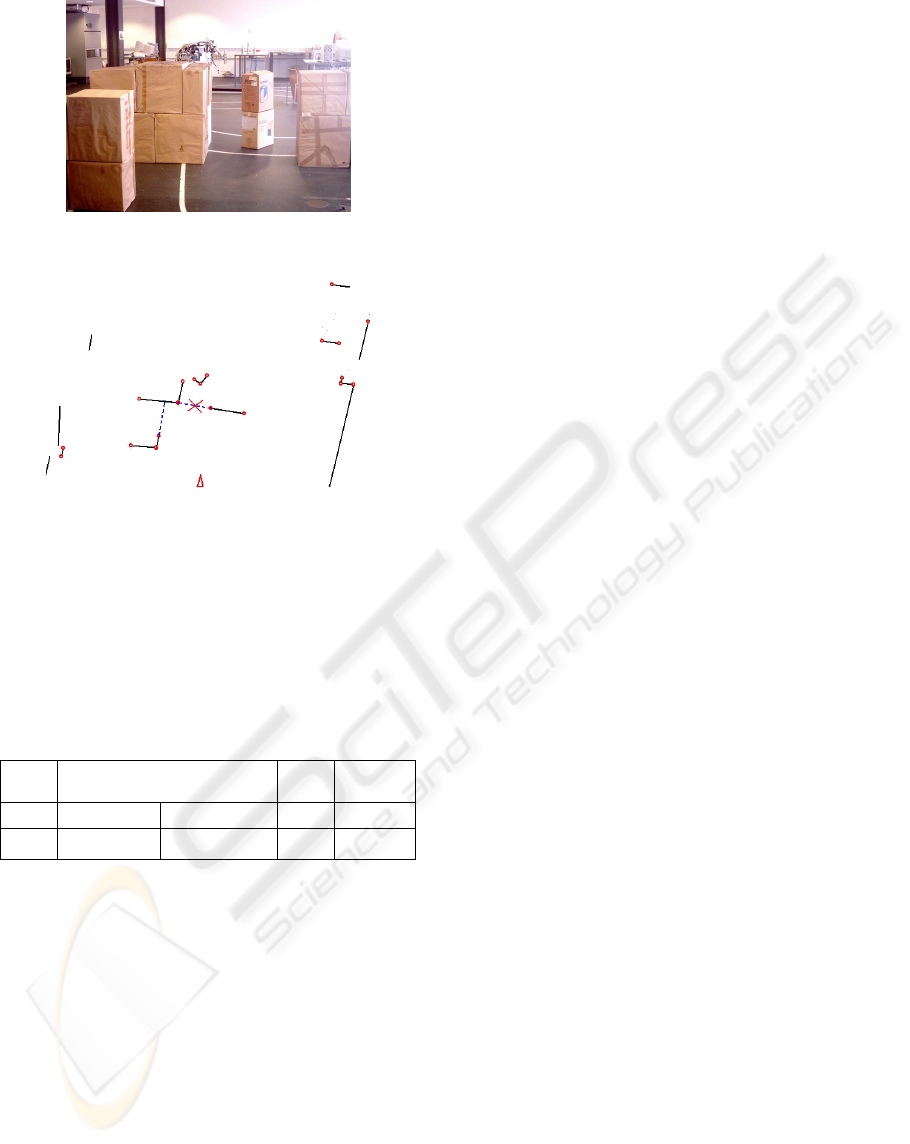
Figure 7: Experimental setup with two narrow passages
built with paper boxes with one of them blocked ahead.
Figure 8: Laser map where two potential narrow passages
were detected but one rejected due to obstruction. The
triangle on the bottom represents the position of the robot.
In Figure 8 the dark lines represent the walls, and
the small circles at the ends of the lines represent the
Complete Points. The two detected entrances of
passages were drawn with dashed lines. The results
of this procedure are summarized in Tab.1.
Table1: Results of narrow passages detection from Figure
8
Type
Coordinates of
Ending Points (mm)
Width
(mm)
Passable
(T/F)
I (344, 2598) (-734, 2761) 1090 False
II (-1378, 1671) (-1171, 2807) 1154 True
Approaching doors resulted as planned and
expected since odometry demands were simple.
Both the solutions depicted in Fig. 5 and Fig. 6
turned out well with the second one more efficient in
cluttered environments.
Crossing the doors proved quite efficient with
the configuration or robot as shown in Figure 6 but a
new configuration where the laser was moved
forwards for other types of data acquisition resulted
less efficient. That is to be enhanced in the near
future.
6 CONCLUSIONS
In this paper an algorithm to detect and cross narrow
passages was described. In feature maps the passage
entrances composed of two corners or one corner
and one wall are the highest-level features and could
be regarded as important natural landmarks that
provide localization information to the robots. The
implementation of the behavior was also simple and
practical. Approaching doors and traverse them also
proved the success of the proposed methods.
ACKNOWLEDGEMENTS
This work has been developed under a bilateral
cooperation between Portugal and the People’s
Republic of China sponsored by the former ICCTI,
Ministry of Science and Technology of the
Portuguese Government.
REFERENCES
Chung, H., Yong Seek Choi, Jang Gyu Lee, 1992. Path
Planning For A Mobile Robot With Grid Type World
Model
, lEEE/RSJ International Conference on
Intelligent Robots and Systems, vol.1, pp. 439–444.
Davison, A.J., Murray, D.W., 2002. Simultaneous
localization and map-building using active vision,
IEEE Transactions on Pattern Analysis and Machine
Intelligence, Volume: 24, No. 7, pp. 865 –880.
Kulich, M., Stepan, P., Preucil, L., 1999, Feature detection
and map building using ranging sensors,
IEEE/IEEJ/JSAI International Conference on
Intelligent Transportation Systems, pp 201-206.
Lionis, G.S., Kyriakopoulos, K.J., 2002, A laser scanner
based mobile robot SLAM algorithm with improved
convergence properties, IEEE/RSJ International
Conference on Intelligent Robots and System, pp. 582
–587, vol.1.
Santos, V., Oliveira, E., 2001. Missões de navegação para
um robot móvel baseadas em tarefas e relações
topológicas do ambiente, Robotica, No. 45, pp. 14–25.
de Wit, C., Siciliano, B., Bastin, G., 1997, Theory of
Robot Control (Eds.), Springer.
Xiang, Z., Santos V., Liu, J., 2003, Robust Mobile Robot
Localization by Fusing Laser and Sonar, Proceeding
of International Conference on Advanced Robotics,
ICAR2003, June, Coimbra, Portugal, pp.276-280.
ICINCO 2004 - ROBOTICS AND AUTOMATION
374