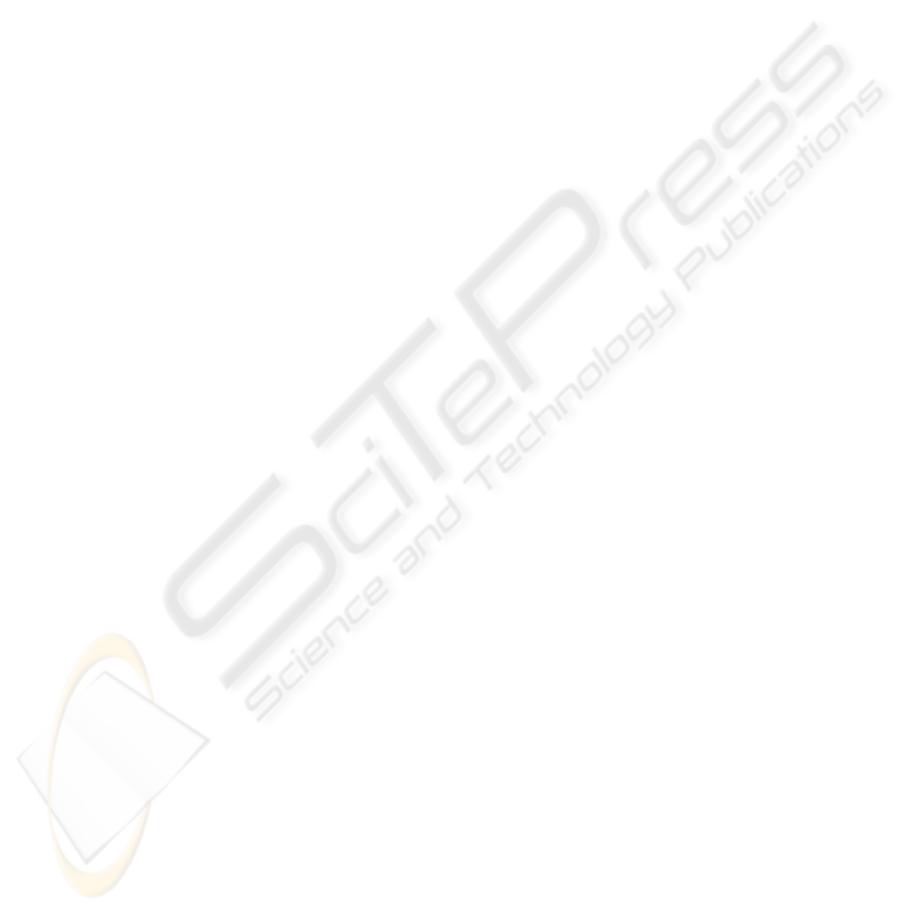
series induces linearization error. In this paper, a
position estimation method with linear process and
measurement equations is developed. Process and
measurement equations are linear by appropriately
constructing state vector and system models. With
linear process and measurement function, we apply
linear Kalman filter to estimate optimally the
position of robot. It avoids linear approximation of
nonlinear system equations and is free of
linearization error. The filter is consistent and
convergent. Comparing with EKF, it gives more
conservative estimation result.
REFERENCES
Alspach, D. and Sorenson, H. 1972. Nonlinear Bayesian
estimation using Gaussian sum approximations. IEEE
Transactions on Automatic Control, vol. AC-17, pp.
439-448.
Bellaire, R.L., Kamen E.W. and Zabin, S.M. 1995. A new
nonlinear iterated filter with applications to target
tracking. In SPIE Proceedings on Signal and Data
Processing of Small Targets, vol. 2561, pp. 240-251.
Borenstein, J. and Feng, L. 1995. Correction of Systematic
Odometer Errors in Mobile Robots. In Proceedings of
the 1995 International Conference on Intelligent
Robots and Systems (lROS'95), Pittsburgh,
Pennsylvania, pp. 569-574.
Chui, C.K., Chen, G. and Chui, H. 1990. Modified
extended Kalman filtering and parallel system
parameter identification, IEEE Transactions on
Automatic Control, 35 (1): 100-104.
Fox, D., Burgard, W., Thrun, S. and Cremers, A.B. 1998.
Position Estimation for Mobile Robots in Dynamic
Environments. AAAI/IAAI 1998: 983-988.
Fox, D., Burgard, W., Dellaert F. and Thrun, S. 1999.
Monte Carlo Localization: Efficient Position
Estimation for Mobile Robots. AAAI/IAAI 1999: 343-
349.
Glielmo, L., Setola R. and Vasca, F. 1999. An Interlaced
Extended Kalman Filter, IEEE Transactions on
Automatic Control, vol. 44, no. 8, pp. 1546-1549.
Ito, K. and Xiong, K. 2000. Gaussian Filters for Nonlinear
Filtering Problems. IEEE Transactions on Automatic
Control, 45(5): 910-927.
Julier, S., Uhlmann J. and Durrant-Whyte, H. 1995. A new
approach for filtering nonlinear systems. In
Proceedings of the American Control Conference,
pp.1628-1632.
Kalman, R.E. 1960. A New Approach to Linear Filtering
and Prediction Problems. Journal of Basic Engineering
Transactions of the ASME, pp.35-45.
Kalman, R.E. and Bucy, R. 1961. New results in linear
filtering and prediction theory. Journal of Basic
Engineering Transactions of the ASME, vol. 83, pp.
95-108.
Kelly, A.J. 1994. A 3D State Space Formulation of a
Navigation Kalman Filter for Autonomous Vehicles,
CMU Robotics Institute Technical Report CMU-RI-
TR-94-19.
Lefebvre, T., Bruyninckx, H. and De Schutter, J. 2002.
Comment on “A New Method for the Nonlinear
Transformation of Means and Covariances in Filters
and Estimators”. IEEE Transactions On Automatic
Control, Vol. 47, no. 8, pp.1406-1408.
Lefebvre, T., Bruyninckx, H. and De Schutter, J. 2003.
Non-linear Autonomous Compliant Motion with a
Non-minimal State Kalman Filter. In International
Conference on advanced Robotics, pp.136-141.
Mehra, R.K. 1971. A Comparison of Several Nonlinear
Filters for Reentry Vehicle Tracking. IEEE
Transactions on Automatic Control, AC-16(4): 307-
319.
Nam, K.H. and Tahk, M.J. 1999. A second-order
stochastic filter involving coordinate transformation.
IEEE Transactions on Automatic Control
, Vol. 44,
No.3, pp. 603-608.
Olson, C.F. 2000. Probabilistic self-localization for mobile
robots. IEEE Transactions on Robotics and
Automation, vol. 16(1), pp. 55-66.
Thrun, S. 2000. Probabilistic algorithms in robotics, AI
Magazine, 21(4): 93-109.
Wall, D.S. and Gaston, F.M.F. 1997. Modified extended
kalman filtering. In Proceedings of International
Conference on Digital Signal Processing, pp.703-706.
Wan, E.A. and van der Merwe, R. 2000. The unscented
Kalman filter for nonlinear estimation. In Proceedings
of Conf. Adaptive Systems for Signal Processing,
Communication and Control, Canada.
ICINCO 2004 - ROBOTICS AND AUTOMATION
248