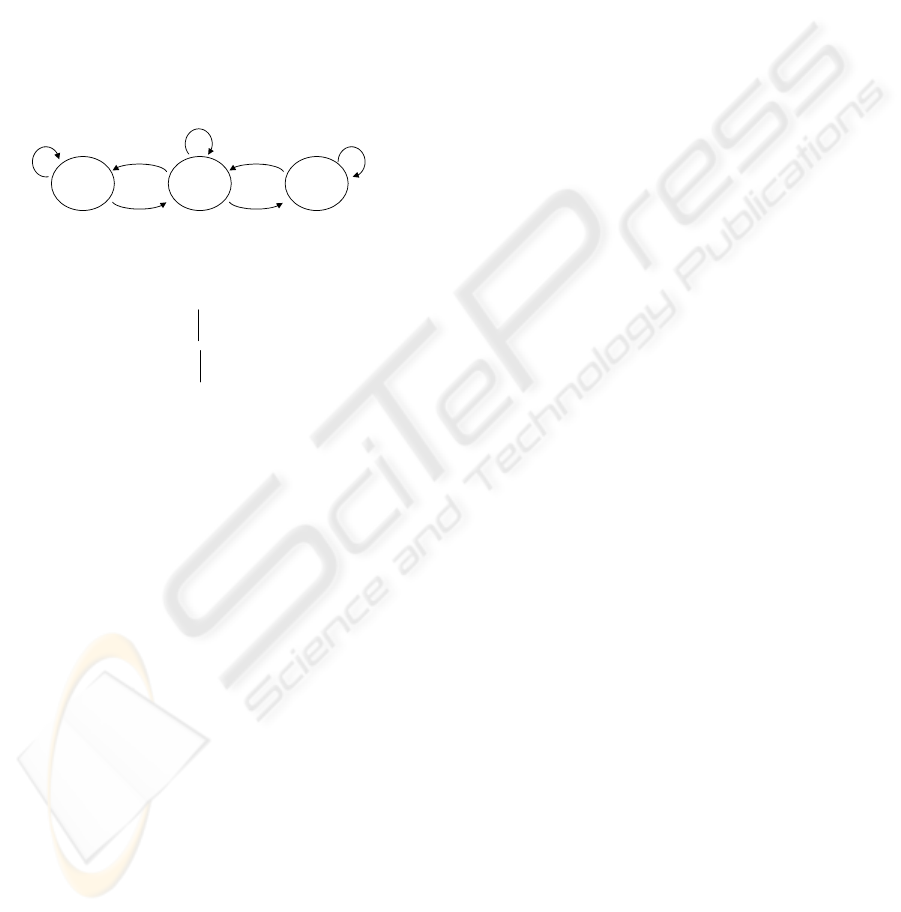
this case. But it must be noticed that the results in
table 1 and 2 for the SPMLD model are achieved
without applying techniques described in section
4.4. For example using a prediction horizon
3
N
and a control horizon
1
u
N leads to the following
results enabling real time implementation
No QPs solved = 1224, Max. No QPs/step = 326
Total optimization time = 29.24 s., Max. time/step = 7.92 s.
The technique of MPC for SPMLD systems has
been examined also with a simple automata, where
automata of Figure 7 have been added to
1
V and
2
V
valves of the three tanks benchmark. We assumed
for a simplification purpose that
03
0=
i.e. the
level in the third tank is always behind the h
v
level.
V
1
open
Wait
V
2
open
a b
close
close
close a
b
Figure 7: added Automata to the three tanks benchmark.
The automata of Figure 7 can be presented as follows
closeWait
bVbwaitV
aVawaitV
openopen
openopen
=
=
=
)&()&(
)&()&(
22
11
(23)
The SPMLD technique succeeds to reduce the total
optimization time to arrive to the specifications,
from 5691.4 s for the classical MLD technique to a
173.5 s, solving 3990 QPs instead of 60468 QPs
where for each sequence I,
variables as well as
the logical control variables that control the
automata are known.
δ
6 CONCLUSION
This paper presents the SPMLD formalism. It is
developed by partitioning the feasible region
according to the auxiliary binary elements
1
of the
MLD model that depends on the state variables. A
reformulation of the MPC strategy for this
formalism has been presented. It is shown that the
SPMLD model successfully improves the
computational problem of the mixed Logical
Dynamical (MLD) model and Piecewise Affine
(PWA) model. Moreover, the partition into several
sub-regions enables to define particular weighting
factors according to the priority of each region.
Future work may consider examining
variables
that depends on the control inputs, by partitioning
the feasible region according to those variables also
instead of leaving them free included in the
optimization vector.
δ
δ
REFERENCES
Bemporad, A., 2003. “A Recursive Algorithm for
Converting Mixed Logical Dynamical Systems into an
Equivalent Piecewise Affine Form”, IEEE Trans.
Autom. Contr.
Bemporad, A., Ferrari-Trecate G., and Morari M., 2000.
Observability and controllability of piecewise affine
and hybrid systems. IEEE Trans. Automatic Control,
45(10):1864–1876.
Bemporad, A., and Mignone, D., 2000.: Miqp.m: A
Matlab function for solving mixed integer quadratic
programs. Technical Report.
Bemporad, A., Mignone, D. and Morari, M., 1999.
Moving horizon estimation for hybrid systems and
fault detection. In Proceedings of the American
Control Conference, San Diego.
Bemporad, A. and Morari, M., march 1999. Control of
systems integrating logical, dynamics, and constraints.
Automatica, 35(3):407-427.
Branicky, M.S., Borkar, V.S. and Mitter, S.K., January
1998. A unified framework for hybrid control: model
and optimal control theory. IEEE Transaction. on
Automatic. Control, 43(1): 31-45.
Dolanc, G., Juricic D., Rakar A., Petrovcic J. and Vrancic,
D., 1998. Three-tank Benchmark Test. Technical
Report Copernicus Project Report CT94-02337.
Dumur, D. and Boucher, P.,1998. A Review Introduction
to Linear GPC and Applications. Journal A, 39(4),
pp. 21-35.
Fletcher, R. and Leyffer, S., 1995. Numerical experience
with lower bounds for MIQP branch and bound.
Technical report, Dept. of Mathematics, University of
Dundee, Scotland.
Kerrigan, E., 2000. Robust Constraint Satisfaction:
Invariant sets and predictive control. PhD thesis
University of Cambridge.
Pena, M.., Camacho, E. F. and Pinon, S., 2003. Hybrid
systems for solving model predictive control of
piecewise affine system. IFAC conference, Hybrid
system analysis and design Saint Malo, France.
Sontag, E.D., April 1981: Nonlinear regulation: the
piecewise linear approach. IEEE Transaction. on
Automatic. Control, 26(2):346-358.
Torrisi, F., Bemporad, A. and Mignone, D., 2000. Hysdel
– a tool for generating hybrid models. Technical
report, AUT00-03, Automatic control laboratory, ETH
Zuerich.
MODEL PREDICTIVE CONTROL FOR HYBRID SYSTEMS UNDER A STATE PARTITION BASED MLD
APPROACH (SPMLD)
85